Novel 3D Cell Mapping Could Slash Drug Discovery Times by 50%
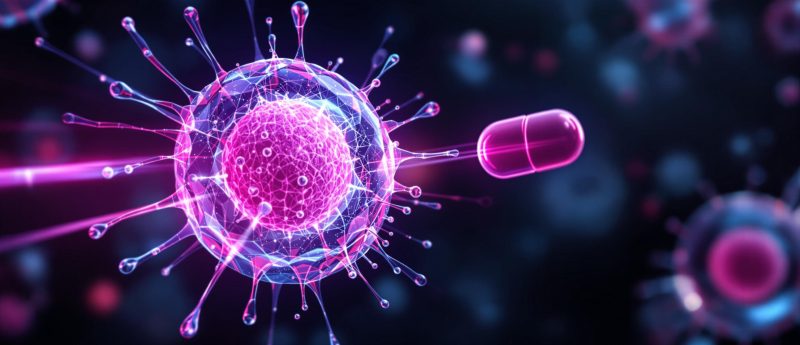
Researchers at the Institute of Cancer Research (London, UK) have developed a new AI model that predicts drug responses based on 3D cell shape changes. Experts believe this could halve the time to deliver new cancer treatments to patients.
The AI model, “MorphoMIL,” predicts cancer drugs being tested on melanoma cells with 99.3% accuracy. According to the Institute of Cancer Research (ICR), this could avoid time-consuming and costly biochemical experiments to test a drug’s effect, potentially shaving years off the drug discovery process.
Cellular Shape is “Like a Fingerprint” of State and Function
A cell’s 3D shape is far more than a rigid structure. Cells are a dynamic and endlessly complex hub of activity—within them, roughly 42 million protein molecules work together to carry out functions essential to life. Because structure closely mirrors function, any shifts in activity on the inside can alter the way a cell looks on the outside.
Cancer cells, for example, are notoriously “potent” biochemical factories, reprograming a cell’s metabolic circuitry to maximize growth and minimize the risk of detection by the body’s defense systems.
While this inner rewiring is invisible, the outward effect is distinctive—scientists often describe tumor cells as having “irregular” shapes with unusual coloring when examined under a microscope.
Using Cell Shape to Dissect Drug Behavior
Perhaps more intriguingly, different compounds can directly induce changes in cellular shape, offering a way of predicting a drug’s effect based purely on visual cues.
A 2013 review, published in Cell, found significant variations in cell appearances that correlated with a drug’s mechanism of action, emphasizing the power of the “morphological fingerprint” in understanding how treatments work.
Since the 1900s, researchers have relied on 2D microscopy to make these observations, using biological stains to visualize structures under a microscope. But cells don’t grow in 2D—it’s like trying to understand the ocean by only looking at it from above: you see the surface but miss everything beneath.
What’s more, analyzing microscopy images to gauge drug activity is exhaustive and inefficient—despite a shift towards computational methods, subtle details can still be easily missed from the thousands of features to look at.
To truly understand how cells change in response to a drug, researchers need more powerful techniques.
Cracking the Code with AI: A Deep Dive into MorphoMIL
In a paper recently published in Cell Systems, ICR researchers propose a solution. Trained on nearly 100,000 3D microscopy images, MorphoMIL allows scientists to easily understand how a drug works—crucially, based on cell shape changes alone.
The model could even detect subtle differences caused by drugs that —despite targeting slightly different proteins— had similar overall effects on cells.
But with “innovative” AI technology coming out all the time, what makes this one any different?
At its core, MorphoMIL marries two key concepts: geometric deep learning (GDL) and multiple instance learning (MIL). While not new to AI, combining these two approaches marks an important step for this area of research.
- Geometric Deep Learning — A branch of deep learning that handles data outside of a regular grid —such as 3D cell representations— where structure is irregular or spatially complex.
MorphoMIL uses GDL to reconstruct cell shapes based on 3D point cloud data—in essence, a collection of (x, y, z) coordinates that represent key features of each cell.
Visual representation of 3D point cloud data. Image generated with Gemini.
Using these coordinates as a guide, the model etches a graphic representation of the cell—first “encoding” into a compressed version, then “decoding” into a higher-resolution one. This process follows a graph-based autoencoder architecture, teaching the model to reconstruct the cell by initially picking out “big” details, like overall shape, before zooming in on the finer details, such as surface protrusions.
Think of it as giving a blueprint to a builder. The builder will use the blueprint to build a house, starting with the foundations and then moving on to finer details like rooms, doors, and windows.
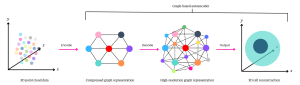
Visual representation of MorphoMIL’s geometric deep learning process.
- Multiple Instance Learning — Where an AI looks at “bags” of data rather than individual instances. Here, this means that MorphoMIL examines groups of cells to make its predictions, reducing the impact of subtle differences between cells that might otherwise skew results. However, the researchers modified the MIL framework ever so slightly. They incorporated an “attention-based mechanism,” instructing the model to focus on cells that are most important for accurate predictions—by doing this, they could shift attention away from “noisy cells” that responded unusually to a drug.
Why This is Important
Together, GDL and MIL act synergistically to deliver some major advancements in the field. Specifically:
- GDL ensures that MorphoMIL can effectively model the spatial complexity of a 3D cellular environment, embracing both the major and minor details of cell structure. While other studies have also used AI to predict drug response from cell shape, many were relying on convolutional neural networks—optimal for grid-structured data but less suited for capturing the nuances of 3D shape.
- Attention-based MIL allows MorphoMIL to look at shape changes to both individual cells and at a wider population level, minimizing noise in the data while still retaining high-resolution analysis. While MIL is not uncommon in this space, integrating attention-based learning into the MIL pipeline is a relatively new approach.
MorphoMIL: Making Drug Discovery Faster and Cheaper?
Normally, delivering a drug from concept-to-market is a lengthy, expensive process—scientists need solid evidence that it both works and is safe for humans.
In the early stages, this features many different biological tests to piece together how a drug behaves, many of which are not only time-consuming but also costly. Later down the line, researchers assess a drug’s performance in patients, often without knowing how well it will work across different populations.
All in all, if a new cancer drug were discovered today, it would take around 12–15 years —and ~$1.3 billion— before it reaches the patients who need it.
With MorphoMIL, scientists can rapidly assess a drug’s effect just from a cell’s shape, bypassing the need for labor-intensive experimental validation. The ICR expects this could save a significant amount of time—especially during the initial phases of drug discovery, clipping the timeline from three years to three months.
Chris Bakal, Professor of Cancer Morphodynamics at the ICR, says: “The tool that we’ve created is so powerful that we will be able to streamline the years-long drug discovery process, saving both time and money. Patients with cancer need new treatment options as quickly as possible, so speeding up this process will be hugely valuable.”
Limitations to Consider
While the results look promising, there are a few things to keep in mind.
Firstly, the researchers acknowledge that the model is “rotationally invariant,” meaning that it doesn’t always recognize cells in the same way if they are turned in different directions. In future, this could be addressed using point-based representations rather than a graph-based approach—essentially, this would cut out the middle step of graph construction and directly “build” a cell from 3D coordinates.
Some aspects of the learning process are also computationally expensive, which could be mitigated with simpler models that are “lightweight,” as reported by the authors.
Regardless, this is an exciting paper that brings novel AI techniques to this area of research. With new technologies continually emerging in the drug development space, it will be interesting to see whether the momentum behind AI translates into meaningful impact—at the peak of its potential, patients could gain access to life-saving treatments faster than ever before.