Health on the menu: AI’s bite-by-bite analysis of UK eateries
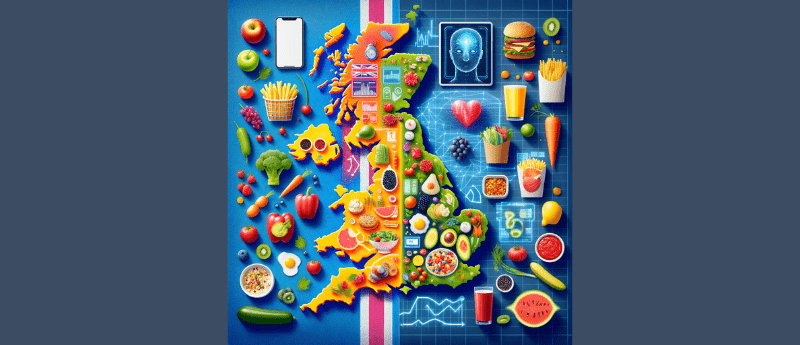
In a first of its kind study, researchers at the University of Cambridge (UK) used AI to assess the healthiness of every single out-of-home food outlet in the UK.
Looking at over 170,000 pubs, cafes, restaurants and takeaway outlets, they found a two-pronged burden in deprived areas with more outlets and less healthy options. Their research offers new perspectives of socioeconomic factors impacting population health with implications for policy decision making and food health research.
To achieve this, the team scraped data from the Just Eat web platform for locations and menus of food outlets. They then applied a deep learning model to rate each outlet from 0–12 (12 being the healthiest) based on 15 menu metrics. These metrics, which ranged from the diversity of vegetables to the selection of desserts, were identified in previous research as the best predictors of the opinions of experts analyzing menus.
With a subset of the UK’s outlets listed on Just Eat, names and hygiene ratings were the only data available for all outlets in the UK. Yuru Huang (a senior author of the study) and colleagues therefore trained another deep learning model to predict the healthiness of all food outlets based on their Just Eat dataset.
They found the average predicted menu healthiness was highest for restaurants, followed by cafes; snack bars and tea rooms; pubs, bars and inns; and fast food and takeaways.
By incorporating location and socioeconomic data, they also explored the distribution of predicted menu healthiness by local authorities (England only). The City of London, Kensington and Chelsea, and Westminster had the highest menu healthiness scores. Meanwhile Northeast Lincolnshire, Luton, and Kinston upon Hill were found to have the lowest scores, with more takeaway and fast food outlets.
The authors point out that precision was not the objective of this study, rather that it is a proof of concept that food health research can be automated at scale using publicly available data. Indeed, it provides a foundation for future work to explore population-scale impacts of menu options on dietary behavior. There are also implications for introducing policies to tackle socioeconomic disparities identified by similar research.
Of course, at this stage the use of outlet names alone is a significant limiting factor for reliably predicting the healthiness of their menus. There also remains many options for additional data integration such as actual buying habits of consumers, which in turn would support the predictive capability of AI models. This could refine dietary behavior insights, aiding in the development of new policies to reduce health disparities in deprived areas. The result of doing so could have positive implications for combating diet-related diseases in such areas, as well as for the wider population.