A Huge New Multimodal AI Framework for Dementia Diagnosis
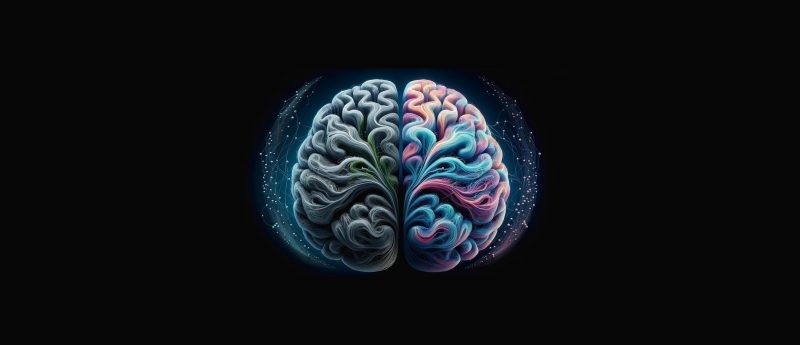
Trained on massive amounts of multimodal data across diverse geographical regions, this new AI framework for dementia diagnosis represents the next step towards a truly useful tool for clinics.
In the ever-evolving field of neurology, the differential diagnosis of dementia presents a complex challenge due to the overlapping symptoms among various etiologies. Accurate diagnosis is crucial for developing personalized management strategies early in the disease process.
A new study published in Nature Medicine introduces an AI model that integrates a wide array of data sources to differentiate between dementia types with remarkable accuracy.
The study, led by Chonghua Xue and a collaborative team of researchers, harnessed data from 51,269 participants across nine independent and geographically diverse datasets.
The AI model utilizes demographics, personal and family medical histories, medication usage, neuropsychological assessments, functional evaluations, and multimodal neuroimaging to identify ten distinct dementia etiologies. The integration of these varied data sources allows the model to mimic clinical reasoning and generate robust diagnostic predictions even with incomplete data.
The AI model demonstrated a microaveraged area under the receiver operating characteristic curve (AUROC) of 0.94 in classifying individuals with normal cognition, mild cognitive impairment (MCI), and dementia.
When differentiating dementia etiologies, the model achieved a microaveraged AUROC of 0.96. Notably, the model exhibited proficiency in addressing mixed dementia cases, achieving a mean AUROC of 0.78 for two co-occurring pathologies.
In a subset of 100 randomly selected cases, the AI-augmented neurologist assessments outperformed neurologist-only evaluations, with AUROC improvements averaging 26.25%.
The model’s predictions aligned with biomarker evidence, and its associations with different proteinopathies were substantiated through postmortem findings, highlighting its reliability and clinical relevance.
This AI framework has significant implications for enhancing dementia screening and diagnosis in clinical settings and drug trials. The ability to integrate and analyze multimodal data ensures that diagnoses are aligned with similar management strategies, providing a comprehensive tool for clinicians. The model’s robustness across diverse datasets and its proficiency in handling mixed dementias mark a step closer to applicability in clinical practise.