Advancing Vaccine Production with More Accurate Antigen Binding Prediction
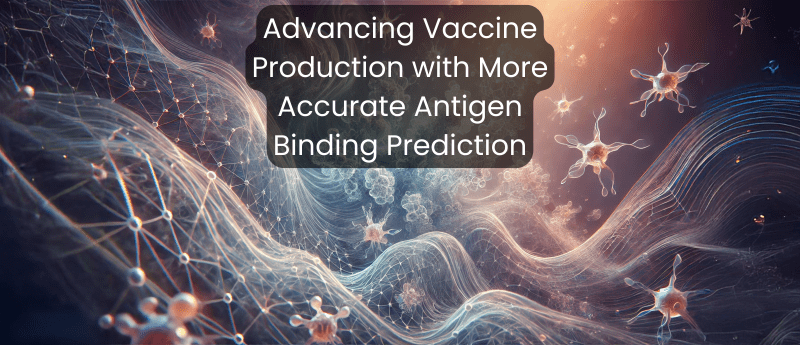
Researchers have made progress towards using AI to engineer vaccines with a new predictive model. It can reliably predict how antigens interact with proteins on the surface of immune cells. This new method performs substantially better than previous efforts. This new tool could make it easier and quicker to generate life-saving vaccines.
Vaccine Production is Fast, But Not Fast Enough
The core of vaccine development hinges on 3 basic processes: (1) introducing non-disease-causing antigens into the body, (2) recognition of these foreign toxins by human immune cells, and (3) acquiring an “immune memory” so that if these cells encounter these antigens in the real world, they are armed with the correct artillery to defend the body from disease.
The field of vaccinology nonetheless faces significant challenges – this is especially true for variable and complex pathogens.
For example, some microbes can mutate very quickly, changing the shape of the antigens on their cell surfaces so that immune cells may no longer recognize them. In some instances, pathogens can evade detection by the immune system entirely.
Indeed, the age of COVID-19 highlighted some flaws in the framework for vaccine production when under the strain of a global pandemic.
AI Is Promising, But Faces Challenges
As such, there is an unmet need for more efficient strategies. One growing area of interest, in this respect, is finding a way of predicting which epitopes (the part of an antigen that interacts with immune cells) bind to which T-cell receptors (TCRs).
This is where AI could change the game.
Scientists have already implemented machine learning (ML)/ deep learning (DL) models to identify the sequences of these epitope:TCR pairs; however, they are not without their caveats:
1. Pre-existing AI models perform well when they are tested with sequences that they are trained on, known as a ‘random split.’ However, it is difficult to develop a model that can make accurate predictions from epitope and TCR sequences that it has not seen before, i.e., where the dataset undergoes a ‘strict split.’
This is limiting in the context of vaccine development, since scientists may have to deal with pathogens that have not yet been encountered, i.e., during global pandemics.
2. Negative/non-binding datasets are a core component of training pre-existing ML/DL tools. These datasets encompass epitope/TCR sequences that are not compatible binding partners. However, these sequences are often randomly generated; as a result, pairs that are labeled as non-binding might actually bind.
After training, the machine could predict these ‘non-binding’ pairs as binding. While this would technically be correct, it is due to erroneous datasets that contain these mislabelled sequences. As such, this leads to overestimations in an AI model’s accuracy.
3. Most ML/DL AI models assign scores to sequences based on singular amino acid substitutions or their individual physicochemical properties. This is a restrictive strategy, disregarding how amino acids can influence each other and alter the binding patterns of epitopes/TCRs.
What’s The Solution?
A recent study published in Frontiers in Immunology shares insights into tackling these issues.
The research group produced a set of supervised ML models that performed substantially better than prior methods, with one methodology showing particular promise.
The article draws attention to 2 main features of the model:
-
- Experimentally validated negative binders. The group refrained from randomly generating negative binders, only including incompatible pairs that had been tested in real life.
- ‘Bigger picture’ approach. The researchers enriched the model’s training by including physicochemical properties based on the whole peptide sequence of an epitope/TCR. Essentially, they chose to look at how amino acids interact in a given context (i.e., how they are influenced by surrounding amino acids) rather than zooming in on individual amino acids.
Implications For The Future
Crucially, the significantly higher accuracy of the AI model (compared to previous reports) indicates that these methods could be applied to future studies to guide developments in the area. If researchers can use similar AI tools to predict how an antigen binds to an immune cell with unseen data, the entire field of immunology would markedly benefit.
Indeed, this may be vital for developing life-saving vaccines when under extreme time pressures.