Causaly Announces New Agentic AI for Drug Discovery
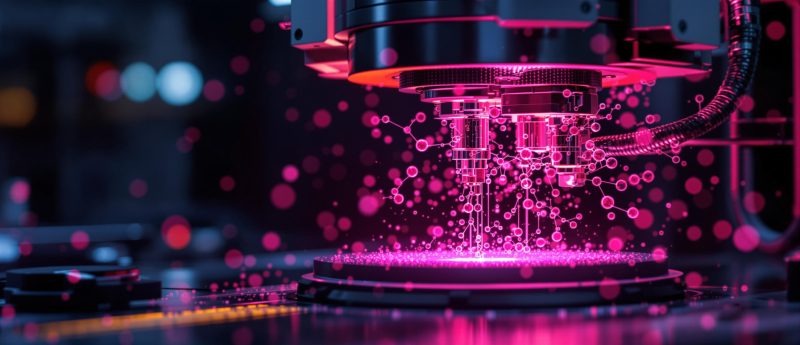
Causaly has released a new version of Discover—a platform that helps scientists easily understand complex biomedical data. The company believes this could massively aid drug researchers, accelerating the time taken to deliver treatments from bench to bedside.
Pharmaceutical companies spend an average of $2.3 billion to develop a single drug. A closer look at this steep price reveals a major culprit: the years spent sifting through data to prove that a medication is both safe and effective—an essential component of any new drug’s success.
Such an arduous process significantly slows down drug development pipelines—end-to-end, getting a new treatment into clinics takes around 10–15 years. For “newer areas of medicine,” like gene therapy, this timeline can stretch up to 30 years.
End-to-End Autonomous Research with Agentic AI
On 31 March, Causaly announced the latest evolution of Discover—a cutting-edge application designed to address this exact problem.
At its core, Discover is an agentic AI—a concept popularized in 2024 as large language models (LLMs) like Google’s Gemini 2.0 and OpenAI’s o3 evolved from simple text generation to more difficult tasks, like planning and reasoning. These AI agents operate autonomously, meaning they can complete tasks on their own initiative without the need for human intervention.
Think of it in the context of an office: Traditionally, LLMs answer questions when asked, providing assistance with administrative tasks like responding to emails. However, an Agentic AI model goes a step further—it can reschedule meetings, book appointments, and follow up with clients—all on its own.
Discover: Bringing Biomedical Knowledge Together, Faster
Discover follows these same principles but translates them into a biomedical language—analyzing and interpreting large datasets without any human input.
It harnesses both internal and external data sources (including the Human Protein Atlas and online web searches), generating comprehensive reports and answering complex research questions that could otherwise take years to answer.
Discover also utilizes information from Causaly’s Bio Graph, a vast biomedical database connecting 500 million facts and 70 million data relationships, which the team claims is the largest of its kind.
Through these initiatives, the company hopes that Discover can accelerate research behind potential new treatments, connecting patients with better treatments faster.
Co-founder and CEO, Yiannis Kiachopoulos, states:
“Causaly Discover serves as the central hub for all information researchers need to accelerate therapies to market. New AI agents now deliver deeper insights so scientists can quickly move from questions to confident decisions with unmatched speed and transparency.”
The team has reported that all information provided by Discover will come exclusively from trusted, verifiable sources, and that follow-up information can be easily requested through Causaly Copilot, their generative AI chat assistant. Scientists can even use Discover to automatically provide alerts and summaries of any new research in their chosen subject area.
Zooming Out: The Bigger Picture
AI in drug discovery is becoming an increasingly crowded market, with one source reporting 463 startup companies already in the space.
So, how does Discover fit into the conversation?
Spotlighting one giant of the industry, Google DeepMind has recently launched its family of machine learning models, “TxGemma,” to help developers build tools for specific drug discovery and development needs.
Similar to an AI “pick-n-mix,” companies can choose a basic model best suited for them before fine-tuning it with their own data—this way, they don’t have to build their own model from scratch, cutting down on both time and money.
Because of this, TxGemma can flex to meet different requirements across the pipeline—for example, to predict how safe and effective a drug is or how well the body absorbs it. But perhaps more importantly —and especially relevant here— Google DeepMind outlines the potential to integrate into agentic systems, tackling “more complex research questions” through multi-step reasoning and a specialized molecular and gene/protein toolkit.
Since agentic AI remains largely unexplored in drug discovery, DeepMind has also released a “Colab notebook” to track TxGemma’s usage, lifting the veil on how agentic AI could shape the field.
Key Takeaway
With the buzz around agentic systems at its peak last year, it is interesting to see this concept now colliding with the drug discovery world.
As technologies become more advanced, the industry could see a shift toward a faster, cleaner pipeline, moving beyond simply using AI to answer questions like “How effective is this compound?” and into an era of autonomous agents—supporting the research, design, and testing of new treatments with minimal human oversight.