Gene editing at its prime: machine learning algorithm accurately predicts the success of prime editing
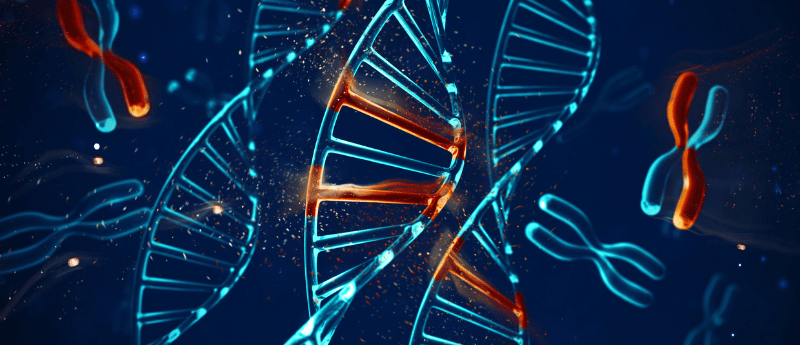
A recent study has created a way of predicting prime editing insertion success using a machine learning algorithm, promising to accelerate gene editing technologies.
A team of researchers at the Wellcome Sanger Institute (Hinxton, UK) have designed a novel machine learning algorithm that allows scientists to accurately predict the probability of successful prime editing. Using the algorithm to analyze the insertion efficiency of thousands of sequences, recurring key factors were identified that were associated with the successful insertion of an edited DNA sequence into the genome of a human cell.
A variation of CRISPR-Cas9 gene editing, prime editing involves a modified Cas endonuclease that creates nicks in a single strand of DNA instead of double-stranded breaks (Cas9 nickase). This Cas9 nickase is fused to an engineered reverse transcriptase and a prime editing guide RNA (pegRNA) that binds the target site and encodes the desired edited sequence.
As the CRISPR components are modified in prime editing, DNA sequences are edited without creating a double-stranded DNA break, as in traditional CRISPR. This is an advantage over traditional CRISPR as DNA is repaired using the cellular mismatch repair system rather than non-homologous end joining or homology-directed repair, reducing random unintended edits and off-target effects. Due to its increased precision, prime editing has enormous applications as a genome editing technique to treat genetic diseases such as cystic fibrosis and sickle cell anemia.
While gene editing tools have taken great strides in advancement over the years, they nonetheless have several limitations, including a poor understanding of the factors that influence editing success. Prime editing is no exception.
Now researchers have discovered a way to overcome this limitation, using artificial intelligence. After designing a library of 3,604 DNA sequences of assorted lengths, different prime-editor delivery systems were used to insert these sequences into the genomes of three human cell lines using different DNA repair mechanisms.
A week after insertion, the cell genomes were sequenced to determine which edits had been successfully inserted. This data was then used to train a machine learning algorithm to detect patterns in which factors were associated with insertion efficiency, such as the type of DNA repair mechanism or the sequence length.
Once trained, the algorithm’s performance was tested on a new set of data and was discovered to accurately predict the success rate of insertions.
In the future, the research team aims to understand if and how human genetic diseases can be treated or cured using prime editing by creating models for every known human genetic disease. This technology has demonstrated thus far that it can accurately predict insertion efficiency, meaning it has the potential to accelerate prime editing techniques and could help researchers determine the best edits to make for a particular genetic flaw.
Leopold Parts, study senior author, concluded: “The potential of prime editing to improve human health is vast, but first we need to understand the easiest, most efficient and safest ways to make these edits. It’s all about understanding the rules of the game, which the data and tool resulting from this study will help us to do.”