MRSA who? Deep learning model identifies two novel antibiotic candidates effective against MRSA
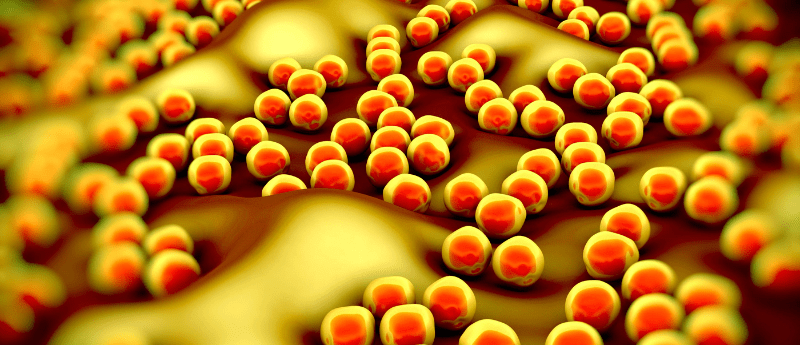
MIT (MA, USA) researchers have leveraged deep learning to identify a novel class of antibiotic candidates that can kill one of the most dangerous drug-resistant bacteria.
Worldwide, methicillin-resistant Staphylococcus aureus (MRSA) is responsible for approximately 35% of bloodstream infections, a figure that has risen by 14% in the last few years. Solely in the US, MRSA causes over 10,000 deaths annually. MRSA infections often complicate medical care, and severe cases can lead to pneumonia, skin infections and potentially fatal sepsis. These statistics emphasize the importance of reducing MRSA circulation and infection rates.
Squaring up to this deadly bacterium, AI has recently been employed to discover compounds that effectively killed MRSA grown in laboratory settings, as well as in mouse models. These compounds also exhibited minimal toxicity to human cells, positioning them as promising drug candidates.
To identify these compounds, the researchers trained a deep learning model with a large dataset of information on 39,000 compounds that had been tested for antibiotic activity against MRSA, including the chemical structures of these compounds. Then, to understand how the model made its predictions, the researchers adapted an algorithm.
The algorithm in question is called the Monte Carlo tree search, a search algorithm that has been previously used to increase the explainability of other deep learning models, such as AlphaGo. This allowed the researchers to unveil which factors influenced the model’s antibiotic potency predictions, creating transparency around the AI’s decision-making process.
Cue the white box. You may have heard of the term “black box” being thrown around in relation to AI. This means that we are unable to see how machine-learning systems make their decisions. This is problematic for a few reasons, including making it difficult to amend the decision-making process when it produces unwanted outcomes, as well as safety concerns due to unwanted biases. Understanding the inner workings of AI systems and how these models are making their predictions, aka a ‘white box’ system, means it is easier for developers to improve design, security and usability. In this case, understanding the model’s decision-making process will enable scientists to design more effective drugs in the future.
Following training, the model then sifted through around 12 million commercially available compounds, identifying five classes predicted to be active against MRSA. Experimental validation pinpointed two promising candidates from the same class, which subsequently demonstrated a tenfold reduction in MRSA populations in mouse models.
These newly discovered compounds kill bacteria by disrupting their ability to maintain an electrochemical gradient across their cell membranes, demonstrating efficacy against Gram-positive bacteria like MRSA while minimizing damage to human cell membranes.
The findings have been shared with Phare Bio (MA, USA), a nonprofit affiliated with the Antibiotics-AI Project, which plans to conduct further analysis for potential clinical applications. Meanwhile, MIT researchers are already exploring the design of additional drug candidates based on the study’s revelations and using the AI models to target other deadly bacteria.