AI-Driven Artifact Detection Improves Diagnostic Accuracy in Digital Pathology Systems
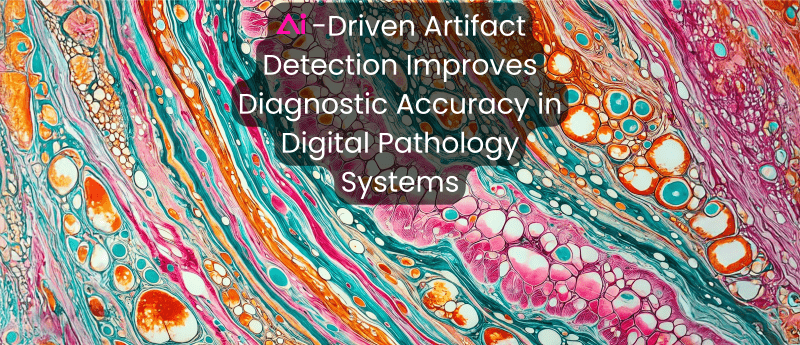
A research group at the University of Stavanger (Norway) has developed an innovative deep learning (DL) algorithm that could significantly improve the speed and accuracy of image analysis. By identifying and excluding errors—known as artifacts—present in microscope slide images, the new model overcomes a key challenge facing current models.
Putting Digital Pathology Under The Microscope
Cancer remains one of the world’s most pressing health issues, with cases expected to rise by 77% between 2022 and 2050. As the demand for timely and personalized diagnoses grows, the healthcare system faces a major challenge: a shortage of pathologists trained to analyze tissue samples under the microscope.
This shortage, combined with the complexity of modern diagnostics as cancer subtypes continue to emerge, means longer wait times for patients, which can delay critical treatment.
In this context, digital pathology is emerging as a key solution. By using AI to analyze digitized microscope images of tissue samples, these systems promise to accelerate diagnosis, reduce human error, and streamline workflows.
However, these computerized systems face a significant hurdle: artifacts—irrelevant details in the slides caused by factors like preparation errors or digital compression.
The Challenge of Artifacts in Digital Pathology
Artifacts, such as blurred sections, air bubbles, or debris, are common in digital images of tissue slides. While human pathologists can easily disregard these visual distortions, AI models often misinterpret them as diagnostically relevant, potentially leading to incorrect analyses.
This issue limits the effectiveness of AI-driven pathology systems in clinical settings, where accuracy is critical.
A recent study published in BMC addresses this problem head-on. The research team designed an artifact detection system using deep learning to identify and exclude these artifacts, ensuring that only the relevant biological data is analyzed.
Developing a Deep Learning Pipeline
The research group developed a series of deep learning (DL) pipelines—structured workflows designed to train, build, and evaluate AI models for detecting artifacts in whole slide images (WSIs).
These pipelines focus on two prominent deep learning frameworks: convolutional neural networks
(CNNs) and vision transformers (ViTs).
Each has advantages: CNNs are better at handling small datasets, while ViTs excel at capturing complex visual patterns. However, both frameworks suffer from issues like poor generalizability and overfitting when applied to new data.
To overcome these challenges, the team proposed a “mixture of experts” (MoE) approach, combining the strengths of multiple DL models. This method involves training several individual models, each specializing in detecting a specific type of artifact (e.g., tissue damage or blurred areas).
The predictions of these “expert” models are then fused to create a more refined and accurate output.
This MoE framework allows the AI system to dynamically combine the expertise of multiple models, which improves reproducibility and generalization. However, this improvement comes with a trade-off: the MoE method is more computationally intensive than traditional single-model approaches.
Meta-Learned Probabilistic Thresholding: A Key Breakthrough
One of the most critical innovations in this research is the application of meta-learned probabilistic thresholding. This technique allows the AI to “learn” optimal thresholds for distinguishing artifacts from relevant tissue, adjusting these thresholds over time as the model is trained.
Essentially, the system becomes better at identifying irrelevant regions (artifacts) and excluding them from analysis as it gains experience.
This thresholding method dramatically improved the AI’s ability to detect artifact-free areas, a crucial development for ensuring that diagnostic algorithms focus only on biologically meaningful tissue. By using this adaptive approach, the system becomes more accurate and reliable, even when faced with diverse or unseen data.
A Practical Impact on Cancer Diagnosis
After extensive testing, the researchers found that the MoE approach outperformed traditional multiclass models in terms of accuracy, particularly in challenging real-world datasets from different hospitals and cancer types.
The DL models were able to detect artifacts with a sensitivity of 97.93%, ensuring that only relevant tissue is analyzed, which greatly improves the reliability of the AI-based diagnostic systems.
This work has the potential to dramatically enhance the efficiency of clinical workflows by reducing the time pathologists spend manually correcting AI errors. With the ability to accurately exclude irrelevant data, these AI models could speed up cancer diagnoses and improve the overall quality of care.
The Road Ahead
While the MoE approach presents a breakthrough in artifact detection, the researchers acknowledge some challenges remain. The model was trained on data from a single cohort, limiting its generalizability across all types of cancer and tissue samples.
Future research will focus on expanding the dataset to improve the model’s robustness and adapting the AI pipelines to handle an even wider variety of clinical scenarios.
Nevertheless, the team’s innovative use of DL pipelines, particularly the MoE framework combined with meta-learned thresholding, represents a significant step forward in AI-driven digital pathology.
By making the diagnostic process more accurate and efficient, this technology has the potential to deliver faster results for patients—a critical factor when time could mean the difference between life and death.