How AI is Tackling the Global Antibiotic Crisis
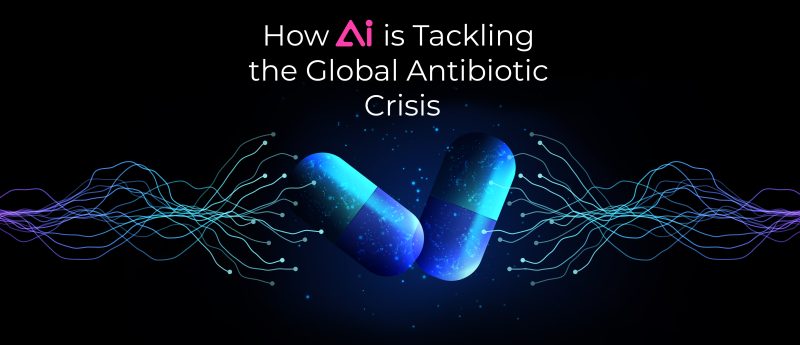
Researchers have developed an AI method to accurately predict ideal drug candidates for a new class of antibiotics known as host defence peptide (HDP)-mimicking polymers, which are typically difficult to engineer. This study sets the scene for accelerating drug development at a pivotal point in the antibiotic race.
Antimicrobial resistance (AMR) is often overlooked as a problem for human health. Yet, by 2050, it is estimated that bacterial infections could become the leading cause of mortality worldwide.
Despite this shocking prediction, a 2015 survey conducted by World Health Organization revealed that the majority of people (57%) believe they are immune to AMR, as long as they follow their doctor’s guidelines when taking a course of antibiotics.
In reality, resistance is an inevitable outcome of antibiotic use. While sticking to the right antibiotic regimen is important for slowing down the development of AMR, it is vital that drug development strategies evolve quickly to fight the war against resistance.
Current Challenges to Antibiotic Design
For the past 50 years, developing new antimicrobials has followed a well-established scheme: (1) identifying biological targets in bacterial cells that are essential for their survival, (2) screening for potential drug candidates (‘hits’) which bind the target and (3) testing and optimising the ‘lead’ compounds to make them as effective and safe as possible. However, this process is long and expensive.
To streamline this method, both in terms of cost and timeline, new therapeutics are often derived from the structures of pre-existing drugs. In order to target acquired resistance mechanisms, researchers will then slightly modify the chemical structure of the new compound, altering its properties.
This has been the case and point for many drug classes, such as the cephalosporins which were designed to fight against penicillin resistance.
However, bacteria evolve rapidly and in numerous ways. Natural selection gives rise to random mutations which change the target protein’s shape, i.e. antibiotics can no longer effectively bind their bacterial target.
More worryingly, antibiotic exposure can even directly accelerate how quickly bacteria mutate. Effectively, bacterial evolution is outcompeting the current rate of drug discovery.
Could AI be the Solution?
Nevertheless, there is hope. Published in the journal Nature Communications, researchers at the East China University of Science and Technology (China) have developed a few-shot AI methodology to aid with designing a type of molecule called host defence peptide (HDP)-mimicking polymers.
This class of polymers exhibit low susceptibility to antibiotic resistance while maintaining powerful antimicrobial potency.
Usually, these types of molecules are difficult to design with AI for two main reasons:
-
- There is a lack of available data relating to HDPs, therefore AI frameworks struggle to accurately identify relationships between chemical structures, biological properties and potency.
- Polymers are large molecules; predicting how one variable (for example, extent of branching) can change their biological effect is complicated by other variables – for example, chain length.
To overcome these challenges, the group focused on building a framework with multiple types of information (i.e., the polymers’ sequences and chemical bond information) to enrich the small dataset.
Their AI used a technique called reinforcement learning to explore different polymer designs, gradually improving its predictions with each iteration.
The range of possible polymer structures was then refined using molecular fragments, known as ‘graph grammar fragments’, which the AI could use to recombine subsets of molecules and produce new compounds. This process allowed the researchers to simulate over 100,000 possible polymers and identify 83 that showed the most promise for fighting drug-resistant bacteria.
From here, one of the predicted molecules was synthesized and tested in the lab. The results were impressive—this polymer was able to kill a wide range of drug-resistant bacteria, including strains that are resistant to common antibiotics.
Implications for the Future of Antibiotics
These researchers have proven that AI can be used to reliably predict promising drug candidates, even when AI training datasets are restricted.
This has significant ramifications for the field of antibiotic development, showing that AI can be used to tackle the constraints of conventional drug design and development by eliminating exhaustive screening and optimization methods.
Going forward, researchers may rely on this approach to optimise the pipeline delivery of HDP-mimicking peptides, and other antibiotic classes, from bench to bedside.
In a broader sense, the results of this study highlight that AI could robustly contribute to the race against antibiotic resistance.