Machine learning model could fight the state of ICU delirium
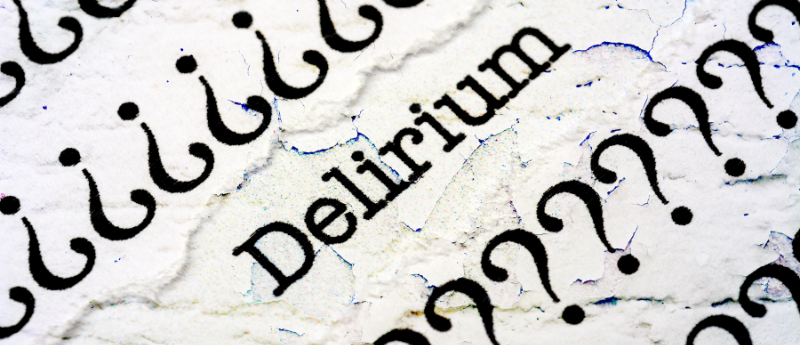
In a recent study, researchers have developed an AI program that accurately predicts the risk of intensive care unit (ICU) delirium.
What is “ICU delirium”? ICU delirium is an extreme state of confusion and anxiety that causes patients to experience changes in cognition, including impaired memory, inattention and disorientation. This mental state is also known to contribute to morbidity and mortality in ICU.
Due to the nature of an ICU environment, patients in this area of a hospital are particularly susceptible to delirium; as many as 80% of ICU patients experience this change in mental state. This is hardly surprising given the combination of harsh lighting, noise and commotion of hospital staff and patients frequently found swarming the ICUs.
Many patients that suffer from ICU delirium and go untreated can even be affected long-term, and may experience cognitive impairment, post-traumatic stress disorder (PTSD) or depression. Consequently, it is pertinent to recognize and treat ICU delirium at the earliest opportunity.
Researchers at the John Hopkins University School of Medicine (MD, USA) have developed a new AI program, which can accurately predict the risk of patient ICU delirium. Minimizing the negative impact of ICU delirium on patient health and the danger it poses to themselves and others.
By applying machine learning to clinical and physiological data from electronic health records, two prediction models were trained and tested to predict ICU delirium. These electronic health records are known to exhibit signatures corresponding to delirium risk.
The first model was a static 24-hour model, which used a snapshot of data from the first 24 hours of ICU admission to predict delirium risk any time during a patient’s stay. This snapshot included details about age, current medications, illness severity, other diagnoses and physiological variables.
The second model was a dynamic model, which used data repeatedly collected over several hours and days, such as heart rate, blood pressure and temperature readings. This was used to continuously predict delirium risk up to 12 hours in advance.
Each prediction model was trained and assessed using a multi-center database (from 2014−2015), and externally validated using two single-center databases with data from over 100,000 ICU stays (from 2001−2012 and from 2008−2019). Each model’s risk prediction performance was compared to results from a widely cited reference model.
Compared to the reference model with a success rate of 73%, the 24-hour model was successful at predicting delirium 78.5% of the time, while the dynamic model had an even more impressive success rate of 84.5%.
At present, the team are performing further testing of the two models on historical patient data. They plan to test the use of the algorithms in a clinical trial and assess how they could impact clinical care in patients admitted to ICU.
Robert Stevens, senior author of the study, declared: “For a lot of these physiological transitions, we think that there are early warning signs that may not be obvious to a clinician but can be picked up on using the kinds of artificial intelligence-supported pattern analysis that we used here.”
These results are hugely promising. Being able to predict ICU delirium will enable interventions to be placed that reduce the harm and risk to the patient. More importantly, these models support dynamic time-sensitive forecasting, which is more accurate at detecting delirium symptoms than current hospital tools.