AI could predict five types of heart failure
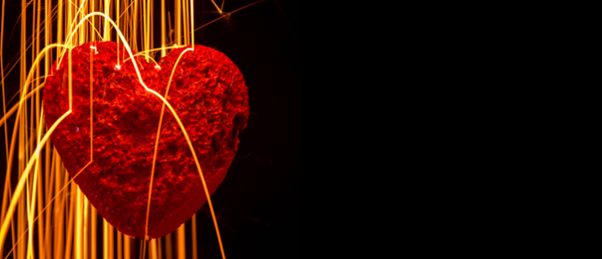
Researchers at UCL used AI tools to categorize five subtypes of heart failure and predict patients’ future mortality risks by analyzing patient data.
Heart failure is a broad term that covers a range of conditions wherein the heart fails to effectively circulate blood throughout the body. Existing methods of categorizing heart failure often fall short in accurately predicting the progression of the disease.
A recent study led by researchers at University College London (UCL) (UK) investigated the use of AI tools to predict the future risk of five subtypes of heart failure in individual patients. By analyzing extensive anonymized patient data from over 300,000 individuals using machine learning, aged 30 years or older and diagnosed with heart failure in the UK over a 20-year period, researchers identified five distinct subtypes. These subtypes include; early onset, late onset, atrial fibrillation related (associated with irregular heart rhythm), metabolic (linked to obesity with a low incidence of cardiovascular disease), and cardiometabolic (linked to obesity and cardiovascular disease).
The research team observed notable differences among the subtypes regarding patients’ mortality risks within the first year following diagnosis. The one-year all-cause mortality risks were; early onset (20%), late-onset (46%), atrial fibrillation related (61%), metabolic (11%), and cardiometabolic (37%).
Further to this analysis, the team then developed an application that clinicians could utilize to potentially determine the specific subtype of heart failure in an individual and their future mortality risks.
Professor Amitava Banerjee, the lead author from the UCL Institute of Health and Informatics stated, “We sought to improve how we classify heart failure, with the aim of better understanding the likely course of disease and communicating this to patients. Currently, how the disease progresses is hard to predict for individual patients. Some people will be stable for many years, while others get worse quickly. Better distinctions between types of heart failure may also lead to more targeted treatments and may help us to think in a different way about potential therapies. In this new study, we identified five robust subtypes using multiple machine learning methods and multiple datasets.”
The study employed four distinct methods to categorize cases of heart failure to ensure impartiality and minimize bias potentially caused by using a singular machine learning approach. These methods were applied to data from two large UK primary care datasets, Clinical Practice Research Datalink (CPRD) and The Health Improvement Network (THIN), which covered a 20-year span from 1998 to 2018. These datasets provided a representative sample of the entire UK population, with linked records of hospital admissions and death records, enabling a comprehensive and reliable analysis.
The team also examined genetic data obtained from the UK Biobank study, focusing on 9,573 individuals diagnosed with heart failure. From this, they found a correlation between particular subtypes of heart failure and elevated polygenic risk scores for conditions such as atrial fibrillation and hypertension.
Professor Banerjee concluded, “The next step is to see if this way of classifying heart failure can make a practical difference to patients – whether it improves predictions of risk and the quality of information clinicians provide, and whether it changes patients’ treatment. We also need to know if it would be cost effective. The app we have designed needs to be evaluated in a clinical trial or further research, but could help in routine care.”