Building 3D Cellular Communication Networks with AI
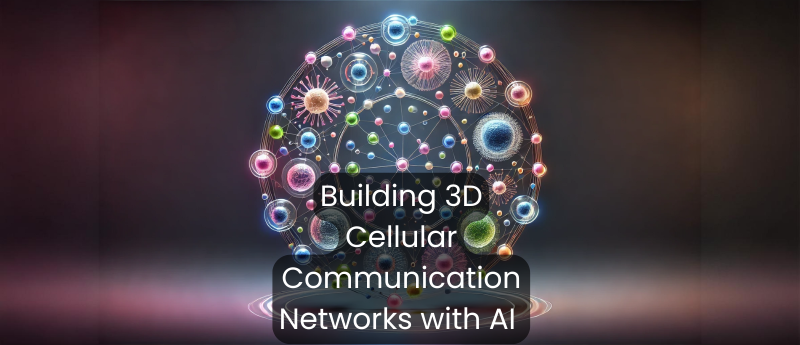
Researchers at the National Natural Science Foundation of China (Beijing, China) have developed an AI tool, called VGAE-CCI, that identifies cell-to-cell interactions (CCIs), and maps them into three-dimensional cellular communication networks. The tool showed remarkable accuracy even when challenged with incomplete or partially incomplete data.
Previous computational methods for studying CCIs (how individual cells communicate with each other) have been limited to analyzing interactions between cells within the same layer, overlooking cross-layer interactions.
These methods are further limited by systematic biases in training datasets and gaps in available information. This new AI model, VGCAE-CCI aims to overcome these challenges, offering researchers a way to better understand complex disease mechanisms that disrupt these CCIs.
Understanding Disease Mechanisms Through Cell-to-Cell Interactions
Cellular communication is vital to maintaining the body’s coordinated functions. It is primarily mediated by ligands secreted by one cell that bind to specific receptors on the surface of other cells.
Disruptions in these signalling pathways are hallmarks of many diseases. For example, in type 2 diabetes, the receptors that respond to insulin (ligand) are either downregulated in number or dysfunctional, thereby causing insulin resistance and hyperglycemia.
Similarly, cancerous cells reprogram their metabolic and intracellular signalling pathways to promote rapid growth and stay ‘hidden’ from immune system cells.
Exploring the different components of cellular signalling and their role in biological systems is a key focus of research. Selectively targeting these components can help identify druggable targets.
How Do Scientists Map Cell-to-Cell Interactions?
Scientists currently rely on two different experimental techniques to study CCIs:
- Single-cell RNA sequencing (scRNA-seq): Captures gene expression levels of individual cells. Using this comprehensive genetic information, scientists can identify genes associated with specific signalling pathways, by identifying genes that code for specific ligands or receptors.
- Spatial transcriptomics (ST): Measures gene activity within tissues while preserving the spatial context of gene expression. Unlike scRNA-seq, which analyzes individual cells, ST examines groups of cells together. Thus, providing a broader perspective, but at a lower resolution than scRNA-seq.
By combining these two techniques, researchers can achieve detailed, high-resolution maps of cellular communication, whilst preserving their nascent environments. However, these methods have limitations:
- Incomplete databases of receptor-ligand pairings hinder accuracy.
- ST-sequencing is prone to systematic biases from data preparation or interpretation which can lead to errors. For example, scientists or algorithms designed for data analysis might infer that receptors and ligands that appear close in the dataset are natural biological pairs; in reality, proximity does not necessarily mean that the two interact.
- Layered cell interactions are often overlooked because tissue samples must be thinly sliced for analysis using spatial transcriptomics. As a result, interactions between layers of cells are often neglected, meaning that the mappings of cellular communication networks are incomplete.
A study recently published in Briefings in Bioinformatics addresses these issues.
VGAE-CCI: A Deep Learning Framework for Predicting CCI
Developed under the guidance of senior author, Tianjio Zhang, Variational Graph Autoencoder – Cell-to-Cell-Interaction (VGAE-CCI) predicts CCIs with remarkable accuracy, even from incomplete datasets. VGAE-CCI is a deep learning framework based on a variational graph autoencoder construction, which was designed to overcome the challenges associated with using ST and scRNA sequencing.
VGAE-CCI can uncover features of cellular communication that are ‘hidden’ in datasets–complex or subtle aspects of CCI networks that are difficult to pick out without machine learning tools. Moreover, the model can identify these interactions even from incomplete datasets.
Delving into the Science
So, what is a variational graph auto-encoder (VGAE), and how was it adapted for this task?
VGAE is a type of neural network designed to learn patterns in graph-structured data, where relationships between elements, such as cells in a biological network, are represented. It integrates two established AI frameworks: variational autoencoders, and graph convolutional networks (GCNs).
Variational autoencoders encode data into a simpler, smaller representation, called a latent space. This latent space captures the most important features of the data while discarding any unnecessary details. By simplifying data in this way, VAEs can identify patterns more effectively and even generate new variations of the data.
GCNs, on the other hand, excel at recognizing patterns and relationships from complex, structured data such as graphs. In terms of CCIs, GCNs can be used to recognize spatial or functional connections between cells. These networks are commonly applied to analyze other forms of structured data, such as molecular graphs, three-dimensional spatial networks or images represented as graphs.
In VGAE-CCI, graphs are made up of ‘nodes, representing individual cells or their genetic profiles; and ‘edges’, which correspond to relationships between cells, such as spatial proximity or receptor-ligand pairings.
How VGAE-CCI Predicts CCIs from scRNA-seq and ST-seq Data
Crucially, the VGAE-CCI model can analyze CCIs in three dimensions by aligning both spatial-transcriptomic and single-cell RNA seq. This alignment is achieved by the following steps:
- Encoding: The VAE compresses the input data to identify key features from the cellular networks.
- Alignment: VGAE-CCI aligns both ST-seq and scRNA-seq data using the Probabilistic Alignment of Spatial Transcriptomics Experiments (PASTE) method, which integrates gene expression profiles with spatial coordinates.
- Decoding: the model decodes outputs –converting the compressed data back into a higher dimensional space, to produce a detailed representation of the 3D cellular-communication network.
Transforming Research with AI
The VGAE-CCI model was trained rigorously, and once this was complete, the researchers also tested VGAE-CCI’s ability to identify cellular interactions in different contexts.
For instance, they evaluated the model’s ability to identify CCIs under challenging conditions, including with missing data points, in which specific data points had been purposefully removed; or when artificial noise had been introduced into the dataset.
Researchers found that VGAE-CCI’s performance was impressive: even when half of the cellular interactions were missing from the dataset, the AI was able to predict edges with 75–80% accuracy, measured by AUROC, AP, and ACC scores. Moreover, VGAE-CCI also demonstrated remarkable robustness when challenged with particularly noisy data.
The final piece of the puzzle was evaluating the model’s ability to extract 3D interactions, interpreted using accuracy ratings before and after alignment of ST and sc-RNA seq data.
The research team observed a notable improvement in the model’s performance after alignment, with the average ACC increasing from 83% to 88%. This highlights the important role of interlayer connections in shaping CCI networks – more importantly, this suggests that investigating these interactions could unveil new therapeutic targets.
The results of this study illustrate the potential of AI to explore CCIs in more depth in both healthy and disease states. Tools like VGAE-CCI can accurately identify three-dimensional cellular interactions, even when faced with incomplete or noisy data.
Utilizing AI systems such as this could help scientists build more comprehensive and precise maps of cellular communication networks, potentially advancing our understanding of the biological mechanisms of various diseases.