Machine learning model can accurately predict liver cirrhosis earlier than ever before
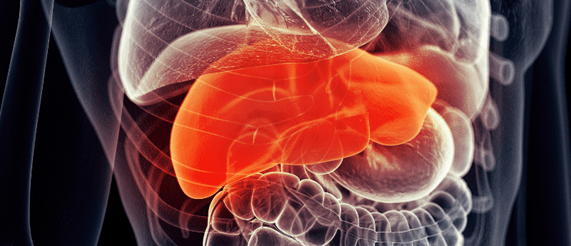
A deep learning neural network model has the ability to accurately predict which patients are at risk of liver cirrhosis by analyzing patient electronic health records (EHRs). This will allow better treatment and management of the condition and improve patient prognosis.
Recently a team at the Medical University of South Carolina (SC, USA), comprised of Don Rockey, Director of the Digestive Disease Research Core Center (IL, USA), and Jihad Obeid, a Professor of Biomedical Informatics, successfully automated liver cirrhosis recognition from patient EHRs using a highly accurate AI model. Historically, extremely difficult to predict patients at risk of liver cirrhosis may now be able to be pinpointed more quickly and receive earlier preventative treatment.
Ever skipped to the end of a book simply because you were bored and wanted to get to the point? Or maybe you skip through episodes in a series because you just did not have the time or attention span to watch every single detail in the whole 47 minutes? But sometimes, these details can be essential and we can miss key, meaningful information.
When it comes to reading or analyzing large amounts of information, it can be difficult not to become fatigued or lose focus. For clinicians, it can be particularly difficult to sift through large amounts of patient data and identify common risk factors that may lead to early disease diagnosis, especially if the condition can be influenced by so many different factors such as liver cirrhosis.
Liver cirrhosis, aka permanent liver scarring, is caused by prolonged damage to the liver over a long period of time. This scarring can occur due to a variety of conditions and diseases, most commonly hepatitis, nonalcoholic fatty liver disease and alcohol misuse. Although liver cirrhosis is considered a leading cause of morbidity and death globally, it is difficult to predict which patients are at risk of developing cirrhosis and there is no cure. Accurate and timely identification of liver cirrhosis therefore remains vital for disease prevention, management and reduction of morbidity and mortality due to liver disease.
“If we could identify a patient predicted to have cirrhosis a year or two earlier, that would give clinicians time to treat and prevent scar tissue from accumulating in the liver but also to prevent complications of cirrhosis,” declared Don Rockey.
Computational approaches have previously been applied to help identify liver cirrhosis patients but with little success. Such approaches have relied on several rounds of keyword searches to navigate narrative text within patient EHRs and these keywords must be supplied by a highly experienced clinician.
Now, Rockey and Obeid have been able to design an AI model that can successfully analyze large patient datasets and identify those at risk of liver cirrhosis. By training a deep learning convolutional neural network (CNN) model with a dataset of 446 patients diagnosed with liver cirrhosis and 689 healthy controls, they were able to identify cirrhosis patients with 97% precision. This CNN model was able to analyze patient EHRs, including clinical text and patient discharge summaries, and pick out key information embedded in the records.
The CNN model also achieved higher precision and accuracy when compared to two baseline comparison models, Random Forest and Naïve Bayes. Furthermore, the CNN model achieved almost perfect precision for cirrhosis identification using only the narrative text within clinician notes.
“Neural networks give more power than a typical statistical AI model because each of these artificial neurons behaves like a model on its own, allowing you to come up with a more sophisticated way of distinguishing and predicting things,” explained Obeid.
Machine learning has the potential to revolutionize medicine and help advise clinical decisions. The team envisions that AI tools will play a crucial role in disease prediction and diagnosis in the future, leading to a more precise estimation of disease burden and improved disease management. The medical ball is now in AI’s court.