AI maps tumor geography for tailored treatments
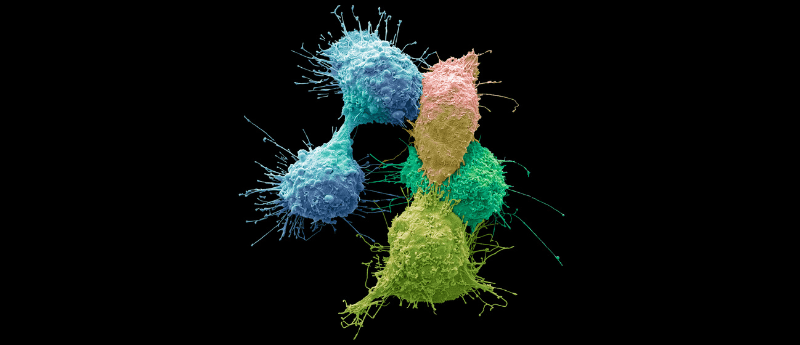
Researchers have integrated AI approaches from satellite mapping and community ecology to develop a tool to interpret data obtained from tumor tissue imaging, with the aim of implementing a more individualized approach to cancer care.
In recent years, huge advances have been made in tumor tissue imaging techniques. These developments have led to an incredibly rich amount of raw data. For instance, dozens of biological markers associated with the function and health of individual cells within tumor tissue can be quantified. This data contains information regarding the specific characteristics of different tumors, such as how they would respond to certain treatments. This could then be used to inform more effective and personalized treatment plans for individual cancer patients. However, strategies to effectively analyze and interpret this wealth of information have lagged behind these strides in imaging.
A collaboration between Karolinska Institutet and SciLifeLab (both Stockholm, Sweden) has led to the development of an AI tool capable of analyzing this information and converting it into useful outputs. This tool is referred to as niche-phenotype mapping (NIPMAP). Researchers integrated two unrelated approaches into NIPMAP. First, AI methods used to identify geographical features, such as conurbations and lakes, were used to map features on images of tumor tissues. Second, approaches used to identify how multiple species inhabit the same area, in a field called community ecology, were used to analyze the roles of individual cells and the relationships between them. This allowed for an intricate understanding of the position and role of individual cells within tumor tissues.
“We realized that the interpretation of tumor images is similar to the interpretation of satellite images and that the relationships between cells in a tissue are similar to the relationships between species in ecology,” elaborates Jean Hausser of the Karolinska Institutet, leader of this research.
The hope is that NIPMAP will be able to interpret the masses of data obtained from modern tumor tissue imaging techniques to identify key information such as which treatment options would be most effective.
Already, researchers have partnered with a cancer hospital in Lyon, France to attempt to use NIPMAP to reveal why only certain cancer patients respond to immunotherapy. A further collaboration with the Mayo Clinic in the USA aims to identify why certain breast cancer patients do not require chemotherapy. NIPMAP and other AI analysis techniques have the potential to drive a far greater degree of personalized and patient-tailored cancer treatment strategies through in-depth analysis of tumor features.