Improving de novo protein-binder design with deep learning
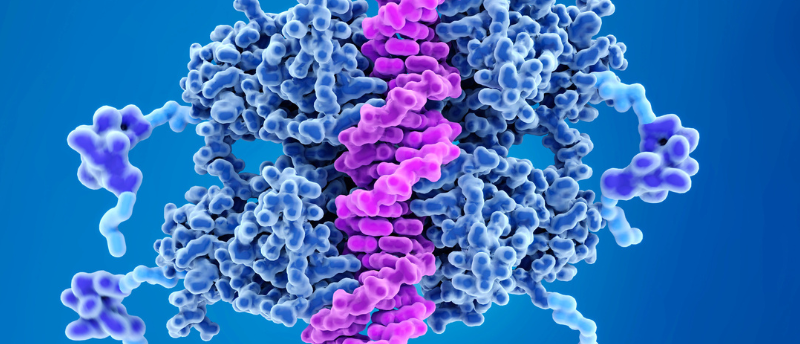
Using deep learning to enhance physical models for protein-binder design increases the target binding success rate.
Researchers at the University of Washington (WA, USA) and Ghent University (Belgium) combined physically based methods with AlphaFold2 or RoseTTaFold to improve de novo protein-binder design. They report a nearly 10-fold improvement in binding success compared to the original energy-based method.
Knowing a protein’s chemical structure and using this to identify or design proteins that can bind to them is key to generating therapeutic candidates and diagnostics. However, “the search space for proteins is enormous,” commented co-author Brian Coventry (University of Washington). For example, a protein made of 65 amino acids, with 20 different amino acid choices at each position, results in 6520 binding combinations – more than the estimated number of atoms in the universe!