AI Identifies Alzheimer’s Drug Able to Induce Short-Term Hibernation
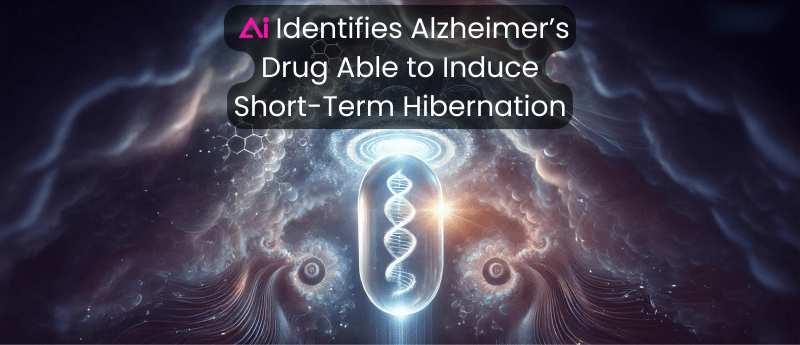
A research group has found that a drug used to treat Alzheimer’s can induce a state resembling a short-term hibernation, known as torpor. The researchers used an AI algorithm to screen for drugs which were likely to produce such an effect, leading to the identification of a prime candidate. Repurposing this drug could help save lives in emergency situations.
What Exactly is Torpor?
Torpor describes an energy-saving process in which animals drop their metabolic rate and body temperature. This usually only lasts for a few hours during the day, and could be considered a lighter form of hibernation.
In the animal kingdom, torpor is used in challenging circumstances that would normally prove lethal to the animal, for example, after severe injury or during harsh winters.
Unlocking Life-Saving Potential
So, how might this relate to humans? Well, scientists are interested in developing a new class of drugs called “biostasis therapeutics” – drugs that can simulate this state of energy conservation.
In some clinical contexts, such as heart surgery, hypothermia is used to decrease metabolic processes and preserve organ function. However, this is difficult to implement in emergency situations where resources are limited.
That’s where the recent workings of Maria Plaza Oliver and colleagues bring this area into focus.
How AI Helped Researchers Find the ‘Right’ Drug
Nonetheless, the group (affiliated with the Wyss Institute for Biologically Inspired Engineering at Harvard University) continued the search for a safe and effective “biostasis therapeutic”. They used an AI tool called NeMoCAD to predict which drugs would produce a torpor-like state.
Broadly speaking, the methods used to build their algorithm can be divided into 3 steps:
-
- Identifying subsets of genes whose manipulation, such as increasing or decreasing their expression, is able to recreate the torpor-like state.
- Comparing two datasets: a library of genes that pre-existing compounds target, and the target ‘signature’, identified in step 1.
- Predictions of which compounds would be optimal for targeting this signature. This integrated two core elements: gene–gene and gene–drug interactions – the former outlining the ways in which different genes influence each other, and the latter referring to how drugs interact with genes.
These different components formed the basis of their machine learning tool, which was then used to generate 378 compounds as possible matches, of which 197 were FDA-approved drugs.
Final selection of the optimal candidate was made by comparing chemical structures of the possible compounds and SNC80 – the molecule that the group had previously discovered.
They found that a drug indicated for treating Alzheimer’s (donepezil, DNP) could have the potential to successfully induce this energy-saving condition.
Indeed, the group applied DNP to an animal model and observed a decrease in activity associated with a torpor-like state, which was easily reversible with a “biostasis inducer”.
What’s more, they suspended the drug in a ‘nanoemulsion’ (tiny droplets made from oil, water and amphiphilic molecules) which changed the absorption of DNP and significantly decreased its toxicity, showing promise for human applications.
Future Implications for Emergency Medicine
The results of this study benchmark an exciting time for the future of emergency medicine. The concept of “biostasis therapeutics” is relatively unexplored, but represents an important avenue for consideration.
These drugs could be administered to people with severe injuries in places with limited resourcing, biding clinicians time to save their lives.
This research also demonstrates the shining possibilities that AI offers in this area. Using machine learning algorithms allows scientists to quickly and accurately predict compounds which can produce a desired outcome – in this case, a state that closely resembles torpor.
Of course, the results of this study should still be regarded with caution. Although the group found that the animal model they used can be used to predict human responses, it is difficult to know if this truly represents what would happen if given in clinical scenarios.