An AI tool for predicting cancer patient survival outcomes
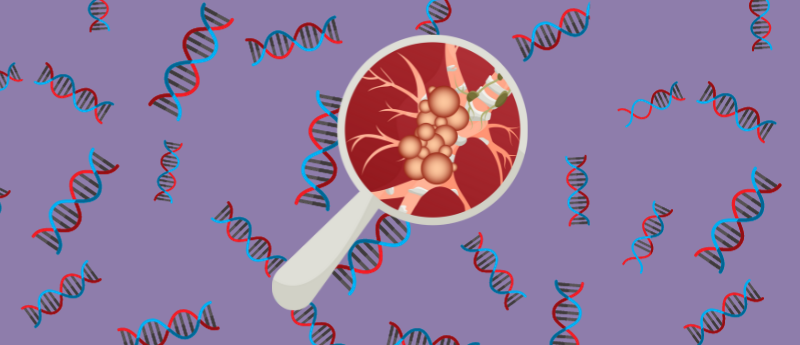
In a study led by UCLA Health Jonsson Comprehensive Cancer Center (CA, USA), a pioneering AI model has emerged. The model analyzes the gene expression patterns of epigenetic factors in tumors to accurately predict patient outcomes across a spectrum of cancer types. Particularly encouraging was the model’s ability to predict clinical outcomes more accurately when compared to traditional prognosis metrics such as cancer grades and epithelial-to-mesenchymal transition.
In the past, cancer has been attributed to genetic mutations within oncogenes or tumor suppressors. However, recent advances in next-generation sequencing technologies emphasize the critical role of chromatin state and the levels of epigenetic factors in the initiation and progression of cancer.
To investigate the correlation between epigenetic patterns and clinical outcomes, the team of UCLA researchers scrutinized the gene expression patterns of 720 epigenetic factors across 24 different cancer types. Remarkably, for ten of these cancer types, distinctive clusters of epigenetic patterns were significantly linked to variations in patient outcomes, including progression-free survival, disease-specific survival, and overall survival.
The identified clusters associated with poorer outcomes correlated with higher cancer stage, larger tumor size, and greater likelihood of tumor spread. Furthermore, certain tissues of origin for five specific cancer types exhibited a higher prognostic efficacy of epigenetic factors than grade or epithelial-to-mesenchymal transition, underlining the cancer-specific relevance of therapeutically targeting these factors. These five cancer types comprised adrenocortical carcinoma, kidney renal clear cell carcinoma, brain lower grade glioma, liver hepatocellular carcinoma, and lung adenocarcinoma.
To validate their discoveries, the team developed an AI model trained using epigenetic factor gene expression levels. The model effectively classified patients into two groups (significantly higher chance of better outcomes and significantly higher chance of poorer outcomes) for the five cancer types with higher prognostic efficacy, confirming the noteworthy differences in survival outcomes. Notably, the genes deemed most crucial by the AI model showed a significant overlap with the cluster-defining signature genes.
This is just one study among many that adds to the wealth of medical AI prediction tools currently being developed. Michael Cheng, the study’s primary author, highlighted the importance of their research, stating, “Our research helps provide a roadmap for similar AI models that can be generated through publicly-available lists of prognostic epigenetic factors. The roadmap demonstrates how to identify certain influential factors in different types of cancer and contains exciting potential for predicting specific targets for cancer treatment.”