AI Tool, TxGNN Speeds up Drug Repurposing for Rare Diseases
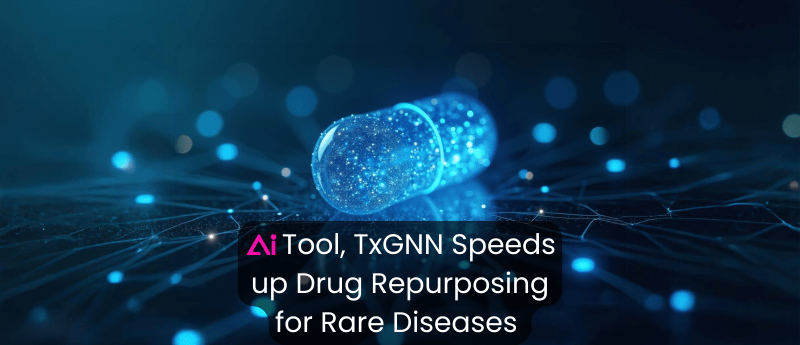
Innovative drug discovery approaches are essential for finding effective treatment. Harvard researchers have developed a new AI tool, TxGNN, that aims to boost drug repurposing and bridge the treatment gap in rare diseases.
The research was led by Marinka Zitnik and her team at Harvard Medical School and the findings were published in Nature Medicine. TxGNN is a graph-based foundation model for zero-shot drug repurposing. There are over 7,000 rare diseases worldwide and, to date, only between 5% and 7% of rare diseases have an FDA approved drug.
This leaves the majority of rare conditions without effective medical interventions, creating significant gaps in care and limiting treatment options. The lack of widespread treatment solutions underscores the need for better drugs and more effective treatments for rare diseases.
Drug discovery is a time-consuming and expensive process that, on average, takes between 12 to 15 years to complete, from research to the approval process. TxGNN resembles similar reasoning that clinicians use by identifying possible drug candidates, both experimental and approved; reporting on side effects and explaining the rationale for decisions.
To inspire further research for treatments, TxGNN is available for free.
TxGNN: How does it work?
TxGNN uses a unique graph neural network (GNN) for zero-shot drug repurposing to identify potential drug candidates by examining shared genomic and biological features across diseases.
TxGNN is trained on a comprehensive medical knowledge graph, (KG), which includes biomedical data. This extremely extensive dataset enables TxGNN to identify and process various therapeutic tasks, such as predicting indications (suitable uses for a drug) and contraindications (circumstances where a drug should not be used), in a transparent format that makes drug discovery significantly easier for researchers.
During the initial experiments, TxGNN successfully identified potential uses for nearly 8,000 existing medicines in treating over 17,000 diseases, including many currently without any approved treatments. This is the largest number of diseases addressed by a single AI model to date, demonstrating TxGNN’s exceptional capabilities.
The tool was trained on large quantities of data including genomic data, cell signaling information, gene activity data and clinical notes. The researchers tested the model by asking it to perform various tasks and verify its performance on 1.2 million patient records. It was then asked to select drug candidates for various diseases and generate additional predictions.
A key component of the TxGNN model is its Explainer module, which enhances transparency by revealing how the AI tool generates its drug predictions through multi-hop connections within a medical knowledge graph. This feature enables researchers to understand the reasoning behind the model’s recommendations, making TxGNN’s decisions more interpretable and reliable for clinical applications.
Zero-Shot Inference: A Paradigm Shift that Sets it Apart
One of the most fascinating aspects of TxGNN is its ability to perform zero-shot inference.
This means that TxGNN can predict the effectiveness of a drug-disease pairing with no prior data. This broadens its capabilities to analyze a far wider range of diseases. Most traditional AI models for drug repurposing are trained on specific datasets to make predictions for diseases but with zero-shot interference, there is no need to extensively retrain the model on each identified disease it is tasked with.
Key Findings
TxGNN was benchmarked against eight leading methods, including a natural language model, BioBERT, graph neural networks, HGT and HAN, and network medicine statistical approaches. Under standard evaluation, where training data included some disease indications or contraindications, TxGNN surpassed HAN, the top performer, by 4.3% in AUPRC for indications.
The team then further assessed models in a zero-shot repurposing scenario. In this setting, Zitnik noted that TxGNN improves the accuracy of its nearest AI competitors by 49.2% for indications and by 35.1% for contraindications.
These results are especially noteworthy as traditional models often falter in zero-shot tasks. TxGNN’s efficacy was also validated across nine disease areas, achieving AUPRC gains ranging from 0.5% to 59.3% for drug indications and 11.8% to 35.6% for contraindications.
Looking Ahead
The development of TxGNN offers significant hope for discovering new therapies for rare diseases that have limited or no treatment. It consistently outperformed existing drug repurposing methods by offering multi-hop interpretable reasoning for its predictions which boosts trust and transparency for researchers.
“With this tool we aim to identify new therapies across the disease spectrum but when it comes to rare, ultrarare, and neglected conditions, we foresee this model could help close, or at least narrow, a gap that creates serious health disparities,” said lead researcher Zitnik.
It’s a versatile tool and the Harvard team believes that with further application, TxGNN could identify even more promising drug repurposing opportunities that could revolutionize the field of drug discovery.