Oculomics | Where the Eye Meets AI
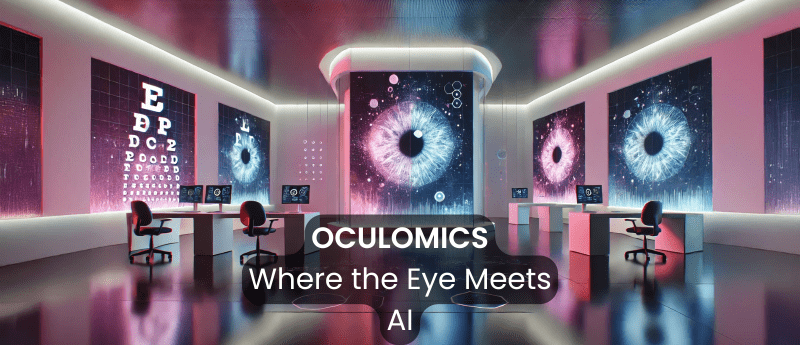
Harnessing advanced imaging technologies and cutting-edge AI, Oculomics reimagines the role of the eye in healthcare, unlocking faster and more accurate ways to diagnose systemic and neurological conditions. We had the pleasure of speaking with Dr. Seigfried Wagner, Honorary Clinical Senior Research Fellow at University College London (UK), to delve deeper into this groundbreaking field, uncovering the latest advancements and their implications for the future of healthcare.
Oculomics: Made Possible by the Invention of OCT
Physicians have long recognized that systemic diseases can manifest in the eyes, impacting the appearance and health of the eye. As early as 1898, Marcus Gunn observed that retinal blood vessel narrowing was linked to hypertension. However, the term ‘oculomics’ was only recently coined in 2020, first appearing in an article published in Translational Vision Science & Technology.
This breakthrough in oculomics was largely enabled about by the development of Optical Coherence Tomography (OCT), a technique that captures high-resolution, cross-sectional scans of the retina. These scans reveal detailed structures, including retinal thickness and morphology.
“OCT uses near-infrared light to measure backscatter from different tissues of the back of the eye. It’s like an ultrasound, but it’s not using sound, it’s using light,”
Dr. Siegfried Wagner, Honorary Clinical Senior Researcher Fellow, UCL.
OCT images allow scientists to uncover unexplored connections between retinal features and systemic diseases, effectively, using the eye as a “window to the body”.
Revolutionizing Oculomics: From Manual Analysis to AI-Driven Insights
Initially, researchers manually segmented OCT scans to identify links between retinal features and systemic health. This process, while insightful, was time-consuming and unsuitable for large-scale study.
The introduction of semi-automated retinal imaging analysis software improved efficiency and sped this process along, but still required researchers to manually correct machine-made errors in vessel segmentation, demanding extensive time and effort.
The advent of deep learning has since transformed the field. Now, some leading experts in the field are building novel AI models to analyze retinal scans rapidly, uncovering patterns that were previously decipher the connections between ophthalmology and overall health.
These algorithms could build tools capable of predicting diseases from a quick and non-invasive scan of the eye, cutting back diagnostic wait times.
There’s More Than Meets the Eye
Researchers have discovered that small changes in the eye, like microvascular density or retinal thickness, can indicate a host of life-threatening diseases. For example, one group recently found that microvasculature density in macular regions is significantly lower in stroke victims. Other groups have found evidence for ocular changes associated with periodontitis (gum disease), Crohn’s disease, hypertension, chronic kidney disease (CKD), and anemia.
Perhaps even more interestingly, a recent study conducted by scientists at the University of Edinburgh found that these ocular changes can occur very rapidly. For example, choroid thinning is a documented phenomenon in patients with CKD – however, a recent study revealed that just one week after CKD patients had received a kidney transplant, the thickness of their choroid significantly increased.
These finidngs highlight that the eye is incredibly dynamic and responsive to systemic changes, underscoring the field of oculomics as a promising diagnostic strategy.
Using the Eye as a Proxy to the Brain
The retina shares its embryonic origins with the brain, making ocular imaging not only useful for predicting systemic disease; neurological disorders also manifest in the back of the eye. Thus, changes to the central nervous system (CNS) often influence the eye’s biological appearance.
Crucially, a whole array of diseases involving the nervous system can also lead to retinal thinning. Scientists in the field are therefore currently focused on using the eye to monitor how diseases progress in patients who are already diagnosed:
“A lot of central nervous system diseases will cause this, so we’re seeing something that’s sensitive, but not very specific. What’s interesting is if this could be used for disease monitoring,”
Dr. Siegfried Wagner, Honorary Clinical Senior Researcher Fellow, UCL.
A key example of this is Parkinson’s disease (PD), a disease characterized by the progressive loss of dopamine-releasing neurons in the brain. Patients often experience motor dysfunction (including tremors and slow movements), and non-motor symptoms such as anxiety and depression, sleeping problems, fatigue, and pain.
Numerous research groups worldwide have investigated the types of changes happening to the retina that occur in PD, finding that significant thinning of the macula’s retinal nerve fiber layer is associated with more advanced PD.
By acquiring data on the genetic and phenotypic characteristics of diseases, automated tools in the future might be able to diagnose specific diseases early on, perhaps before the onset of symptoms, without any invasive diagnostic procedures. This is particularly valuable for neurodegenerative diseases that are incurable once symptoms appear.
AI-Driven Integration of Oculomics into Clinics
In the more immediate future, researchers hope that AI will be able to easily and quickly analyze microscopic details in retinal scans or images, helping clinicians monitor disease progression.
A pivotal study, published in Neurology in 2023, exemplifies this.
A research team, led by Siegfried, used two huge datasets, AlzEye and BioBank, to find associations between the retinal thinning of a specific region known as the ganglion cell inner plexiform layer, and rates of neurodegeneration in Parkinson’s disease.
The analysis of this vast amount of data was made significantly easier by a type of imaging software called the Topcon Advanced Boundary Segmentation (TABS) tool, which rapidly and automatically measures retinal thickness.
This software incorporates AI to segment digital OCT scans with high precision using dual-scale gradient information, combining both fine and course details. This allows the tool to look at retinal features both on a micro- and macro-scale, helping to smooth out any noise in the data that might affect the AI’s performance, i.e., artifacts in the images.
Siegfried remarks:
“I continue to be amazed by what we can discover through eye scans. While we are not yet ready to predict whether an individual will develop Parkinson’s, we hope that this method could soon become a pre-screening tool for people at risk of disease.”
He then continues to say: “Finding signs of several diseases before symptoms emerge means that, in the future, people could have the time to make lifestyle changes to prevent some conditions arising, and clinicians could delay the onset and impact of life-changing neurodegenerative disorders.”
Despite this huge advancement, TABS still has its limitations for ocular-based disease monitoring.
For example, some features like image cropping (which helps exclude irrelevant areas from images, e.g., eyelashes, as well as cropping artifacts in images) and quality assessment are not integrated into the TABS workflow.
This means that noise and poor-quality images have to be manually filtered by researchers, significantly adding to the time needed to use this tool.
Ideally, AI software used in this capacity should incorporate these features automatically to maximize its potential for oculomics.
Building Bigger and Better AI Tools: AutoMorph
Siegfried has also been involved with the development of a deep learning pipeline that aims to overcome these issues, which is called AutoMorph.
AutoMorph simplifies analysis by automatically cropping images to a standardized size, grading image quality, segmenting anatomical features like veins and the optic disc using advanced neural networks, and quantifying retinal attributes such as vessel tortuosity and optic disc ratios. This automation allows AutoMorph to automatically analyze OCT scans without the need for human input, saving a significant amount of time and potentially freeing up healthcare resources.
Using AutoMorph to Gauge Neurodegeneration in Schizophrenia
Excitingly, AutoMorph’s applications could extend beyond Parkinson’s disease or systemic diseases; it could also have the power to transform schizophrenia clinical management.
In September 2024, a paper published in Elsevier set the scene for future developments. A team of scientists, led by Siegfried Wagner, used AutoMorph to evaluate retinal images from over 35,000 patients affiliated with UK Biobank.
This time, the type of images used were taken using a combined mixture of OCT, OCT angiography (OCTA), and color fundus photography (CFP). OCTA and CFP visualize the vasculature of the retina, helping researchers to get a more complete picture of the eye, while OCT is more useful for evaluating retinal layers.
In patients with schizophrenia, retinal thinning is a well-documented phenomenon, often targeting the macular ganglion cell-inner cell plexiform layer (mGC-IPL).
The group used AutoMorph to segment CFP images, focusing on detailed features like micro vessel width, tortuosity, and optic cup-to-disc ratios. To look at broader features of retinal morphology, such as mGC-IPL and RNL thickness, they enlisted the help of TABS.
Indeed, the study revealed that a high polygenic risk score of schizophrenia was associated with a significantly thinner mGC-IPL, irrespective of factors like age, diabetes, hypertension, smoking, and cholesterol levels. However, vascular changes (like tortuosity, and blood vessel width) were not significantly correlated with the schizophrenia risk score.
The group’s findings illustrate the potential of AI-guided imaging tools to detect subtle changes in retinal morphology, helping to quickly and easily monitor diseases that profoundly affect one’s quality of life. This could inform clinical decision-making, helping doctors to select optimal treatment strategies for their patients.
Siegfried Wagner stated:
“When we speak to the psychiatrists, they love the idea because it’s so hard to get brain imaging from their patients. If you can just do a point-of-care bedside test and get a nice quantitative measure of disease progression, it’s very scalable.”
Conclusion and Future Perspectives
Oculomics offers the potential to determine overall health from a quick snapshot image of the eye. In the past, this concept has been inaccessible due to the exhaustive manual labor required to analyze and annotate ocular images from patients.
Now, in the age of artificial intelligence, researchers can build models that easily segment these images, picking up on intricate patterns in datasets that might otherwise be missed. Evaluating these features would then allow clinicians to track disease progression in patients with non-invasive methods.
This being said, using AI in this capacity is still far from being ready for routine use in the clinics. Some concerns need to be addressed before this new AI-based technology can utilize oculomics for disease monitoring or diagnostic procedures. This includes factors such as health & data equity, model validation, healthcare professional training, and ethical concerns.
Nonetheless, the world of AI is moving fast, and it will be exciting to watch how cutting-edge machine learning tools bring this emerging field into the orbit of clinical communities.