AI could assist oropharynx cancer treatment
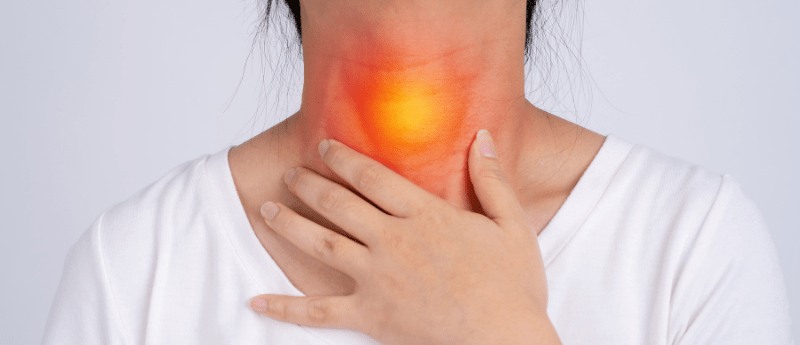
Researchers have developed a deep learning algorithm that performs better than expert radiologists when identifying extranodal extension (ENE) in oropharynx cancer patients.
Oropharynx cancer affects the oropharynx; the middle section of your throat including your tonsils and base of the tongue. The majority of oropharynx cancers are caused by human papilloma virus (HPV), the most prevalent sexually transmitted infection in the United States.
Although a rare type of cancer that is relatively easy to treat with chemotherapy and radiation or surgery, the presence of ENE, when tumor cells extend beyond lymph nodes and perforate the peri-nodal tissue, is a risk factor for HPV-associated oropharynx cancer recurrence and generally reduces survival rates.
Unfortunately, ENE is difficult to recognize in imaging tests prior to treatment, often resulting in unnecessary treatment-related toxicity effects and worse complications and quality of life. Early identification of HPV-associated oropharynx cancer patients with ENE is therefore of vital importance in determining the most appropriate treatment.
Investigators from Brigham and Women’s Hospital (MA, USA), and Dana-Farber Brigham Cancer Center (MA, USA) may have now designed a solution to this problem. After developing a deep learning algorithm and training it to identify ENE, they assessed the algorithm’s performance by putting it up against four head and neck specialist radiologists.
Having already demonstrated that the algorithm detected ENE with a high degree of accuracy in several prior datasets, the team of investigators performed a retrospective evaluation of the algorithm’s performance compared to the radiologists, utilizing data from surgical pathology reports and pretreatment CTs from a large multicenter phase 2 oropharynx cancer clinical trial (ECOG-ACRIN Cancer Research Group E3311).
Published in The Lancet Digital Health, the study results show that in fact, the AI algorithm performed better than the four expert radiologists at detecting ENE, with a greater degree of sensitivity and fewer missed ENEs.
“What was important about this study is it tested the algorithm in the context of a very large randomized clinical trial, where patients who were enrolled, by definition, were supposed to be screened out for having ENE, and yet a significant portion still ended up having ENE,” explained Kann, a doctor in the Department of Radiation Oncology at Brigham and Women’s Hospital. “When we applied the algorithm to this population to see how it would have done in predicting ENE, we found that it performed well with a high degree of accuracy — better than all four expert head and neck radiologists.”
These results indicate that incorporation of the algorithm into clinical practice may provide physicians with additional insight and greater accuracy of information in regards to patients with ENE. This may improve patient quality of life and treatment outcome as we can decide on the most appropriate treatment option based on AI guidance. The AI algorithm is currently being tested further in a clinical trial, to assess if it can help improve treatment outcomes.