Google Enhances Fetal Health Prediction with AI
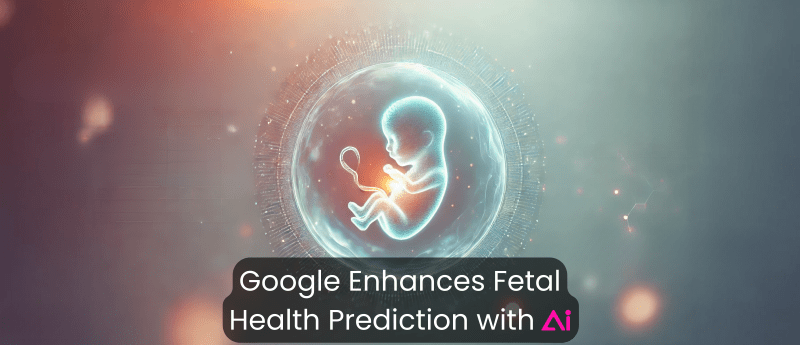
Tech and internet giant Google has announced the development and testing of a machine learning (ML) model designed to predict fetal health based on cardiotocography (CTG) data.
Researchers are investigating which factors may impact the effectiveness of current deep-learning models. Additionally, they have designed a prototype that could help clinicians assess CTG information to detect fetal hypoxia and potentially enhance health outcomes.
What Is Cardiotocography?
Cardiotocography is a very common non-invasive practice that tracks the fetal heart rate and uterine contractions throughout pregnancy. By analyzing CTG data, clinicians can detect potential health issues such as fetal discomfort or high blood pressure early.
However, despite CTG being regularly used in modern healthcare, the technique confronts significant limitations.
Fetal cardiac graphics and visual explanations are often difficult to decode, and reading accuracy varies depending on the qualities of specific examiners. This means that CTG interpretations can be subject to interpretative inaccuracies and a lack of objectivity.
Additionally, engaging with continuous CTG procedures may result in potential fetal misdiagnosis and delayed intervention, and even a rise in cesarean section and operative vaginal delivery.
How Can Google’s Initiative Make a Difference?
To address medical subjectivity in CTG interpretation, Google researchers have focused on deploying deep learning techniques to detect fetal hypoxia, a critical condition characterized by lack of oxygen during pregnancy.
The objective of the research was to provide clinicians with objective interpretation advice to reduce work pressure and potentially improve fetal outcomes.
To do this, Google relied on the deep neural dataset CTG-net to analyze fetal heart rate and uterine contraction data to predict fetal hypoxia.
To enhance efficiency, CTG-net presented new methodological configurations, including ‘architecture and hyperparameter optimization’, ‘single input variation’ and ‘addition of metadata’.
What Are Deep Neural Networks?
To understand how Deep Neural Networks (DNNs) function, it is important to recognize that a traditional neural network is designed with inspiration from the human brain. Typically, a neural network consists of three layers of artificial ‘neurons’: an input layer, a hidden layer and an output layer.
On the other hand, deep learning networks comprise numerous hidden layers and can be understood as an upgraded version of traditional neural networks. These extra layers enable the network to perform complex operations on large volumes of data, allowing it to learn intricated patterns and representations.
Consequently, deep learning models have become a cornerstone method in medical image analysis.
Research Methodology and Results
The construction of the CTG-net model relied on convolutional neural networks intended to assess fetal pulse and contraction of the uterus data and successfully understand their temporal correlations.
Moreover, to compare objective and subjective measures — umbilical artery blood pH and the Apgar score respectively—, researchers used the CTU-UHB Intrapartum Cardiotocography Database, which included 552 CTG recordings.
The results indicated that those models incorporating pH data — considered “ — outperformed others. This highlights deep learning models’ ability to minimize subjectivity in CTG interpretation and its potential to enhance fetal outcomes.
Near Future Challenges
Although AI has the potential to significantly advance various healthcare fields, such as cancer research, protein stability prediction and vaccine production among others, its application in fetal health prediction is still in development.
Although encouraging, Google’s study’s results are based on limited data from only one hospital in Prague, Czech Republic. Consequently, further research including a larger and more diverse dataset will be required to fully validate the proposed conclusions.
“We used de-identified open-source CTGs from 552 patients at a single hospital in Prague, Czech Republic. To enhance the robustness of our findings, future investigations should involve a larger and more diverse dataset sourced from maternity centers worldwide, encompassing varied clinical contexts, demographics, and outcomes. […] Further work is needed to understand how such prediction algorithms can be optimally integrated into clinical workflows to improve neonatal outcomes.”
Researchers involved in Google’s CTG-Based Fetal Health Prediction study.
Moreover, before AI applications in fetal health forecasting and modern healthcare can be fully trusted, additional investigation is necessary to address broader concerns related to data privacy, bias, and device clinical validation. Addressing these urgent issues will be essential to ensure that AI tools comply with ethical standards and regulations that safeguard patient interests.