Behind the Paper | A New Frontier for Personalized Cancer Treatment
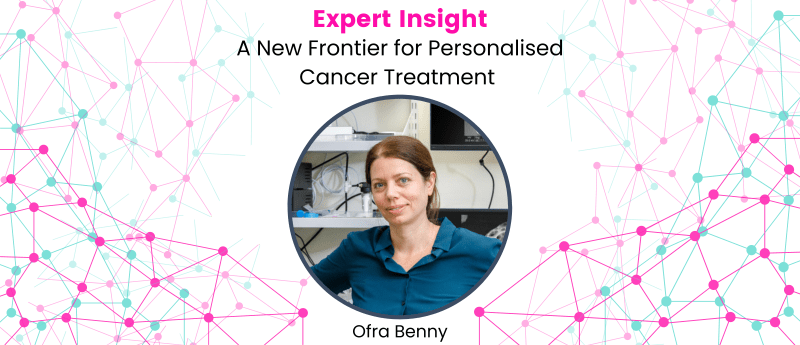
This is a peek behind the scenes of some extremely exciting research to develop a revolutionary method of classifying cancer cells. Called ‘mechanomics’, the method uses an AI algorithm to analyse uptake of fluorescent particulates into cancer cells. The uptake serves as a proxy for the structural phenotype of each cell, allowing it to be classified from other visually indistinguishable cell types. It’s ultimate implication is to enable prediction of malignancy and resistance in patients.
In this interview we chat with Ofra Benny, who led the project. She talks to us about the imperative use of computational methods to achieve astonishingly accurate results, as well as ongoing work to integrate the method into hospitals for rapid personalised treatment services (using organ-on-a-chip technology). We discuss model training using known cancer phenotypes, Ofra’s business endeavours, the transformative nature of AI in research, and the importance of interdisciplinary collaboration (amongst a number of other topics).
Table of Contents
Techniques- Flow Cytometry and Fluorescent Labelling
Implications- Predicting Recurrence and Drug Responses
Introduction
So I’m Ofra Benny, professor in pharmaceutical sciences here at the Hebrew University in Jerusalem. I did all my degrees at the Technion in Israel which is kind of the equivalent of MIT.
So I’m coming from an engineering background and then I did my postdoc. I started as biotechnology engineer and then I went to a long postdoc at Harvard Medical School. I did my postdoc in a very well-known lab of Judith Walkman. He was a very well-known scientist and researcher and unfortunately passed away when I was there. I was his last postdoc but yeah it was a unique experience.
But luckily you know I had the chance to work with someone like him who was giant you know it was very creative and I came for more from engineering and materials and then I started to dive in the field of cancer, cancer research, cancer biology and all over my career. I’m kind of integrating these two disciplines.
So I always have engineering, have biology or biological sciences and recently with the emergence of all the AI tools and the machine learning we realized how much it can contribute to our field of biology and cancer research and gradually we started to have more and more projects that involving AI.
So I’m running a relatively big lab of 15 students and team members that focus on bioengineering and integration of biology and engineering. That’s more or less what we do and all our projects are aimed to address really unmet clinical needs.
One of the advantages that we have here in this atmosphere that we have specifically in the university in my campus is that the pre-clinical side which is the research side we’re physically very close to a hospital so we are communicated a lot with clinicians and in that way it’s really important because we know we address real questions and not like something we make in our own heads.
The Project
So this project started a long time ago when we realized that in cancer much of the selection of the therapy that is given to specific patients many of these decisions are based on a trial and error kind of or on fixed protocols. So basically a patient comes and diagnosed with a certain cancer and give him or her very fixed protocols for first line therapy. In many cases the disease come back and reoccur and the patients cannot really benefit from the therapy anymore and then the oncologists need to give them something new but they have no idea what to give them sometimes.
So today as you probably know there are many advances in genomics for example so they can have genomic profile of the patients. They can find specific mutations that give them some ideas of what therapy to select. But in most cases there is not enough information even in the genetic analysis that can help the oncologist to give really a rational selection of drug of therapy. So we realized that this is a big problem.
Eventually we want to give the patients the most personalized therapy, the best optimized therapy for this specific person and we understand that there is no not enough information that can be translated to the real life because it’s highly complex. Especially when you start to analyze single cell.
So we started to say wait a minute so we need to find some more general principle for for predictions for for trying to predict how the cancer cell will behave. So we wanted to be able to measure something that is already a result of many parameters.
At the same time we had some big research in my lab that found very a very interesting correlation between cell mechanical properties, meaning the stiffness of cancer cells and their ability to to be aggressive or less aggressive. So we said okay, this is very interesting so maybe we can just measure mechanical properties of cells. Let’s say you can squeeze cells by AFM or whatever and you can say whether they are very stiff or very deformable. So that was another paper that we published also in Science Advances two years ago and we found that indeed there is a correlation between a mechanical properties and behavior or function of cells.
For example the cancer cells that are more deformable they are also more aggressive, which makes sense because they know how to move. So this is one aspect that’s nice, but you to be able to measure that this is extremely difficult because now you have to squeeze each cell. It doesn’t make sense.
But then another big advance that we did, which is really what we had in this project, is to to use actually a kind of a proxy measurement for that [cell deformation] so we can use particles, solid particles, nano or micro particles, and we found that actually the uptake of these particles are really dependent on the ability of cells to make these deformations.
So imagine that you have some a balloon that has to engulf something. It has to change shape. So one aspect is the fluidity of the membrane and the second aspect is actually the formation, all the skeleton has to really change shape. So we said okay so we want to measure both.
What we’ve done and this is really interesting. We took a panel of particles, each coated with a unique color. So we have let’s say small nanoparticles are red, medium particles are green, and the large particle are blue and it’s always like that.
So now we take these particles and we incubate them with cancer cells. We started to measure the spectral a signal the colors basically by moving the spectra and then looking at the colors. Using this you can know how much of the particles were taken up by the cells. It’s really nice.
It’s hard to explain it to sometimes all the rationale, but that’s how it worked really.
It was a journey that we started with understanding the mechanical properties, and we realized that uptake can be a proxy measurement. And now this is the step, the huge step that we did now, is really by looking at patterns of uptake. And now the machine learning was so useful for us, we can actually link between uptake and function.
So what we’ve done was to start to do the learning phase, the training. So we did a lot of analysis, we took one population of cells that we knew is more aggressive than others, because we did this, you know, it’s a kind of bias approach here at the training, because it’s supervised basically.
So we give, we index the cells, we said this population is very aggressive because we measure them, and this one is not aggressive. Now I want you to look at all the uptake patterns, but in a single cell.
So we can look at the single cell on these uptake patterns. And then we did the same thing with resistance to certain drugs. So we looked at the drug resistance, we looked at the aggressive invasiveness of cells. And after the training session, then we could give again a mixed population and ask the machine now that they give you only uptake information. Now you have to tell me how much of these cells are really, you know, aggressive, how much they are. So this is the point of this study. It’s very nice. We got very high accuracies.
I want to say something that is very critical here because one of the controls that we did, we said, okay, but basically if the machine is so smart, maybe we can just let it look at the cells, you know, look at the morphology or because the function is so linked to physics, to the physical properties of the cells. Maybe we can just give the size, you know, the granularity or whatever. But then we took a subpopulation of cells that look alike. They look very, very similar. But we knew that the one subset is more invasive and the second one is non-invasive. But they look very similar physically. And then we did the same test. We put these particles, we looked at the uptake patterns, and then it was able to find it. But if you would look at them, you wouldn’t see this differentiation.
Empowering Research with AI
I don’t want to take away from the really interesting thing that you’ve achieved, but just to talk a little bit about the how the the use of machine learning has impacted your research here, because I actually had a meeting just before this with someone else doing some research. And I think an interesting point of conversation is how in academia you guys access machine learning and particularly the compute that you need to be able to achieve this.
Yeah, no, it’s a good point because this is an emerging technology for us and I’m sure that the impact will be super valuable for medicine and for biology. And we understood where my, you know, I’m not coming from this area, but I recognize how critical it is because I knew there are patterns that we cannot see in use in analytical way.
I mean, it’s statistical, a statistical method. So I was sure of that. And then I knew that I have to do this link with experts in AI. So I actually I think in academia, it’s very important to collaborate at this stage because it’s very hard to be an expert on everything. That’s the situation.
So I think today, if you ask about the impact, the impact is for sure is revolutionary. I mean, it’s going to change the medicine and human health and I’m sure that and we already start to see the initial, you know, we start to glimpse on how to see the beginning of all that with the genomics and even drug design, all this. And it gives us an opportunity to start with personalized medicine.
I mean, it’s amazing. We couldn’t do this study otherwise because we had so much, the big data is really big. Single cell and I’m sure there will be a lot of this in the coming years, but at this point, the access to have the accessibility to this, I think it’s for collaborations for now, at least in academia, in academic setting. That’s what I think.
And yeah, because you have to defend your work somehow. I’m not an expert in all, so I have to have someone that will be able to, you know, to lead this and to train the students.
Yeah, I think that’s really interesting and I think you’ve hit the nail on the head there and it builds on the point that people say like “AI isn’t going to replace people, people who use it are going to replace people who don’t” in a way. And it sounds like it has empowered your research.
Is it exciting for you to be able to have this new tool in your box that you can accelerate your research or do new things with this idea that you’ve got? Is it something that’s exciting for you?
Yeah, no, I feel that this is a very critical tool that we cannot, you know, if for now we used to be very kind of conservative with classical methods that we use for many years as researchers coming from biology, but I feel that we have to open our mind now because this is another era, we have to be there. I think we start to see that, I mean, all the innovations now involve big data.
People think that it [AI] will replace the researchers eventually. But I think in the coming years, we will need still to generate the data to ask the questions, but I’m not ruling out by the way. It might be that one day the machine will beat us, it might be.
No, I think it’s so interesting to talk to someone like you about, you know, because I’m interested in both sides of it. And, you know, I don’t think AI is the solution to everything by any means. I’m not trying to say like, oh, it’s gonna, you know, hopefully it will solve a lot of our problems. But it’s really interesting just to see how it’s been been used. And that’s what I want to share, like, through this platform that we’ve got, I want to share with people how is this stuff being used and hopefully give other people, other researchers inspiration about how they can use it in their own work.
Techniques- Flow Cytometry and Fluorescent Labelling
We had a debate whether to use imaging indeed or to go to other types of data, because we could do imaging. In our case it was limiting for us to use imaging because we rely on our detecting fluorescence. And then in imaging, sometimes you have overlays, it’s technically quite difficult to do. So we decided to go with some other technology, which is flow cytometry.
So in flow cytometry, what we can do is to measure fluorescence, but it gives you the fluorescence in a couple of colors you can do at the same time, which is important. So you have a spectral measurement, but the importance of flow cytometry is that it can give it to you in a single cell and in large numbers.
So potentially we could do imaging, but we decided to go with flow cytometry because of the throughput.
It’s relatively simple to do, you don’t have to use a microscope, you don’t have issues of overlay between colors and so on. So in our case, it makes sense to do it by flow cytometry, but definitely imaging is an option.
I mean, we could do a lot of microscopic imaging and then to do machine learning, like image analysis. Yeah, I mean, potentially it could work by imaging as well. But we decided to focus on this flow cytometry, it’s a single cell. It’s basically what I was trying to coin this term in the paper that I call it “mechanomics”, because it’s kind of a new types of omics. It’s not genomics, it’s phenotypic omics.
So actually, I’m able now to give a lot of information in a single cell, like multiplex kind of, but this is already a phenotype and not specific. It’s hard to explain what I mean here, but it’s not the lego, you know, the each lego part, it’s actually already an emergent phenomena that you are measuring. So it’s an interesting concept that you can look at the phenotypes and indeed in imaging you also can look at phenotypes because you see behavior of the whole organism, or something that is some of a lot of parameters. You already measure something that sum up a lot of factors.
Yeah, I think “mechanomics” is a great term as well. I think it fits very nicely what you’ve described in your work. And I guess that leads on quite nicely to what do you feel the implications of this, what you found are moving forward in terms of not just your research, but potentially clinically as well.
Implications- Predicting Recurrence and Drug Responses
Yes, so eventually what we aim to do is to take it to the clinic. So we have already a plan to start a clinical validation. So what we do is we take biopsies from patients, from cancer patients, we do the same analysis, and now we have to wait and correlate it with the clinical outcome.
So, for example, we’re looking at recurrence. This is one parameter, so this is recurrence. Let’s say the patient is being treated and you can predict whether this patient will respond for a long time, or maybe the disease will recur. And also I want to be able to predict resistance before they even start treatment. So this is what we’re trying to do.
I will mention here that we already have a spin-off company that’s called PreQ that is working on organ-on-a-chip, if you know this technology.
So basically this company, what we do is we take biopsies from patients here from Adasa Hospital in Israel. We grow the samples, but instead of one tumor, now we do hundreds of them so you can screen drugs. And I can give the oncologist an answer, whether they’re a short list of drugs that are debating what drug to give the patient, we can tell them this one works, this one is the patient is resistant.
So it’s now in clinical trials. Yeah, it’s really cool. So I think we will be able to integrate this technology with the mechanomics. Maybe next step will be to somehow to merge it with this organ-on-a-chip technology, and then it’s really personalized medicine.
So you take samples, you do the same analysis on a specific person, you can ask specific questions, but of course the training will have to be very specific for a clinical, like a narrow clinical question, for example resistance or invasion or something like that.
Yeah. Wow, that’s a really powerful implication of what you’re doing.
Other Business Endeavours
What’s your involvement with this? Are they associated with the university? Did you say they’re a spin out of the university?
Yeah, actually I’m the CSO. Yeah, I’m a professor here. I have already four startups that I started for my lab. Actually, each one is in another field. One is a drug for ophthalmology. It’s a compound for AMD, for wet AMD.
So you have a compound for it?
Yeah, we have something very exciting. It’s unrelated to this conversation, but it’s actually anti-angiogenic drug that is not dependent on VGF. Yeah, so it’s really nice because it’s a new mechanism. We think we will be able to combine it or to use it for patients that has only partial response when they finish the Lucentis or Avastin or whatever. All the drugs today target VGF, right, in the AMD. And this is a completely new mechanism of action. So it’s very promising.
And we are working also with the head of the ophthalmology department here. It’s very nice. I think it’s a promising drug.
Your research or your work spans across domains, then, so you’re not just focused on cancer?
No, not only on cancer. Most of my work is cancer or angiogenesis, like the formation of blood vessels. And this is relevant for other diseases as well, like disease in ophthalmology, in the skin.
So the motivation is usually we start with the unmet need, like we try to understand what is missing now, what solution needed to be solved using technologies and advanced materials and so on. And then we try to really tailor the solution per the problem.
So we have medical devices, for example, we develop some drug-eluting devices, kind of implants. Many solutions that are half engineering, half biology, but it’s always in the context of clinical issues that we are trying to solve. So that’s more or less what my lab do.
I mean, we have a big panel of projects that all of them, almost all of them are very applicable projects. But sometimes we have also basic science. I mean, in this case, for example, we started with the really basic research that we realized how the mechanical properties affect function. And that was very interesting. But then it led us to this application.
Yeah. It’s a very neat curve of, it sounds almost like, I’m not saying you got lucky, but it sounds always lucky that you found this relationship. Because this is the kind of thing that sometimes there seems to be gaps of years between someone finding the mechanics and then application.