A Novel Method for Non-Destructive Cancer Diagnosis During Surgery
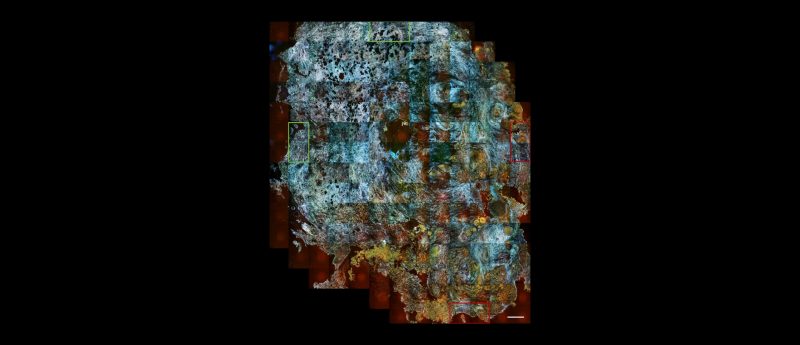
A recent study has introduced a near-real-time automated cancer diagnosis workflow combining dynamic full-field optical coherence tomography (D-FFOCT) and deep learning to address the limitations of traditional intraoperative assessments. This new method aims to provide a rapid, nondestructive, and accurate diagnosis of breast cancer during surgery.
Cancer remains one of the leading causes of mortality worldwide, with approximately 19.3 million new cases diagnosed annually. Surgery is often the most effective treatment, offering the potential for a cure. An intraoperative diagnosis is essential for making immediate decisions in the operating room, such as during breast-conserving surgery (BCS), where accurate margin assessment can reduce the need for reoperation. Currently, frozen section analysis based on hematoxylin and eosin histology is the most widely used intraoperative diagnostic method. However, this process is time-consuming, labor-intensive, and involves specimen destruction.
D-FFOCT is an innovative optical technology that captures intracellular metabolic features without requiring tissue preparation or staining. This method provides high-resolution visualization of live cells, creating a “virtual histology” that can distinguish between normal, benign, and cancerous tissues.
The deep learning model used in this study was based on the Swin Transformer architecture, a state-of-the-art computer vision model. The model was trained and fine-tuned on 10,357 D-FFOCT patches from 182 slides captured between April and June 2018. Subsequently, the model was tested on an independent set of 42 slides obtained between June and August 2018.
The deep learning model demonstrated outstanding performance in classifying benign and malignant breast tissues. The model achieved an accuracy of 97.62%, sensitivity of 96.88%, and specificity of 100%. Only one case of invasive ductal carcinoma was misclassified.
In a simulated intraoperative margin evaluation procedure, the new workflow significantly reduced the time required for diagnosis from approximately 30 minutes (with traditional methods) to just 3 minutes. The acquisition of D-FFOCT images was nondestructive, preserving the entire tissue sample for postoperative examinations.
The integration of D-FFOCT imaging with deep learning algorithms offers a promising alternative to traditional intraoperative histological analysis. This novel approach provides rapid, accurate, and nondestructive cancer diagnosis, potentially streamlining surgical procedures and reducing reliance on pathology laboratory resources.