How can neural networks predict Parkinson’s disease onset years ahead of time?
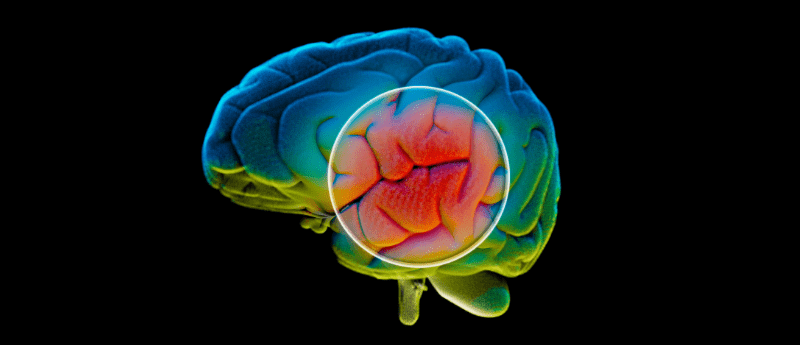
AI can now predict the risk of developing Parkinson’s disease years before symptoms first manifest based on the analysis of metabolites in an individual’s blood.
A collaboration of scientists from Boston University (MA, USA) and UNSW Sydney (Australia) have created an AI tool that can predict the onset of Parkinson’s disease many years before symptoms develop by identifying significant biomarkers. This AI tool could not only lead to the earlier diagnosis of Parkinson’s disease, but also represents an opportunity to advance the diagnostic accuracy for numerous other diseases and conditions based on metabolomics data as well as other omics technologies.
The study involved analyzing the blood samples of healthy participants who took part in The European Prospective Investigation into Cancer and Nutrition (EPIC) study, utilizing a machine learning neural network termed CRANK-MS (Classification and Ranking Analysis using Neural network generates Knowledge from Mass Spectrometry).
The team selected a sample of 39 patients from EPIC who went on to develop Parkinson’s disease up to 15 years after sampling and analyzed their metabolomic data with CRANK-MS. This data was then compared to metabolites from 39 healthy matched control patients that also took part in EPIC. From this, they were able to discern which distinctive metabolite combinations could be early indications of future Parkinson’s disease development.
“Typically, researchers using machine learning to examine correlations between metabolites and disease reduce the number of chemical features first, before they feed it into the algorithm,” stated Alexander Donald, an associate professor at UNSW who developed CRANK-MS. “But here we feed all the information into CRANK-MS without any data reduction right at the start. And from that, we can get the model prediction and identify which metabolites are driving the prediction the most, all in one step. It means that if there are metabolites which may potentially have been missed using conventional approaches, we can now pick those up.”
Parkinson’s disease is currently diagnosed by reviewing signs and symptoms, based on both physical and neurological assessments. Atypical symptoms such as sleep disorder and apathy can also manifest a long time before motor symptoms in some patients. CRANK-MS may now present an opportunity to predict the future risk of developing Parkinson’s disease when such atypical symptoms initially appear.
The analysis identified a total of five metabolites that were most strongly predictive of Parkinson’s disease: triterpenoids (downregulated in Parkinson’s disease patients compared to controls), cholestane steroids (downregulated), diacylglycerol (downregulated), vitamin D (upregulated) and polyfluoroalkyl substance (PFAS) (upregulated). Interestingly, previous research has also associated these metabolites with the disease.
Triterpenoids are neuroprotectants with antiviral, antimicrobial and anti-inflammatory properties. Several foods, such as beans, olives and apples are sources of triterpenoids, so it is intriguing that this metabolite was lower in concentration in the blood of those who later developed Parkinson’s disease compared to healthy controls. Additionally, higher levels of PFAS in Parkinson’s disease patients compared to controls could indicate greater exposure to industrial chemicals. Subsequent research could explore any naturally protective properties that these foods may possess against developing Parkinson’s disease.
The researchers highlight the need for further research with much larger and more diverse participant cohorts, to increase the validity and reliability of the CRANK-MS results. Nevertheless, although the study sample size was fairly small, the results are extremely promising: utilizing metabolomic analysis, CRANK-MS predicted the onset of Parkinson’s disease with up to 96% accuracy.
“We’ve built the model in such a way that it’s fit for purpose,” concluded Diana Zhang, a UNSW researcher who was also involved in developing CRANK-MS. “The application of CRANK-MS to detect Parkinson’s disease is just one example of how AI can improve the way we diagnose and monitor diseases. What’s exciting is that CRANK-MS can be readily applied to other diseases to identify new biomarkers of interest. The tool is user-friendly where on average, results can be generated in less than 10 minutes on a conventional laptop.”