FlyWire: How A Complete Model of a Fruit Fly’s Brain is Transforming Neuroscience
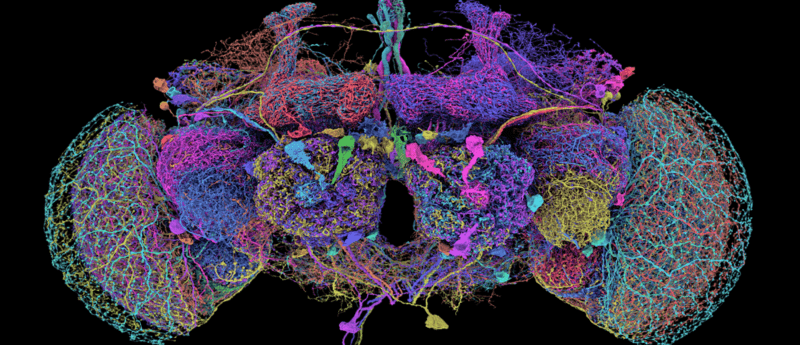
Image credit: FlyWire.ai
FlyWire is a groundbreaking neuroscience project that recently achieved the first complete reconstruction of a fruit fly’s brain. Drawing on the collective expertise of 127 institutions, AI, and the keen eyes of citizen scientists, the project surmounted formidable technical challenges to deliver a freely accessible, high-resolution map of every neuronal connection. Based on an in-depth webinar with Mala Murthy and Sebastian Seung, this deep dive reveals how the FlyWire initiative is transforming our understanding of neural circuitry and setting the stage for future breakthroughs in neuroscience.
Sebastien Seung has been a professor of computer science and neuroscience at Princeton University’s Neuroscience Institute since 2014, where he is head of the Seung labs. Before this, he worked at the Massachusetts Institute of Technology as a Professor of Computational Neuroscience and Physics.
Mala Murthy serves as the Director of the Princeton Neuroscience Institute and is the Karol and Marnie Marcin 1996 Professor of Neuroscience at Princeton University. Her work centers around how the brain extracts valuable information from the sensory world and utilizes information to modulate social behavior.
Why is The Brain So Difficult to Understand?
The brain is an exceptionally complicated organ, with huge networks of neurons carrying out highly specific functions. Mapping the intricate wiring of these neurons to build a connectome could reveal critical insights into the mechanisms behind certain behaviors, emotions, or sensory perceptions. Broadly speaking, this could help scientists identify important targets for neurological diseases such as Parkinson’s disease, dementia, and depression.
However, mapping the entire circuitry of a brain is no mean feat, and scientists have long sought more advanced experimental techniques that could help achieve this goal.
One promising tool for connectome studies is electron microscopy (EM.)
EM uses beams of electrons to illuminate biological specimens at nanoscale resolution, allowing researchers to observe cells or organelles with far greater detail than traditional light microscopy.
As such, this has been and continues to be invaluable for exploring the brain’s connectivity.
However, while EM offers unmatched resolution, there is a caveat: the field of view is often too small to capture the brain’s entirety. But, increasing the field of view to visualize larger areas reduces image resolution, limiting its application for connectome constructions.
Sebastien Seung illustrates this issue: “The difficulty is that neurons are both small and big at the same time. A thin branch of a neuron might be 100 nanometers or less in diameter,” he says, “and yet, a neuron must be long enough to extend from a giraffe’s brain to its spinal cord.”
It is worth pointing out that in the 1980s, EM successfully visualized the entire connectome of a nematode (Caenorhabditis elegans), but their brains only contained 300 neurons. This small scale meant it was feasible to map its neural connectivity completely.
Electron Microscopy Imaging for Bigger Brains
Trying to capture the neural connectivity of larger organisms is, however, a different story.
Even fruit flies have more complex brains – in fact, this relatively simple organism has almost 470 times the number of neurons that nematodes have.
To capture this substantially bigger neural network, scientists would have to use a much wider field of view that would sacrifice the resolution of the image. It then becomes difficult to identify neural structures that are important for connectivity, like synapses.
This trade-off between resolution and field of view has limited EM’s application for more comprehensive neurological studies.
“We know that neurons and synaptic connections underlie neural activity, and ultimately the behaviors generated by the nervous system,” Mala Murthy explains, “But we don’t know how, because we haven’t had these connectomes with synaptic-level resolution.”
That is, until the pair co-founded FlyWire: a research project dedicated to reconstructing the entire neural network of the brain of an adult female Drosophila melanogaster, i.e., a fruit fly.
The connectome captures the brain at incredibly high resolution, mapping 500,000 connections (chemical synapses) between 140,000 neurons.
To achieve this high resolution while covering such a large area, scientists scaled up a specific variant of EM (serial section transmission EM) to dramatically increase its imaging capabilities.
Briefly, this technique visualizes small sections of a specimen by transmitted electron beams; the more electrons that pass through the sample, the lighter that region appears in the image. Physically thicker or electron-dense areas, such as nuclei, absorb more electrons and so appear darker.
Images from individual sections are then aligned to form a complete 3D image of a larger area – i.e., the brain of a fruit fly.
Despite these optimization efforts, they encountered another issue: while EM imaging at this unprecedented scale could capture the entire connectome, they had obtained an exhaustive amount of data that required intensive analysis.
Sebastien emphasizes this problem: “If you have a 3D image that allows both zooming in and zooming out to incredible detail while seeing the whole picture, you have a lot of data; a cubic millimeter of brain would be over a petabyte of data: 1015 bytes.”
Harnessing the Power of AI to Recreate the Brain
With this daunting task ahead, AI was employed to analyze and annotate connectivity features; primarily, this involved distinguishing between postsynaptic and presynaptic neurons.
The team used a machine learning model called ‘3D U-Net’ – a type of convolutional neural network (CNN) adapted to analyze spatial information in three dimensions.
The U-Net component means that the model follows an encoder-decoder pattern: in the ‘encoder’ phase, images are compressed to extract their key features. They are then upscaled again in the ‘decoder’ phase to restore resolution.
3D U-Net represents neurons as connected components in a graph that is made up of nodes (also known as vertices) and edges
In this graph, each node corresponds to a supervoxel – a cluster of 3D pixels (voxels) representing a section of neural tissue. Edges, on the other hand, model relationships between these supervoxels; an edge connecting two nodes means that the supervoxels are physically linked, indicating that they are part of the same neural structure.
On this basis, AI models were trained to differentiate and classify synaptic connections, helping to label neurons in the EM images.
Nonetheless, Sebastien and Mala faced yet another challenge: AI is not always 100% accurate and will make mistakes that need to be manually corrected.
“Artificial intelligence doesn’t usually replace humans; it just augments them. In the end, we actually need human intelligence to come and fix the errors that the AI made,” says Sebastien.
In other computational contexts, errors might not significantly affect the AI’s overall performance. However, Mala highlights that, in this instance, scientists were working with only one dataset, i.e., brain images from one fly, to map neural networks. So, any errors made by the AI (e.g., incorrectly labeling a synapse) would limit the connectome’s accuracy.
Data Analysis: A Problem Shared is a Problem Halved
To tackle this issue, Mala and Sebastien ‘opened up the data’ (i.e., publicly released the dataset), enlisting the help of hundreds of scientists, including some of the leading drosophila experts in the scientific community.
“The logic was that if we opened up the data, it would drive science forward in unexpected ways, which we’ve seen,” says Mala.
She goes on to emphasize collaboration at the core of the proofreading process: “It really was the work of a huge team of people…People who had been working their whole careers and wanted a whole brain connectome jumped in and brought their full teams on board.”
It wasn’t just scientists, though, that worked on proofreading these images. Volunteers from the public (citizen scientists, in this project known as ‘flyers’) also chipped in. Sven Dorkenwald (Allen Institute, University of Washington) developed a platform called CAVE that turned
proofreading into an interactive game-like experience.
Users underwent tasks such as verifying neuron segments, merging fragmented neurons, and splitting incorrectly joined neurons, helping to correct the errors that were made by AI.
Finally, they enlisted the help of computers; specifically, a software called ChunkedGraph.
ChunkedGraph organized the annotated 3D images into a hierarchy of smaller, manageable supervoxels. These supervoxels gradually combine to form complete parts of the image, enabling researchers to focus on small, detailed regions without altering the integrity of the overall dataset.
Through the collaboration of advanced software, leading academics, and dedicated citizen scientists, the once-impossible task of creating a connectome became a reality. Mala and Sebastien had successfully developed FlyWire: an accurate and complete model of the fly’s brain.
What Lies on the Horizon for FlyWire?
FlyWire has promising applications in neuroscience research– for example, scientists can use the connectome to trace important neural circuits that mediate different behaviors, emotions, or sensory processing.
Excitingly, it is already proving extremely useful in the field; particularly for understanding neurobiological components of vision.
For example, a study published in Nature used the FlyWire connectome to explore how recurrent connections between neurons allow the fly to perceive different hues.
The use of the FlyWire drosophila connectome for research has not just been limited to vision though.
Another part of the brain that hadn’t previously been mapped is the sub-esophageal zone (SEZ), a structure containing neurons that connect the brain to motor neurons, as well as neurons that drive taste perception.
Two different studies have used the connectome to investigate these different components of the SEZ: One research group researched the underlying circuitry that lets flies carry out motor functions like walking and stopping, while the other group built a model to predict feeding behaviors in response to different tastes.
In this way, FlyWire is not just a powerful tool – it’s a game-changer in neuroscience. By enabling a holistic, whole-brain view rather than isolating sections of the brain, FlyWire allows researchers to uncover new information that was previously inaccessible, opening new frontiers for neurological research.
Sebastien reflects humorously on the transformative nature of this work: “In science fiction movies, you often hear about the idea of uploading your brain to a computer and living forever… Mind uploading is now a trope of science fiction, and sometimes I joke that one fly did die for this study, but this fly could live forever in simulation.”
He goes on to say: “It is a crazy thing where science fiction is now becoming sort of legitimate scientific discipline, just through a fly.”
Looking ahead, FlyWire holds immense potential. Moreover, as AI technology continues to evolve, it could unlock a wealth of possibilities for researchers, providing unprecedented insight into the complexities of the brain.