New AI Boosts Early Detection of Vision Loss
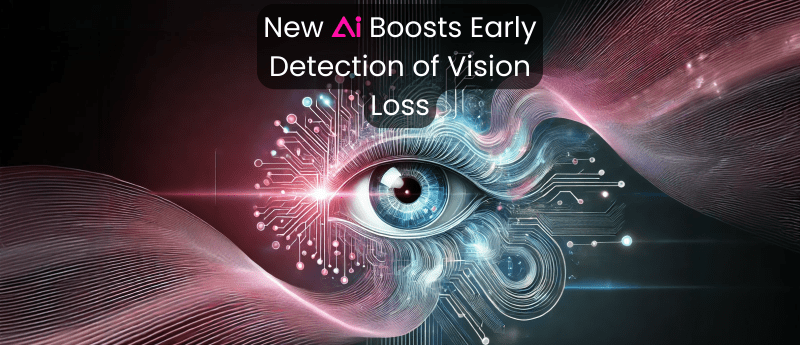
In the world of eye care, a new AI model is showing great promise in improving the diagnosis of macular degeneration, a leading cause of blindness worldwide.
Age-related macular degeneration (AMD) and diabetic macular edema (DME) are two of the most significant causes of vision loss, particularly among the elderly. As the population ages, the number of people affected by these conditions is steadily increasing.
Early detection is critical, but the tools currently available have their limitations, often making it challenging to diagnose these conditions accurately and promptly.
Enter MSA-Net, a new deep learning model that aims to change the tide. Developed by a team of researchers from the Indian institute of Technology, MSA-Net is designed to tackle the specific challenges of classifying Optical Coherence Tomography (OCT) images, a key tool in diagnosing retinal diseases like AMD and DME.
OCT imaging is the gold standard for diagnosing retinal conditions. However, accurately classifying these images is no small task. Traditional methods struggle with two main issues: they don’t always capture all the necessary features, and they often fail to focus on the most critical areas of the retina, like the macular region where these diseases typically manifest.
These shortcomings can lead to misdiagnoses or delays in treatment, which in turn can result in irreversible vision loss.
How MSA-Net Works
MSA-Net, short for Multi-Scale Attention Network, addresses these problems head-on with two major innovations: a multi-scale architecture and a spatial attention mechanism.
-
- Multi-Scale Architecture: Unlike traditional models, MSA-Net processes images at multiple scales. This means it can detect both large and small features within an OCT image, ensuring a more comprehensive analysis. The result is a model that is not only more accurate but also more efficient, requiring less computational power.
- Spatial Attention Mechanism: The second key innovation is MSA-Net’s ability to focus specifically on the macular region, where crucial changes due to AMD and DME often occur. This targeted approach helps the model zero in on the most important areas, making it less likely to miss critical signs of disease.
Performance
The developers put MSA-Net to the test using two major datasets: one from Noor Eye Hospital and another from the University of California, San Diego. The results were impressive.
On the Noor Eye Hospital dataset, MSA-Net achieved a classification accuracy of 98.1%, with a sensitivity of 97.9% and specificity of 98.0%. When tested on the University of California, San Diego dataset, after fine-tuning, it still performed exceptionally well, with a 96.7% accuracy rate.
These figures not only highlight MSA-Net’s precision but also promising robustness across different datasets.
Implications for Eye Care
For ophthalmologists, MSA-Net could be a game-changer. By automating the classification of OCT images, the model can significantly reduce the workload of eye care professionals, allowing them to focus more on complex cases. More importantly, the model’s accuracy means that eye conditions could be detected earlier, potentially saving patients from severe vision loss.
MSA-Net’s ability to consistently and accurately analyze retinal images also opens the door for more widespread screening, especially in areas with limited access to eye care specialists. This could be particularly beneficial in rural or underserved regions, where early detection and treatment are often delayed.
Looking Ahead
While MSA-Net has shown great promise, it’s not without its challenges. The model still struggles somewhat with classifying early-stage AMD, particularly when the features are less distinct. There’s also the issue of class imbalance in datasets, which can affect performance. The developers are already looking at ways to refine the model further, focusing on improving its ability to detect these subtle features and adjusting for imbalanced data.
In a field where early and accurate diagnosis can mean the difference between sight and blindness, MSA-Net represents a significant step forward. As AI continues to evolve, tools like this one could soon become an integral part of eye care, helping doctors deliver better outcomes for their patients.
MSA-Net is not just another AI model; it’s a powerful tool that could reshape how we diagnose and treat some of the most common causes of blindness. By improving the accuracy and efficiency of OCT image analysis, MSA-Net has the potential to transform eye care, making early detection and effective treatment more accessible than ever before.