AI-Powered Fatty Liver Disease Monitoring Helps Tackle Growing Global Burden
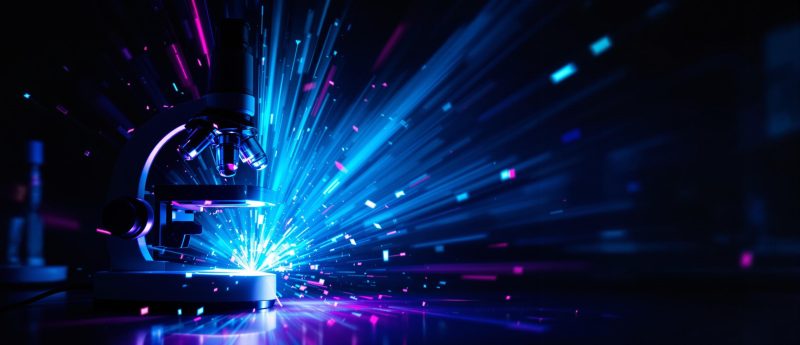
Fatty liver disease is rapidly rising globally but remains hard to diagnose and treat. The EMA’s approval of AIM-NASH and innovations like QP-Liver mark a shift toward AI-driven, precise, and efficient solutions for metabolic liver disease diagnosis and trials.
Exploring Fatty Liver Disease: A Growing Global Health Concern
Metabolic dysfunction–associated steatotic liver disease (MASLD), formerly known as non-alcoholic fatty liver disease, is characterized by excessive fat accumulation in the liver. If left unmanaged, this fatty buildup can cause lasting damage—namely, progressing through different stages of inflammation, scarring, and, in some cases, cancer.
Linked closely with metabolic disease, escalating rates of type 2 diabetes and obesity have echoed a dramatic rise in MASLD cases—over the past three decades, the number of people impacted by MASLD worldwide has increased by nearly 50%. Now, scientists are increasingly acknowledging this global epidemic as a significant health challenge for countries around the world.
Currently, about 38% of the population is thought to have MASLD, affecting more than one in three adults. But in high-risk regions like Southeast Asia and the Americas, incidence can reach as high as 48%.
In the UK, liver disease is now the third most common cause of premature death, with mortality more than doubling in the past two decades. In 2021 alone, 10,521 deaths were recorded in England due to liver disease, signaling a pressing need for innovation in diagnosis and treatment.
While mild forms of MASLD can often be managed through lifestyle interventions, more advanced stages typically require pharmacological treatment, or specialist intervention may be required to prevent further liver damage and manage associated metabolic conditions like type 2 diabetes and cardiovascular risk.
The Limitations of Traditional Liver Disease Trials: Biopsy Bias and Bottlenecks
Owing to its increasing global burden, a number of clinical trials are underway to investigate potential treatments for more progressive forms of MASLD. These trials often rely on liver biopsies—the gold standard for evaluating disease stage, as non-invasive methods often fail to reliably differentiate between stages of liver injury.
An essential component of the biopsy is histological assessment—where clinicians look at tissues and cells under a microscope to assess things like fat accumulation, inflammation, hepatocyte ballooning, and fibrosis in liver tissue. Through these, pathologists can determine how far the disease has progressed and gauge how well a new treatment works.
But there’s a problem. Manual interpretation introduces subjectivity and inconsistency between pathologists, hampering the reliability of trial outcomes—ultimately, this can create bottlenecks for drug development and regulatory approval.
Because of this inconsistency, several trials have failed to demonstrate efficacy—not necessarily due to a lack of therapeutic effect, but because of variability in biopsy interpretation that skews the results.
EMA’s Green Light for AIM-NASH: A Game Changer in Liver Disease Research
In March 2025, the European Medicines Agency (EMA) approved AIM-NASH, an AI-powered pathology tool developed by PathAI, for use in fatty liver disease clinical trials.
The tool assists pathologists by automatically identifying and quantifying key histological features of the disease, such as hepatocyte ballooning, inflammation, fat accumulation, and fibrosis—core criteria used to assess disease severity and treatment response. By reducing inter-observer variability and standardizing evaluations, AIM-NASH enhances the reliability of trial endpoints and supports more consistent regulatory submissions.
AIM-NASH was trained on over 100,000 annotations from 59 expert pathologists, analyzing more than 5,000 liver biopsies across nine large-scale clinical trials.
According to the EMA’s Committee for Medicinal Products for Human Use, the AI system demonstrated greater reproducibility and consistency than consensus-based scoring by human experts, offering a new standard for assessing disease severity and drug response.
Specifically, AIM-NASH achieved a 97% agreement rate with consensus for distinguishing advanced fibrosis (stage F4) from earlier stages (F1–F3), compared to 96% agreement among pathologists. It also demonstrated a strong performance when evaluating whether NASH had improved without worsening fibrosis, agreeing with final decisions 86% of the time—slightly better than the 82% agreement rate among human pathologists.
These findings suggest that AIM-NASH could mitigate reader variability, providing a more reliable assessment of therapeutics in NASH clinical trials.
Beyond AIM-NASH: Other AI Tools Driving the Future of Liver Diagnostics
But AI’s role in liver diagnostics is expanding beyond histopathology. QP-Liver, developed by Quibim, is another AI-based tool that uses magnetic resonance imaging (MRI) scans to assess fat and iron levels in the liver, allowing for early and non-invasive fatty liver disease monitoring.
Unlike traditional imaging, QP-Liver provides automated liver segmentation and quantification of key biomarkers, equipping clinicians with faster and more reliable diagnostic data. As of March 2024, the tool is CE and UKCA certified, signaling its readiness for use across the EU and UK healthcare systems.
Flipping the diagnostic coin, a Cedars-Sinai team recently unveiled “EchoNet-Liver”—a research-stage tool that detects chronic liver disease using echocardiograms, scans typically used to image the heart. During an echocardiogram, the ultrasound beam passes through the upper abdomen, where the liver sits just adjacent to the heart. As a result, clinicians often capture part of the liver incidentally while checking for signs of cardiovascular disease. This is like a diagnostic byproduct—usually ignored during the procedure, but a potential reservoir of screening gold.
It’s this goldmine that EchoNet-Liver taps into. Ordinarily, these byproduct scans wouldn’t offer much clinical value—they lack the resolution and sensitivity to detect key signs of liver disease, like fat accumulation and fibrosis. But EchoNet-Liver, trained on 1.5 million echocardiogram videos, can pick out subtle patterns—liver textures or shapes—that a human eye might easily overlook. According to the researchers, the AI performed comparably to human radiologists interpreting traditional imaging scans, such as MRIs or abdominal ultrasound scans, for liver disease detection.
“Our deep-learning model can help doctors spot liver disease that might have gone unnoticed and thus direct appropriate follow-up testing,” said said David Ouyang, MD, a cardiologist in the Department of Cardiology in the Smidt Heart Institute, an investigator in the Division of Artificial Intelligence in Medicine, and a senior author of the study.
While not as powerful as QP-Liver, tools such as EchoNet-Liver could provide an easy and inexpensive way of flagging the patients who need further investigation. Think of it like a pyramid: At the base (the initial screening stage), the “basic” equipment is deployed widely. As patients are directed towards the right care, the pyramid ascends to “bigger and better” models with greater diagnostic performance, selectively available to more specialized departments.
What’s more, EchoNet-Liver lends itself to a distilled process of multi-disease monitoring, where a singular visit could spotlight multiple problem areas—in this case, both the heart and the liver—enhancing early detection of diseases that may have otherwise gone unnoticed until later.
“These novel findings exemplify how AI models are helping us to augment clinical diagnostics at a body-systems level instead of just individual organs,” said Sumeet Chugh, MD, director of the Division of Artificial Intelligence in Medicine at Cedars-Sinai.
AI’s Growing Influence on Global Health and Clinical Research
The integration of AI into healthcare aligns with global digital transformation efforts. The World Economic Forum highlights how AI tools are already assisting with fracture detection, stroke care, and optimizing surgical decision-making.
And, in the context of fatty liver disease, AI has the potential to replace invasive liver biopsies with non-invasive, scalable technologies, accelerating drug development timelines and reducing trial costs.
Perhaps an underappreciated yet equally important potential of AI is its ability to standardize diagnostic assessments—minimizing inter-observer variability, and enhancing the reliability of clinical trial outcomes. By providing consistent and reproducible evaluations, AI-driven tools contribute to more accurate patient stratification and treatment monitoring, ultimately improving patient care and advancing therapeutic innovations.
Currently, Madrigal Pharmaceuticals’ Rezdiffra is the only FDA-approved treatment for MASH in the U.S., and other pharmaceutical companies such as Eli Lilly and Novo Nordisk are in advanced-stage trials.
The standardization offered by AIM-NASH could provide these trials with more reliable endpoints for researchers, boosting a drug’s chance of success through the pipeline.
Conclusion: A Data-Driven Leap Forward for Global Liver Health
The approval of AIM-NASH by the EMA is a landmark in clinical research—ushering in a future where AI reduces human bias, enhances diagnostic accuracy, and accelerates therapeutic innovation.
As AI tools like AIM-NASH and QP-Liver become more integrated into trials and clinical practice, they promise a new standard for how liver disease is diagnosed, monitored, and treated. This shift toward digital pathology and imaging has the potential to improve patient outcomes and contribute to a more consistent and accessible approach to liver disease diagnosis and management globally.