Beyond radiology: the role of AI in healthcare
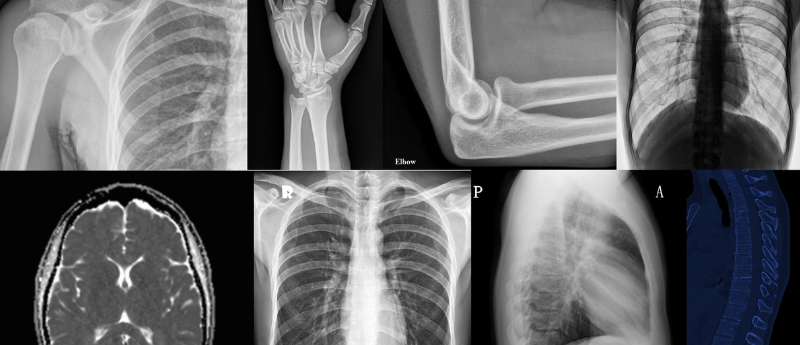
In this opinion piece, Darren Stephens (Qure.ai, UK) explores the impact of AI on the medical diagnostics community, how radiology AI-enabled solutions can reduce strain on the NHS (including Qure.ai solutions) and some obstacles associated with the widespread application of AI in the NHS and other primary healthcare systems.
As Senior Vice President & Commercial Head for the UK and EU at Qure.ai (Mumbai, India), I see first-hand the impact of AI on the medical and diagnostics communities. Over my career, I have gained fantastic insight from working in pharmaceuticals, infection prevention, point of care, diagnostics, teleradiology, and managed services across pathology and imaging. Within my role at Qure, I get to bring this holistic experience to the team to ensure we build the right structure, processes and systems to best support the end users of our solutions and, more importantly, to ensure that patients get access to affordable and timely care across the markets we serve.
Globally, Qure.ai is one of the most reputed names in the AI medical imaging space. From lung cancer to tuberculosis, stroke & intracranial haemorrhages to musculoskeletal fractures, our CE and FDA-certified solutions can identify signs of diseases on X-rays and CT scans and help radiologists to make faster and more accurate decisions. Qure’s products are deployed in over 70 countries, impacting more than 12 million patient lives.
In the UK, we work with over 25 NHS Trusts, the first of which was the Royal Bolton Hospital (RBH). In April 2020, it became the first NHS Trust to adopt our AI-aided technology in radiology. Our AI solution, qXR, was utilised by clinicians and radiologists to report chest X- rays as a secondary reader to ensure no potential COVID cases were missed, reduce reporting turnaround times, support clinical decision-making for better patient outcomes and to help alleviate hospital staff workloads.
The Medica Group Plc. is one of the leading teleradiology providers in the UK, and over 100 NHS hospitals utilise its services. qER, our AI solution to screen head CTs, has been integrated into Medica’s emergency night-time service to enhance report times and ensure that no intracerebral haemorrhage or infarct is missed.
We have also partnered with clinical stakeholders for AI-enabled chest X-ray triage for faster and earlier lung cancer diagnosis. Currently, the average time to diagnosis for lung cancer patients can be as much as 62 days, with NHS England’s Faster Diagnosis Standard (FDS) target as no more than 28 days. I believe AI can contribute to reducing this time significantly.
At present, the NHS is in the same boat as other healthcare systems across the world. That is, taking slow, steady steps to emerge from the shadow of the COVID pandemic and facing up to a staggering waiting list of patients, some of whom were able to defer treatment and others who were simply unable to access diagnostic services and are now in danger of diseases having progressed. And that is not all.
The NHS is purportedly operating with 154,000 fewer full-time staff than it needs, as well as the possibility of significant budgetary shortfalls. With finances coming under severe strain due to rising staffing costs and mounting energy bills, the need to achieve better patient outcomes looks like an increasingly steep mountain to ascend.
In this scenario, AI-enabled solutions quietly support stakeholders’ critical roles across the medical value chain. In the NHS, AI is utilised in myriad ways to improve healthcare delivery, patient outcomes and operational efficiency. For example:
- Radiology: AI-powered tools analyse medical images, such as X-rays and CT scans, to help radiologists make more accurate diagnoses, which can help reduce waiting times and improve patient outcomes.
- Administrative tasks: AI-powered Robotic Process Automation (RPA) tools are helping automate routine yet time-consuming administrative tasks, such as scheduling appointments, managing patient records, and processing insurance claims. This can free healthcare professionals to focus on more complex tasks, such as patient care.
- Disease management: Be it prostate cancer, coronary heart disease, or eye ailments, AI is utilised to help identify patients at risk of developing chronic diseases. This allows healthcare providers to intervene early, provide preventive care and manage the conditions more effectively.
If we make an effort to broaden our horizons from the micro to macro level, it is clear that AI can offer huge direct and indirect benefits that go beyond radiology departments. So yes, in the near term, conversations are dominated by the value AI brings to radiology and how time – to evaluate the right AI solution and train medical personnel on using it well and money – to purchase and integrate the AI solution within existing workflows are needed to encourage widespread adoption.
However, AI solutions in radiology are bound to have a ripple effect across the health ecosystem and impact many other aspects of healthcare. Reducing wait times must be an absolute priority for the NHS, and AI can assist in achieving this goal after the complete patient journey is traced. Let us face it; the better the understanding of the clinical workflow and clearly identifying potential bottlenecks, the better the AI-powered solution and pathway design. So, mapping the patient journey in microscopic detail will allow AI solutions providers to better analyse bottlenecks and develop tools to address specific issues. These can include:
- Patient engagement: AI-powered chatbots and virtual assistants can help patients get quick and accurate advice about their symptoms and direct them to the appropriate care setting. This can help reduce the number of unnecessary visits to emergency departments and primary care providers, freeing up resources for those who need them most.
- Predictive analytics: AI can predict patient demand for specific services and allocate resources accordingly. For example, AI-powered models can forecast the number of patients needing a particular type of surgery or test and thus schedule appointments to reduce wait times.
- Resource allocation: AI can optimise resource allocation, such as staff and equipment, to minimise wait times. For example, AI-powered scheduling tools can help match patient demand with available resources, ensuring patients receive care as quickly as possible.
- Workflow optimisation & triage: AI can help streamline clinical workflows, reducing bottlenecks and wait times. For example, AI-powered tools can help healthcare providers prioritise patients based on urgency and ensure that tests and procedures are completed promptly.
- Patient flow management: AI can track patient flow through hospitals and clinics, identifying bottlenecks and allowing healthcare providers to intervene before they become problematic. For example, AI-powered tools can monitor patient wait times in emergency departments and alert staff when wait times exceed a certain threshold.
Suppose we were to cast the net beyond wait times. In that case, drug discovery is also a huge area of need, wherein AI can help researchers analyse vast amounts of data to identify new drug targets and potential treatments. This could speed up the drug discovery process and bring new therapies to patients faster.
But some obstacles associated with the widespread application of AI in the NHS and other primary healthcare systems must be surmounted.
- Data quality and interoperability: AI relies on high-quality data to function effectively. Organisations should prioritise data quality and invest in data standardisation and interoperability initiatives to ensure that AI models can access high-quality data.
- Regulatory and ethical considerations: The use of AI in healthcare raises ethical and regulatory issues, such as data privacy, transparency, accountability and bias. Hospitals must develop clear ethical and regulatory frameworks for the use of AI in healthcare and engage with relevant stakeholders to ensure that these frameworks are transparent and inclusive.
- Lack of trust and understanding: Healthcare professionals, patients and the public may need more understanding about using AI in healthcare. Some may view AI as a threat, while others may be concerned about the accuracy and reliability of AI-powered solutions. These concerns must be addressed in simple, understandable and trustworthy ways.
- Cost and resource constraints: Deploying AI-powered solutions requires resources, including investment in hardware, software and training. These funding pathways must be clarified to enable experimentation and faster adoption.
Currently, AI is being utilised in the NHS and elsewhere to assist clinicians by addressing pressing issues and gaps in radiology. Ecosystems like the NHS need to adopt and embrace AI technologies to ensure that we can get the most out of our valuable clinical resources. In the long term, translating AI’s benefits across departments and the health landscape requires careful planning and a collaborative and inclusive approach that involves healthcare professionals, patients, regulators and other stakeholders to ensure that AI-powered solutions are effective, safe and equitable.
Author profile:
Darren Stephens, Qure.ai
Darren is the Senior Vice President and Commercial Head of the UK and Europe. He brings a wealth of experience gained from working in pharmaceuticals, infection prevention, point of care, diagnostics, and managed services across both pathology and imaging to deliver solutions across Europe including the UK’s National Health Service (NHS) and Ireland’s Health Service Executive (HSE).
Before joining Qure, Darren was the Country Manager in the UK and Ireland for Everlight Radiology Ltd, one of the fastest-growing global teleradiology companies in the market. He also held roles at Ecolab and Pfizer.
Disclaimers:
The opinions expressed in this feature are those of the interviewee and do not necessarily reflect the views of Future Medicine AI Hub or Future Science Group.