New test diagnoses ovarian cancer with 93% accuracy
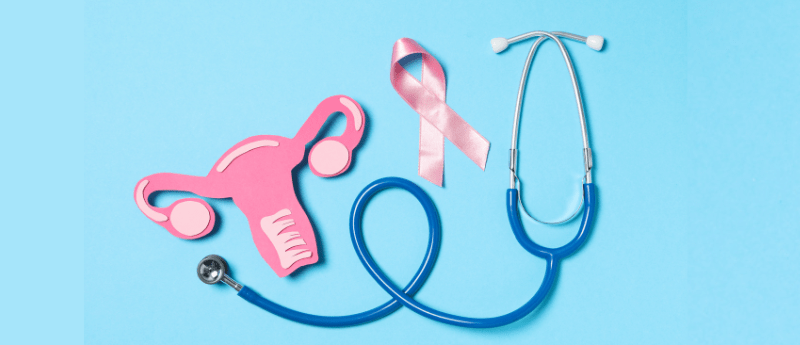
Researchers at the Georgia Tech Integrated Cancer Research Center (ICRC) (GA, USA) have introduced a diagnostic test for ovarian cancer boasting an impressive 93% accuracy.
Globally for women, ovarian cancer is one of the most common causes of cancer-related deaths. In the UK, it is the leading cause of gynecological cancer deaths, above womb, cervical and vulval. Prognosis generally depends on when the cancer is discovered. However, most early ovarian tumors are difficult or impossible to feel, meaning ovarian cancer is typically detected only when late-stage symptoms manifest, for which there are low survival rates.
There is a critical need for an accurate early diagnostic tool. Traditional attempts to create precise early-detection tests have faced obstacles due to the complex and diverse molecular pathways involved in ovarian cancer development.
“Because of high-level molecular heterogeneity among patients, the identification of a single universal diagnostic biomarker of ovarian cancer has not been possible,” explained John McDonald, a professor at the School of Biological Sciences at the ICRC.
Addressing this challenge, McDonald and his team employed a novel approach, integrating “logic-based mechanistic machine learning.”
Focusing on metabolic profiles obtained through mass spectrometry, researchers analyzed serum samples from 431 ovarian cancer patients and 133 healthy women for ovarian cancer biochemical markers, offering comprehensive insights into individual metabolic states. These samples were collected from four geographically diverse locations to ensure metabolic data quality.
Leveraging machine learning selection and performance optimization methods (recursive feature elimination coupled with repeated cross-validation), the ICRC team identified the most reliable metabolites. Using these results, the team then developed a consensus classifier (final machine learning model) that distinguished cancer from control samples with a remarkable 93% accuracy.
“This personalized, probabilistic approach to cancer diagnostics is more clinically informative and accurate than traditional binary (yes/no) tests,” McDonald commented. “It represents a promising new direction in the early detection of ovarian cancer, and perhaps other cancers as well.”
While the model requires further fine-tuning and improvements, its potential to detect ovarian cancer before clinical symptoms appear opens new avenues in the early detection landscape, as well as for other cancers.