AI to Guide Surgical Decisions in Breast Cancer
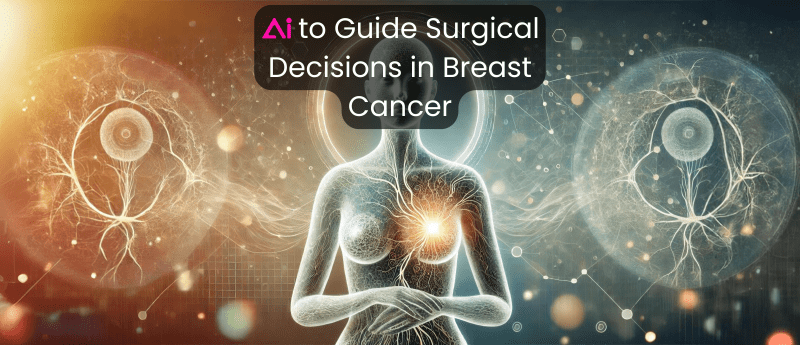
Researchers have developed an MRI-based AI model to accurately decide which cancer patients need a surgical procedure and which ones don’t. Traditional imaging techniques often fail to do so, overlooking some patients where vital surgery could mean the difference between life or death.
In breast cancer patients, the spread of cancer to the lymph nodes in the armpit is a key factor in predicting how the disease will develop. Consequently, differentiating patients whose cancer is likely to do this serves an important role in optimizing treatment.
Despite this importance, current imaging techniques largely struggle to accurately differentiate these patients, meaning clinicians could miss patients who need complete lymph node-removal.
In this case, some of the cancer cells in the axillary lymph nodes will be removed, but some cancer cells are left in the remaining lymph nodes.
In a study published in the journal Nature, Honbing Luo and colleagues at the Sichuan Cancer Hospital & Institute (China) aimed to address this by improving AI’s ability to predict the spread of breast cancer to the lymph nodes of the armpit.
While deep learning models have already been developed in this context, the researchers optimized the technology by the inclusion of a new clinical phenotype into the AI’s algorithm: peritumoral edema (PE). This is a phenomenon where extracellular fluid surrounds the tumor, which then shows up as a high-intensity signal on an MRI scan.
However, the group also incorporated multiple parameters into the model, to paint a more refined picture of what features can accurately predict those with a high ALNM burden. Crucially, the group reported that PE can strongly enhance the performance of the AI.
The optimized model was able to effectively discriminate between 96.3% of cases in a real-world setting. Alongside this, their model demonstrated a negative predictive value (NPV) of 95.2% – that is, the AI’s ability to accurately exclude patients who did not have a high ALNM burden.
The group compared their results to reports on previous models which sample from a smaller pool of features, one of which could only correctly discriminate between 69% of cases.
This substantial increase in discriminative power is strong evidence that AI models which analyze multiple types of information at once may be more accurate than those with smaller parameters.
As such, AI can help clinicians to correctly identify breast cancer patients who need more invasive surgeries. In a broader sense, this has wider implications for cancer imaging techniques.
Currently, imaging tools may miss patients who need certain treatments or procedures. However, the advent of AI has the potential to drastically improve this area, leading to better accuracy and, importantly, more lives saved.