Deep learning model could accurately predict risk of endometrial cancer recurrence
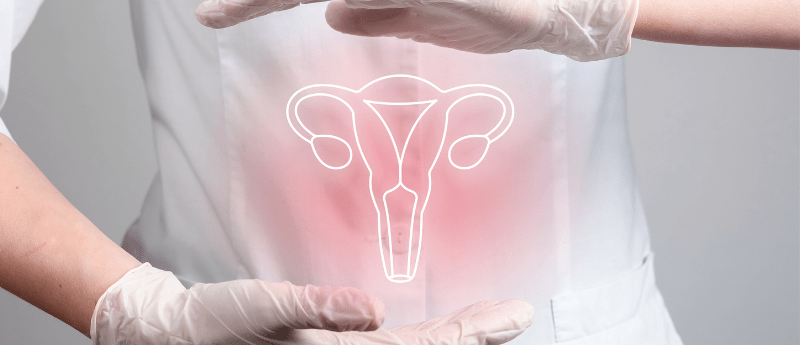
Researchers have created a deep learning model that could accurately predict the risk of endometrial cancer distant recurrence by analyzing a single histopathological tumor image.
Endometrial cancer is the most common type of cancer affecting the uterus; approximately 3% of women and people AFAB will suffer from uterine cancer at some point in their lives. Although endometrial cancer is extremely curable if detected in early-stages, with a 96% 5-year survival rate, patients who develop recurrent disease have a relatively poor prognosis with a median survival time of around 12 months.
The risk of recurrence is influenced by a variety of factors, including age, exercise and disease stage. Patients with low-risk endometrial cancer can usually be treated with surgery alone, whereas adjuvant therapy is recommended to patients with a high to intermediate risk of recurrence. Accurate differentiation between patients at high and low risk of endometrial cancer recurrence is therefore of paramount importance to establish the correct personalized treatments that are optimized for the patient and avoid unnecessary treatment-related toxicity.
At present, risk is assessed based on tumor characteristics and molecular profile, utilizing techniques such as histopathology or molecular testing of tissue samples. However, these methods have several disadvantages, including the significant variability between pathologists when interpreting histopathologic slides and the high costs associated with molecular testing.
Now, a joint effort by the AIRMEC Consortium has endeavored to surpass these limitations, through designing a deep learning model with the ability to accurately predict distant recurrence risk in patients with endometrial cancer utilizing a single histopathological slide. The collaboration included the TransPORTEC Consortium, the Department of Pathology at Leiden University Medical Center (Netherlands) and the Department of Pathology and Molecular Pathology at the University of Zurich (Switzerland).
“Deep learning is a powerful computer-aided predictive technology that has entered the field of pathology because it can be trained to read complex visual information from tumor slides after digitization,” explained Sarah Fremond, a PhD candidate in computational pathology and deep learning for endometrial cancer at Leiden University.
The team built the model utilizing long-term follow-up data from 1,761 patients with endometrial cancer, enlisted in the PORTEC-1/-2/-3 randomized clinical trials and three additional clinical cohorts. None of the patients had been treated with adjuvant chemotherapy before the study took place.
The study results were presented at the AACR (American Association for Cancer Research) Annual Meeting 2023 (April 14−19 2023, FL, USA). The model was trained to recognize predictive recurrence features with a dataset of 1,408 patients, in which a single digitized histopathological tumor slide image was obtained from each patient. The model’s performance was assessed on a new dataset from the remaining 353 patients whose data was not involved in training the model.
Of the 353 patients in total, the model classified 89 with a high risk of distant recurrence, 175 with an intermediate risk and 89 with a low risk. The model’s performance was highly accurate: 36% of patients that were classed as high-risk, 15.43% of patients classed as intermediate-risk and 3.37% of patients classed as low-risk developed an endometrial cancer distant recurrence, congruous with the model’s predictions. Interestingly, the model also outperformed features identified by pathologists that are usually utilized to categorize risk, for example tumor grade and molecular class.
There are, however, some important study limitations. For example, the results lack generalizability across different populations and sufficient validation on a wider scale. The results also do not take into account tissue scanning and slide preparation method disparities between different laboratories.
Nevertheless, this model represents a cost-effective tool to assist the prediction of endometrial cancer recurrence. “Although additional external validation is needed, the performance of this model serves as an important proof of concept that deep learning models have the potential to optimize clinical care for patients with endometrial cancer,” Fremond concluded.
The team plan to further improve the model’s accuracy and performance by incorporating risk-influencing variables into the model that are not identifiable on histopathological images.