AI is Helping to Solve the Mystery of Ovarian Cancer
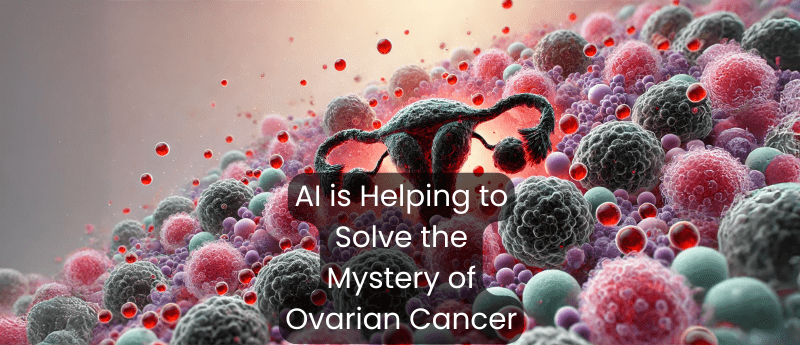
Researchers at the National Natural Science Foundation of China have developed a new AI model, called DFASGCNS, capable of predicting prognostic outcomes of patients with ovarian cancer.
Ovarian Cancer is Often Diagnosed Too Late
Detecting ovarian cancer in the early stages is notoriously difficult due to its vague and non-specific symptoms.
Early warning signs tend to mimic other diseases, such as irritable bowel syndrome, or manifestations of mental health problems, like stress or depression. As a result, cases of ovarian cancer are frequently diagnosed only at advanced stages, specifically, at stage III or IV. Treatment options are limited at these stages, and patient outcomes are generally poor.
The pathophysiology of ovarian cancer is a complex process and involves many molecular factors. Broadly, these can be grouped into three major categories or ‘omics’: epigenomics, genomics, and transcriptomics.
Scientists are working to unravel the molecular drivers of ovarian cancer, by expanding our knowledge in these three omics categories. This is a crucial step toward identifying new diagnostic biomarkers and potential druggable targets.
Beyond simply understanding the pathophysiology of ovarian cancer, researchers are also focusing their efforts to develop a tool to predict patients’ prognoses. Accurate prognostic tools could enable doctors to personalize treatment plans, allowing for early identification of patients who might benefit from more aggressive therapies.
Understanding Such a Complex Disease Requires State-of-the-Art Technology
AI has the potential to dramatically advance our understanding of the pathophysiology of ovarian cancer and improve predictions of patient outcomes.
AI systems could detect subtle patterns in multi-omics data shedding light on factors associated with poor prognoses and identifying the molecular contributors driving the disease’s progression.
However, achieving this is no small task. Current research primarily employs two main approaches: feature-fusion methods and graph-based fusion methods:
- Feature-fusion methods: integrate and merge clinical data of different omics to capture traits linked with certain prognostic outcomes, such as differential gene expression. However, this clinical data is usually huge and is hard to simplify. Thus, these techniques represent them in a low-dimensional space – essentially, compressing the data by condensing the number of features in the original dataset into a smaller number. While this approach is efficient, this method might overlook subtle but important details, leaving gaps in our knowledge of molecular mechanisms driving ovarian cancer.
- Graph-based fusion methods: focus on the relationships between different types of omics data, rather than individual features. This approach allows researchers to categorize different tumor subtypes by identifying distinct epigenetic, genetic, or transcriptomic signatures across patient groups. Although this method excels at capturing interactions between multimodal data, they are less suited for studying individual features —such as specific mutations or methylation patterns—that might contribute to the development or progression of ovarian cancer.
Combining the Best of Both Worlds
A new study published in PLoS One introduces a new AI model, DFASGCNS, to address the limitations of feature-fusion and graph-based fusion methods.
This innovative AI model combines feature-fusion and graph-based fusion methods into a dual fusion channel strategy, enabling the identification of key individual features in cancer samples while simultaneously analyzing connections across different omics data.
This AI model utilizes stacked layers of graph convolutional networks (GCNs) in the framework, which are neural networks designed to analyze and learn from graph-structured data by capturing relationships between nodes and their connections (edges).
Stacked-layer GCNs offer many benefits over single-layer GCNs.
In a single-layer GCN, patient samples are represented as ‘nodes’ linked by ‘edges’ that reflect their relationships. Using this graph-structured data, the single-layer GCN learns the features of patient samples and detects patterns.
However, single-layer GCNs are limited to capturing interactions between adjacent nodes, neglecting more complex associations between distant samples from different omics streams.
Stacked GCNs address this limitation, as they can capture subtle patterns between diverse data types and detect patterns between distant nodes tied to different omics streams.
For example:
Two cancer samples are analyzed and belong to the same tumor subtype. One sample displays high RNA expression, while the other has low methylation levels. In a singular GCN, this relationship would not be connected and analyzed together, however, stacked GCNs connect these two samples by looking at how other nodes with similar features overlap.
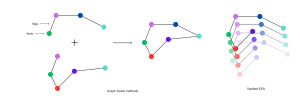
(Left) Graph-fusion methods: Each graph represents different types of omics data. Nodes represent patient samples and edges represent the relationships between these samples. Graphs are fused to represent multi-omics data. (Right) Stacked GCN: Multiple GCN layers stack to model complex, long-distance relationships, revealing deeper patterns within the fused graph.
Stacked GCN provides a more comprehensive understanding of the key genetic, transcriptomic, and epigenomic factors driving the development and progression of ovarian cancer.
It also enables more accurate prediction of patient outcomes, helping to personalize treatment plans for each patient. In this study, the research team reports that their AI model achieved an accuracy rating (ACC) of over 70%.
Is AI the Future of Ovarian Cancer Research?
The study published in PLoS One highlights how AI can help scientists investigate the mechanisms underlying ovarian cancer and identify potential mutations or molecular signatures that might be involved.
However, AI models usually suffer from a trade-off between in-depth analyses of individual features from single-omics data, or low-resolution analyses of multi-omics information.
By integrating a dual fusion channel strategy with a stacked GCN framework, DFASGCNS strikes a balance between the two and bridges this gap.
This architecture allows researchers to examine both subtle, localized changes and global shifts affecting various biological pathways.
This approach not only holds promise for improving clinical predictions of patient outcomes but also offers a deeper understanding of ovarian cancer among the scientific community.