Artificial Tumors to Help AI Detect Early-Stage Cancer
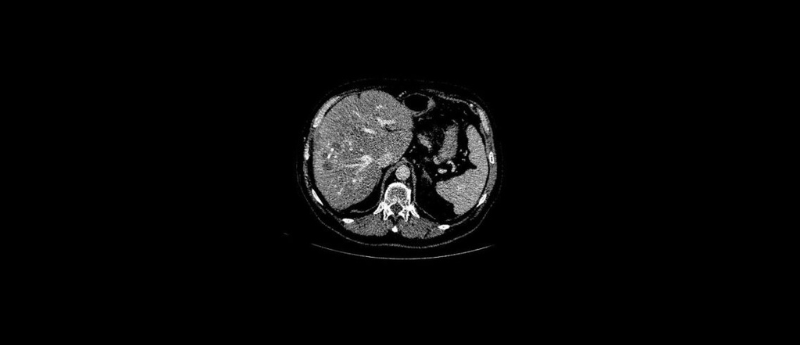
A Johns Hopkins University-led (MD, US) research team has designed a method to generate enormous datasets of artificial, automatically annotated liver tumors on CT scans. This is enabling AI models to be trained to accurately identify real tumors without human help.
Led by Alan Yuille, a Bloomberg Distinguished Professor of Cognitive Science and Computer Science and the director of the Computational Cognition, Vision, and Learning group, the team will present its research at next month’s Conference on Computer Vision and Pattern Recognition.
The team’s work could play an important role in solving for the scarcity of high-quality data needed to train AI algorithms to detect cancer. This shortage stems from the challenging process of identifying tumors on medical scans, which can be extremely time-consuming, often relying on pathology reports and biopsy confirmations.
For example, there are only about 200 publicly available CT scans with annotated liver tumors—a minuscule amount for training and testing AI models to detect early-stage cancer.
Collaborating with radiologists, the team devised a four-step method of creating realistic synthetic tumors. First, they chose locations for the artificial tumors that avoided collisions with surrounding blood vessels.
Next, they added random “noise” patterns so that they could generate the irregular textures found on real tumors, and generated shapes that reflected real tumors’ varied contours.
Last, they simulated tumors’ tendency to push on their surroundings, which changes their appearance. The researchers say that the resulting synthetic tumors are hyperrealistic and have passed the Visual Turing Test—that is, even medical professionals usually confuse them with real tumors in a visual examination.
The team then trained an AI model using only these synthetic tumors. The resulting model significantly outperforms similar previous approaches and can achieve comparable performance to AI models trained on real tumors, they say.
“Our method is exciting because, to date, no existing work utilizing synthetic tumors alone has achieved a similar or even comparable performance to AI trained on real tumors,” says team member Qixin Hu, a researcher from the Huazhong University of Science and Technology.
“Furthermore, our method can automatically generate numerous examples of small—or even tiny—synthetic tumors, which has the potential to improve the success rate of AI-powered tumor detection. Detecting small tumors is critical for identifying the early stages of cancer.”
According to Yuille, the team’s approach can also be used to generate datasets and train models to identify cancer in other organs that currently suffer from a lack of annotated tumor data.
The team is currently investigating advanced image processing techniques to generate synthetic tumors in the liver, pancreas, and kidneys, but hopes to expand to other areas of the body, as well.
“The ultimate goal of this project is to synthesize all kinds of abnormalities—including tumors—in the human body to be used for AI development so that radiologists don’t have to spend their valuable time conducting manual annotations,” says Hu. “This study makes a significant step towards that goal.”
The team’s dataset and corresponding code are available for academic use, and Yuille has shared a video describing the team’s research.