DeepGlioma: the AI system developed to help diagnose brain tumors
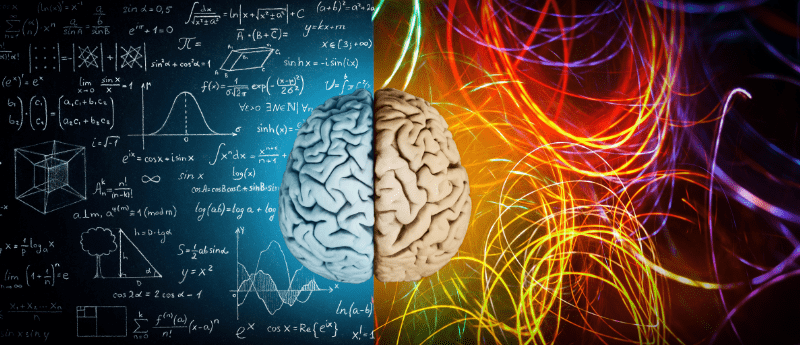
AI model DeepGlioma could classify brain tumor subtypes through identifying genetic mutations in real time.
AI can predict genetic mutations in cancerous brain tumors in less time than it takes the more influential of us to take a selfie (under 90 seconds to be exact), according to a recent study published in Nature Medicine. A group of engineers and neurosurgeons from Michigan Medicine (MI, USA) collaborated with researchers from several other universities, including New York University (NY, USA) and the University of California (CA, USA), to design an AI-powered screening system they state has the ability to diagnose brain tumors.
The most prevalent brain tumor type in adults is a glioma, originating from glial cells. According to the American Association of Neurological Surgeons, 78% of malignant brain tumors are gliomas. Glioma treatment is dependent on the type of glioma and different molecular subtypes can influence treatment risks and outcomes based on genetic disparities between patients. For example, patients with astrocytomas, a type of diffuse glioma, may increase their life expectancy to an average of 5 years when the tumor is fully removed, compared to other types of diffuse gliomas.
Recent advances in molecular classification have enhanced brain tumor management through increasing diagnosis accuracy and enabling treatment to be personalized. Despite this, the range of molecular diagnostic tests to classify diffuse gliomas are limited in access and obtaining results is time consuming, which delays potential treatments and surgery, as well as hindering research in clinical trials.
“Barriers to molecular diagnosis can result in suboptimal care for patients with brain tumors, complicating surgical decision-making and selection of chemoradiation regimens,” noted study lead author Todd Hollon, a neurosurgeon at University of Michigan Health. It therefore remains essential to create a faster way to identify different glioma subtypes utilizing molecular markers.
Now, an AI diagnostic system termed DeepGlioma could accelerate and simplify molecular diagnosis of diffuse gliomas in under 90 seconds. DeepGlioma diagnosed over 150 patients enrolled in the study by identifying mutations utilized for glioma subtype classification by the World Health Organization. The system attained a molecular classification accuracy of 93%.
DeepGlioma utilizes a combination of rapid optical imaging (a technique termed stimulated Raman histology) and deep-neural networks to analyze brain tumor tissue obtained during an operation and swiftly determine genetic mutations in real time. Before DeepGlioma, surgeons had no way to identify glioma subtypes during surgery, meaning this technology has the potential to expediate treatments and improve patient prognosis.
Patients with diffuse gliomas often have poor prognosis and few treatment options. One barrier to developing novel treatments for diffuse gliomas is the limited ability to test how drugs may affect brain tumors. Unfortunately, less than 10% of glioma patients are enlisted in clinical trials, which can restrict participation of certain molecular subgroups and hinder drug development research. With the creation of DeepGlioma, the researchers also aspire to encourage an increase in early clinical trial enrollment.
“Progress in the treatment of the most deadly brain tumors has been limited in the past decades- in part because it has been hard to identify the patients who would benefit most from targeted therapies,” declared senior author Daniel Orringer, an associate professor of neurosurgery and pathology at NYU Grossman School of Medicine. “Rapid methods for molecular classification hold great promise for rethinking clinical trial design and bringing new therapies to patients.”