How artificial intelligence could redefine clinical trials in cardiovascular medicine: lessons learned from oncology
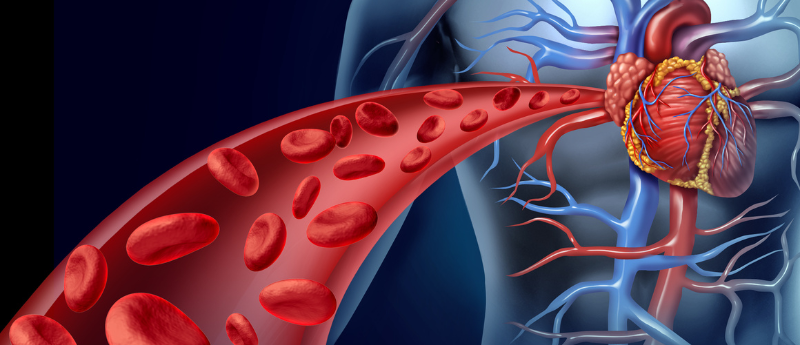
Personalized Medicine, our partnered journal, has recently published a Commentary exploring the use of AI-assisted randomized clinical trials in cardiovascular medicine, for earlier result predictions and lower risk to patients.
Features & limitations in randomized controlled trials
The randomized controlled trial (RCT) is the gold standard for evidence in clinical research. RCTs allow an investigator to test a specific hypothesis by controlling for known confounders via regression analysis and ameliorating the effect of potential unknown confounders via randomization. Theoretically, RCTs provide unbiased estimates of a relative treatment effect when conducted with adequate number of subjects [1]. Ideally, each and every medical intervention would be subjected to rigorous and well-powered RCTs to demonstrate effectiveness before the interventions are implemented into clinical practice. However, practically, this ideal is unattainable for several reasons, the most important of which are the immense expense of planning and executing an RCT; difficulty recruiting patients to participate and challenges in generalizing findings to those that have been excluded from the RCT. In fact, today, over 30,000 cardiovascular disease (CVD) related clinical trials in clinicaltrials.gov have either not been completed or have not reported results in peer-reviewed publications because of limited power (i.e., difficulty in recruiting potential candidates), inadequate period of follow-up, heterogeneous study populations with unaccounted inter-individual variabilities, adverse events that were potentially unrelated to interventions or null publication bias. These deficiencies often lead to difficulties interpreting trial results or generalizing trial findings to individual patients at the point of care [2,3]. Furthermore, many RCTs deviate from the ideal analytic strategies because of design flaws. Importantly, clinical trials often enroll patients who may not be representative of the targeted population in the first place [4]. RCTs also cannot reveal the distribution of risk in the overall population, nor provide accurate measures of absolute effectiveness for a treatment when the treatment effects can differ between the clinical trial and the real world (e.g., medication adherence, observer effects, patient characteristics or drug interactions). For these reasons, there has been an ongoing search for better experimental approaches.
In this paper, we will discuss the potential for adaptive, artificial intelligence (AI) guided clinical trials to generate evidence in cardiovascular medicine. AI may allow an investigator to predict results sooner and potentially harming fewer patients, or to perform AI-assisted randomization strategies using a high-dimensional set of variables.