Man vs machine: an X-ray competition
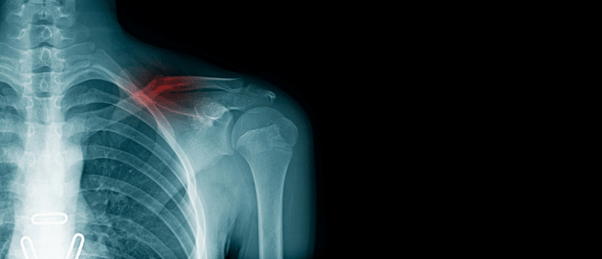
An AI tool’s performance for identifying normal and abnormal chest X-rays has been compared to the performance of experienced clinical radiologists, and the results are remarkable.
A study recently published in Radiology has assessed the ability of an AI tool to correctly identify normal and abnormal chest radiographs (X-rays). A team from the Department of Radiology at the Herlev and Gentofte Hospital (Copenhagen, Denmark) compared the AI tool with the performance of clinical radiologists in a clinical setting, providing the first study of its kind to evaluate a commercially available AI tool for use in clinical radiology. The results signify huge potential for this to relieve the increasingly taxing workload for radiologists globally.
Chest radiography is an important imaging test used to routinely diagnose a broad range of diseases affecting the chest, including the heart and lungs. Chest X-rays can indicate conditions such as pneumonia, cancer and organ abnormalities. There is currently a universal shortage of radiologists while the demand for medical imaging, particularly CTs and MRIs, has risen exponentially.
Accurate interpretation of chest X-rays by the clinician is vital to avoid diagnostic complications. However, chest X-rays are prone to subjectivity variability between physicians and a heavy workload only exacerbates risk of human error. Therefore, improving diagnostic accuracy whilst reducing workloads for radiologists is of paramount importance in this medical field.
In recent years, AI technologies have been developed and tested in radiology to automate the interpretation process of X-rays. However, AI tools must be rigorously evaluated before their enforcement in clinical settings and previous research has lacked sufficient and well-characterized patient samples.
In this study, chest X-ray images from 1529 in-hospital patients, emergency department patients and outpatients were examined by a deep learning AI tool that grouped each radiograph into one of two categories: “high-confidence normal” and “not high-confidence normal”, or normal and abnormal respectively. The deep learning algorithm was trained using approximately 600,000 chest radiographs.
The reference standard was supplied by two board-certified thoracic radiologists and a third radiologist was referred to for any discordant opinions. All three radiologists were blinded to the AI decisions.
The AI tool identified 28% (120 out of 429) of normal chest X-rays and performed particularly well in examining outpatient X-rays. The tool also achieved almost perfect sensitivity for identifying abnormal chest X-rays (99.1% sensitivity) compared to 72.3% sensitivity for the radiologists.
“The most surprising finding was just how sensitive this AI tool was for all kinds of chest disease,” study co-author Louis Lind Plesner noted. “In fact, we could not find a single chest X-ray in our database where the algorithm made a major mistake. Furthermore, the AI tool had a sensitivity overall better than the clinical board-certified radiologists.”
Further research is necessary to assess additional implementation of the AI tool in clinical settings with radiologists as a standardized reference. However overall, the AI tool was identified to safely automate between 6.2% and 11.6% of all chest radiographs, suggesting that it could be a reliable time-saving tool for radiologists, even just for a fraction of automatization.