‘Chemical Chat-GPT’ Paves the way for Rational Dual-Target Drug Design
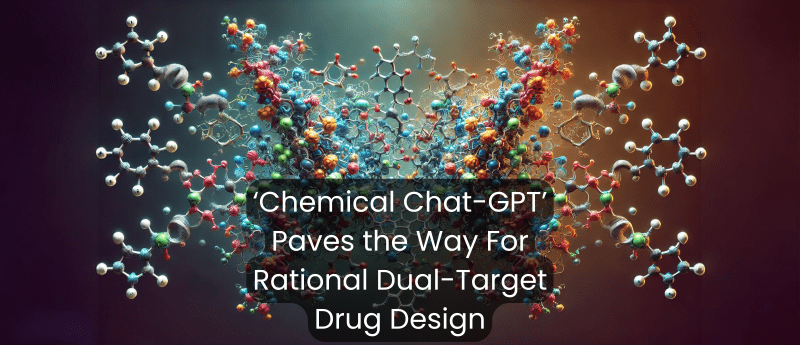
Researchers at the University of Bonn (Germany) have developed an AI tool capable of predicting compounds with multiple targets in biological systems. Traditional multi-target drug discovery is a complicated process with limited scope for targeting distinct biological structures and processes. However, AI has the potential to predict novel compounds with diverse mechanisms of action. From this, researchers might be able to design more powerful therapies for a broad range of diseases that have historically been difficult to treat.
Polypharmacology: Designing Drugs With Wider Biological Impact
Diseases typically manifest when multiple biological systems collapse.
Therefore, it is understandable how drugs with only one target often produce disappointing treatment outcomes for patients.
To address this, scientists are turning to an area of growing research called “polypharmacology” – a concept that hinges on the development of singular compounds with multiple targets.
While the prospect is exciting, designing mutli-target (MT) drugs is a challenge, involving lengthy and inefficient screening processes.
To help MT drug development, scientists are increasingly interested in AI to screen databases for compounds likely to have MT capabilities – which so far has mostly centered around machine learning (ML) techniques.
Machine Learning Can be Useful, But Has its Caveats
More interestingly, ML could open up opportunities to discover compounds with structurally and functionally distinct targets.
For example, while a compound might easily target families of proteins with similar structures implicated in similar processes, in some instances it would be useful to target structures with completely different functions – e.g., a protein that carries out metabolic reactions and one involved in DNA replication.
However, ML has its own limitations in this field. While useful for developing compounds with 2 targets, machine learning falls short in predicting compounds with more than 2 targets, i.e., triple targeting drugs, since these models would require significantly more data than is generally available.
Furthermore, although they are useful for identifying potential candidates, these models only screen for molecules; AI tools that can actually generate new MT compounds are few and far between.
These restrictions hinder progress in the polypharmacology field, calling for more complex AI models that can design completely new compounds with multiple, diverse biological targets.
Digging Deeper with Chemical Language Models
A pivotal study published in Cell Reports Physical Science sets the scene for such an undertaking. Sanjana Srinivasan and Jürgen Bajorath developed a type of large language model designed to understand chemical structures.
Specifically, they worked on developing a transformer-based chemical language model (CLM), that follows a series of steps:
1. SMILES pre-processing. Single-target chemical compounds are converted into a text format known as SMILES (Simplified Molecular Input Line Entry System) notation. Each molecule is converted into a string of characters (letters or symbols.)
2. Encoder-Decoder architecture. This is the core of the system: a transformer with an encoder-decoder architecture, which is commonly used in natural language processing tasks. The encoder first ‘reads’ the SMILE sequence using several layers:
a. Self-attention layers. This feature lets the machine understand how characters (i.e., chemical groups or atoms) relate to each other, regardless of their distance from each other. For example, the model can identify connections between two characters (atoms) that are far apart in the sequence but are structurally linked in the molecule.
b. Feed forward layers. After initial processing through the self-attention layers, the information about atoms or groups is refined through feed forward layers. The data is transformed (using two linear transformations and a non-linear activation function) so that the model captures more complex relationships. The feed forward layers focus on each individual atom’s specific characteristics – for example, which ‘group’ (e.g., an aromatic ring or a methyl group) a carbon in the sequence is associated with.
Another important feature of the encoder is positional encoding, which helps to maintain the position of tokens in the sequence as data moves through the layers.
Once the model has processed structural and functional information from single-targeting compounds, the decoder then generates a new SMILES sequence of a DT compound.
3. In the final stage, the newly-generated SMILES sequence is translated back into the chemical structure of the potential compound.
In the pre-training phase, for any given biological target pair, single-target (ST) compounds were mapped to dual-targeting (DT) compounds to recognize patterns between their structures and properties. The models were then fine-tuned to understand relationships between ST and DT compounds for structurally and functionally distinct biological targets.
Using this information, their AI model could generate new compounds with DT capability.
The team tested three pre-trained models, each with a different similarity threshold (0%, 25%, and 50%) to gauge how structural similarity between single-target and dual-target compounds affected performance.
The model with a 0% similarity threshold struggled to generate new compounds due to the lack of shared structural patterns to learn from. However, when the similarity threshold was increased to 25% and 50%, the model was able to reproduce a greater number of test dual-target compounds — specifically, compounds with known dual-target activity that were excluded from the training set.
When challenged to reproduce DT compounds from a known database, the 25% and 50% pre-trained models achieved high reproducibility, ranging from 23.3%–63.3%. Additionally, new compounds generated by the models showed up to 98.4% uniqueness and were considered highly accessible for synthesis, meaning chemists could feasibly produce them.
Looking Ahead
Such a model could be deployed for easy prediction and generation of new compounds with dual-targeting capabilities. This could open up the field of polypharmacology, speeding up development of MT compounds that are traditionally more inaccessible than ST drugs.
However, the ideal would be to explore how AI could help scientists to produce drugs with more than two biological targets, which is currently limited by little data on pre-existing compounds.
While this study does not stretch beyond DT compounds, it is nonetheless exciting to see the potential of CLMs in this field, facilitating generation of chemically-feasible compounds that are active against more than one biological target.