ESM3 | A New Frontier for Advanced Protein Design
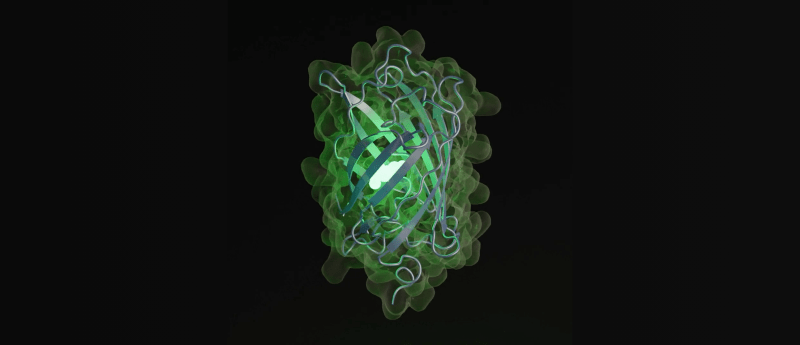
Featured image by Evolutionary Scale: render of a new green fluorescent protein generated by ESM3
Evolutionary Scale (from Meta’s Fundamental AI Research unit; NY, US) has unveiled a third generation generative AI model for advanced protein design. Called ESM3, it is a language model capable of fabricating entirely new proteins. In an astonishing demonstration of its capabilities, Evolutionary Scale used ESM3 to create a novel green fluorescent protein equivalent to 500 million years of evolution from its nearest naturally occurring ancestor.
Training
ESM3 is trained on an enormous dataset comprising billions of protein sequences from a wide range of environments, including diverse ecosystems and extreme conditions. Notably, the dataset includes both natural protein sequences and synthetic data.
The training process of ESM3 involves a technique known as masked language modeling. In this approach, parts of the protein sequences are hidden (masked), and the model is tasked with predicting these hidden parts. This forces ESM3 to learn the deep connections between sequence, structure, and function. By doing so, it can develop a comprehensive understanding of how proteins work.
ESM3 is a large-scale model with 98 billion parameters. Evolutionary Scale claim that it was trained using the largest amount of compute ever for a biological model, with over 1×10^24 FLOPS (floating-point operations per second).
How it Works
Proteins are essential molecules that perform a vast array of functions within living organisms. Each protein’s function is determined by its unique sequence of amino acids, its three-dimensional structure, and its biological role. ESM3 is capable of reasoning about these three attributes simultaneously, which is crucial for both understanding existing proteins and designing new ones.
A significant technical challenge in this endeavor is enabling the model to reason about sequence, structure, and function together. ESM3 addresses this by converting three-dimensional protein structures into discrete tokens, essentially transforming them into sequences of letters that the model can process similarly to how it processes natural language. This allows ESM3 to handle vast amounts of biological data effectively.
The model can generate new protein sequences by starting with a fully masked sequence and iteratively predicting the masked positions until the sequence is complete. Scientists can guide this generation process by specifying certain aspects of the desired sequence, structure, or function, allowing for the design of proteins tailored to specific applications.
ESM3 also improves over time using feedback mechanisms. The model can evaluate the quality of its own generations and make adjustments to enhance its performance. Laboratory experiments and existing experimental data provide additional feedback, refining and aligning the model’s outputs with real-world biological success.
Simulating Evolutionary Time
The most striking achievement of ESM3 is successfully generating a new green fluorescent protein, named esmGFP, which shares only 58% sequence identity with the nearest known fluorescent protein. Such distant natural proteins are typically separated by hundreds of millions of years of evolution, highlighting ESM3’s capability to explore new regions of protein space.
By generating protein sequences that diverge significantly from known sequences, ESM3 can mimic the timescales of natural evolution, exploring new areas of protein sequence space. This allows the model to create novel proteins, expanding the possibilities for scientific discovery and practical applications in medicine and beyond.
Open Source
Evolution Scale stated their commitment to open science in their press release, promising to share research and code for their models. This will start with the weights and code for a ESM 1.4B model, which are currently available on GitHub. While this is relatively minute compared the full 98B ESM3 model, it is a welcome contribution that will allow researchers to explore with greater customization.
Medium and large versions of ESM3 will be accessible through Evolution Scale’ API (currently in closed beta), as well as partner platforms AWS and NVIDIA AI Microservices.
Alpha Fold vs ESM3
While AlphaFold and ESM share the common goal of advancing our understanding of proteins, they do so in different ways. AlphaFold excels in predicting accurate protein structures from sequences, making it invaluable for structural biology.
ESM, on the other hand, offers a broader scope by not only predicting structures but also generating new protein sequences and functions, simulating evolution, and providing a platform for programming biological systems.