Diagnosing schizophrenia through speech analysis
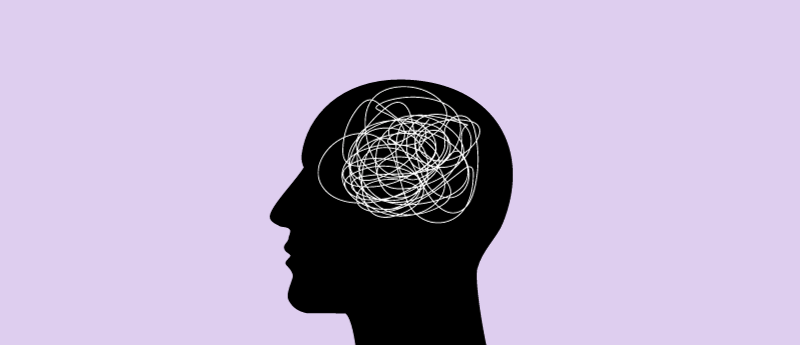
Through recognizing elusive speech patterns in patients with schizophrenia using AI language models, scientists have created a potential novel means of diagnosis.
Published in PNAS, the study provides important insights into understanding how speech patterns might influence the creation of ‘cognitive maps’: visual mental representations of memories and concepts.
The team, from the UCL Institute of Neurology (London, UK), developed natural language processing (NLP) tools for the automated analysis of speech, which they were able to characterize in patients diagnosed with schizophrenia.
Presently, mental health conditions such as schizophrenia are diagnosed with a subjective assessment by a mental health professional, during which a patient is asked questions about their mood, thoughts and behaviors. Medical tests such as blood tests and brain imaging are rarely used, and as such, we lack a thorough understanding of the influencing biological factors. Misdiagnosis is common and often exacerbated by frequent symptom overlap, which occurs across many mental illnesses.
A total of 26 participants diagnosed with schizophrenia and 26 control participants completed two verbal fluency tasks, where they listed as many words as they could belonging to the category “animals” (category fluency) or starting with the letter “p” (letter fluency) in 5 minutes.
Using an AI NLP model that they trained on a large dataset of text depicting the meaning of words, the researchers were able to analyze the participants’ answers. They discovered that the influence of semantic similarity on behavior was reduced in participants with schizophrenia when compared to control participants, meaning that the AI model was better able to predict the word choice and answers of the control participants. Participants with the most severe forms of schizophrenia had the least predictable responses.
The researchers explain that these observations are likely due to how the brain forms relationships between ideas and memories, and how it stores this knowledge in structured conceptual representations (cognitive maps).
This theory was supported by magnetoencephalography scans, which the researchers used to analyze brain activity in the participants. The brain activity in areas storing these cognitive maps was found to correlate with the semantic similarity of the participants’ answers (predictability), as well as negative psychotic symptoms. Overall, the study suggests that some symptoms of schizophrenia may indicate a dysregulation of neurocognitive processes associated with forming cognitive maps.
The team aims to test this technology on a wider scale, in more patients and with more speech variation, in the hopes that it could one day be applied in clinics. By enabling doctors and scientists to understand how the automated analysis of language could help diagnose and assess psychiatric conditions, the technology could help the 24 million people suffering from schizophrenia globally.
Matthew Nour, study lead author, commented: “We are entering a very exciting time in neuroscience and mental health research. By combining state-of-the-art AI language models and brain scanning technology, we are beginning to uncover how meaning is constructed in the brain, and how this might go awry in psychiatric disorders. There is enormous interest in using AI language models in medicine. If these tools prove safe and robust, I expect they will begin to be deployed in the clinic within the next decade.”