Building a predictive model to assist in the diagnosis of cervical cancer
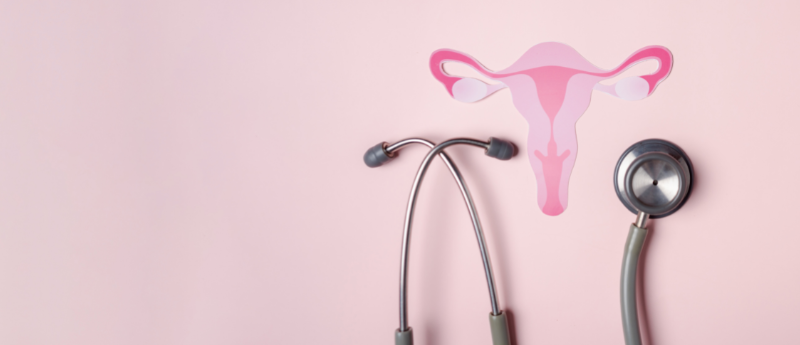
Future Oncology, our partnered journal, has recently published a Research article exploring the accuracy of a predictive model algorithm in estimating cervical cancer risk. Using multiple machine learning models, this algorithm was able to combine predictive probabilities for cervical cancer.
Abstract
Aim: Cervical cancer is still one of the most common gynecologic cancers in the world. Since cervical cancer is a potentially preventive cancer, earlier detection is the most effective technique for decreasing the worldwide incidence of the illness. Materials and methods: This research presents a novel ensemble technique for predicting cervical cancer risk. Specifically, the authors introduce a voting classifier that aggregates prediction probabilities from multiple machine-learning models: logistic regression, K-nearest neighbor, decision tree, XGBoost and multilayer perceptron. Results: The average accuracy, precision, recall and f1-score of the voting classifier were 96.6, 97.4, 95.9 and 96.6, respectively. Furthermore, the voting algorithm gains average high values for all evaluation metrics (accuracy, precision, recall and f1-score). The f1-score of the algorithm is 96%, which demonstrates the robustness of the model. Conclusion: The findings suggest that the probability of having cervical cancer can be accurately predicted utilizing the voting technique.