AI Could Help Detect Pulmonary Embolism Early, Study Finds
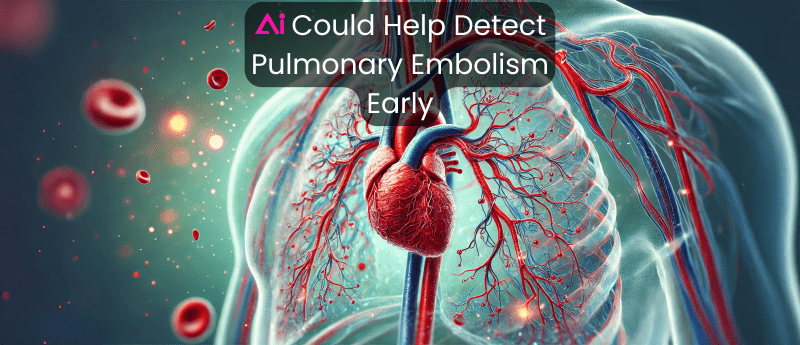
Two AI-based tools developed by researchers from Sheba Medical Center (Israel) demonstrated high accuracy in identifying patients suffering from pulmonary embolism (PE).
The study, published recently in the Journal of Medical Internet Research, utilized machine learning (ML) models to detect patients with a high likelihood of developing PE. By relying on previous patient medical history data, the ML models accurately identified patients at high risk for PE before any clinical checkups took place during hospital admission.
Methodology and Results
The two ML models were developed and trained to use demographics, comorbidities and medication record information from 2,568 patients with PE and 52,598 control patients.
To evaluate 13 input variables, the study employed a Random Forest classifier, which is formed by different tree-structured classifiers trained on bootstrapped samples. Three hyperparameters were tweaked to improve the RF’s performance:
- Maximum depth— defined by the researchers as “the longest path possible from a tree root to a tree leaf”;
- Minimum sample split— “the minimal number of samples required to split an internal node”;
- Split criterion— “either the Gini index impurity or the information gain”
The model’s outcome was determined by a majority vote among the trees.
The objective of the study was to determine if the ML models could predict PE during hospitalisation by analysing history data including age, sex, Body Mass Index, past clinical PE events, chronic lung disease, anticoagulant use and past thrombotic events.
The two models demonstrated high accuracy in identifying patients with PE obtaining an 80% overall accuracy in classifying PE and non-PE patients.
Whilst seven features were included in the methodology, researchers pinpointed three as critical for PE detection: a history of PE, chronic lung disease and previous thrombotic events.
What This Could Mean for the Future of PE Detection
The ML models used in the study demonstrated a significant capacity to quickly identify patients at high risk of PE even before clinical examination. Additionally, the study identified two other factors that may indicate an increased risk of PE diagnosis: a history of deep vein thrombosis and previous pneumonia.
“In addition to past PE, which is arguably the most important variable for marking patients with suspected PE, past diagnoses of pneumonia and DVT may also lead to PE,” Segal, Boaz Lerner, PhD, associate professor in the department of industrial engineering and management at Ben Gurion University and colleagues said. “Thus, we recommend overattention to admitted patients with these past diagnoses.”
“We are seeing a shift in medicine which hints to the future of care,” Segal added. “Instead of a personal experience, we are moving towards big data accumulation. Instead of clinical intuition, we are embracing AI. I expect future studies to continue focusing on how we can integrate big data and AI into clinical prediction models for different diseases.”
Is AI Ready to Meet the Expectations?
In recent years, AI has proven to have the potential to contribute to advancements in several different fields of healthcare, including cancer investigation, dementia diagnosis and vaccine production.
However, AI still faces challenges surrounding AI device clinical validation, data privacy and its ethical application in modern clinical procedures. This context limits the wider adoption of AI as a reliable tool in modern healthcare.
As a result, future research must focus on finding a solution to these issues to ensure current healthcare continues to prioritise safeguarding patient’s needs.