AI for Medical Imaging and the Future of Healthcare | Ángel Alberich-Bayarri | Quibim CEO
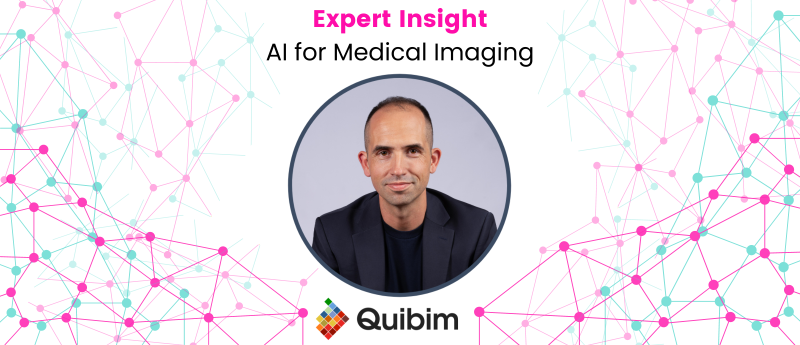
In this illuminating interview, Ángel Alberich-Bayarri, the CEO and co-founder of Quibim (Valencia, Spain), shares his journey from a biomedical engineer, to leading a pioneering company at the intersection of AI and radiology. Quibim (Quantitative Imaging Biomarkers In Medicine) embodies Ángel’s vision of transforming radiology into a more objective science by developing imaging biomarkers akin to traditional blood or urine tests. He details the evolution of Quibim from its research-focused beginnings to a company that now boasts FDA and CE mark clearances for its innovative AI-driven diagnostic tools, such as those for prostate cancer detection and non-invasive liver disease assessment. Ángel also discusses the challenges and breakthroughs in AI implementation, Quibim’s cloud-based business model, and the significant partnerships that support their backend compute needs. Looking forward, he envisions Quibim leading a healthcare revolution towards preventive care through advancements in whole-body MRI and AI, driven by the ambition to create digital twins of humans, reflecting his motivation to innovate and reshape healthcare.
Please could you provide an overview of your career to date?
So, you know, I’m not a doctor. I’m a biomedical engineer. I did a PhD in computer vision applied to medical imaging to magnetic resonance imaging (MRI) data. And basically, we saw that there were some parameters that could be measured out of the MRI images. And I was always inspired by biomarkers from blood, from urine, you know, that when you have a sample and you can convert it into a series of measurable quantities. And I said, ok, I want to do the same with images. And then, you know, I had a colleague that later became Quibim co-founder, who is a key opinion leader in radiology. And it was clear to us that radiology had to change to become a more objective science because I was coexisting with radiologists in the same workspace and I could see how they were reporting a brain. They were saying expressions like this brain has a moderate atrophy or grey matter has a moderate atrophy. Right. So I was always struggling on what is moderate. So how different it is from a high risk or from a low risk. Where are the cut-off values? So obviously, of course, there were no responses to that, other than “well, according to my experience, the impressions I see, the size of the ventricles that I see more or less reflects that this is a high atrophy” or whatever. So that forced us trying to, you know, to start trying to develop a new space of imaging biomarkers. That’s how we called it. We published a lot in research and we wrote the main guidelines in imaging biomarkers quantification. We funded the European Imaging Biomarkers Alliance. And then we have been very much involved in all the imaging biomarkers spaces everywhere. That’s what ended up giving name to the company. That’s Q-U-I-B-I-M. It’s quantitative imaging biomarkers in medicine. It’s a meaning.
So, you know, after these years of research, in algorithms for brain, for liver, for musculoskeletal, for cancer, when I was 30, I decided to buy the majority of the shares of the company and to be the CEO and to start hiring people. In 2015, I hired the first employee. I started to convert all my research into code, into product. And it was tough because at the very beginning, there was a lot to do in converting all the research and getting evidence and converting it into product. But in 2018, I started having the first CE mark clearance. And, you know, then 2019, we filed for FDA. One of the very first tools we filed was on the prostate cancer detection using AI. And for that, we have trained AI models based on convolutional neural networks that we used to learn to detect the organ and learn to find the lesions. And the brain was also another area that we had very much implemented. So we ended up also converting it into a robust product mainly for Alzheimer’s disease and multiple sclerosis. So we were characterizing the gray matter atrophy, the volumetry of the gray matter per regions, and being able to tell whether the patients are out of the health range or, you know, they are in depending on the age. And then on the multiple sclerosis, trying to use AI also to detect the brain and to extract potential lesions in the white matter. And for all that, we use ensembles of convolutional neural networks that have been trained in many patients. And yeah, we achieved FDA, CE, and UKCA for that. And that’s an example.
Another product coming to the more recent news that we had in the pipeline was in the area of metabolic diseases, metabolic syndrome. We see a lot of, you know, obesity, diabetes in the current landscape, and that’s only going to grow, right? We see more and more young people with metabolic issues. And one of the big ones is, you know, fatty liver disease, iron deposits in the liver. And actually, the coexistence of fat and iron in the same space in the liver can lead to cirrhosis, right? It has a potential to lead to cirrhosis and other areas. So today, the only way you have to monitor that, if you want to do that accurately, is with a biopsy. So you need to do a biopsy in the liver. Usually, it’s a frequent biopsy every six months, one year, but that’s a really uncomfortable procedure. It has risks of infection. The needles are not that thin, so it’s an invasive procedure. What we want to do is like, okay, can we monitor the blood pressure? Can we monitor the iron and the fat in the liver pixels, so that we can see in which areas we have a higher risk of having a most relevant disease, like a fibrosis or a cirrhosis, right? And ultimately, this has led basically to another application, especially after the development of the new drugs. We have Ozempic, Binobo, and we have other similar drugs that basically are having an effect of reducing the fatty amount, the fatty liver disease. And you can monitor the effectiveness of these drugs using our tool. This would be a great application. Non-invasively, you can see how the liver is changing through time after the drug administration. So, that’s one of the most relevant applications.
The technique is very fast. It’s very fast. This is an MRI sequence that is done in a breath hold, in a single breath hold. So, 24 seconds done, so all the images are acquired, yes. And then the analysis is based on AI. So, AI detects the liver organ, detects the abdominal perimeter also, and then from the liver organ, it’s quantifying the fat and iron, right? And it provides the results in a report.
How is Quibim’s software currently being used by customers?
Yeah, so right now, I mean, the company, Quibim, we sell to hospitals our solutions, software as a medical device, like QP-Liver. These are tests that apply to a single individual. You analyze one MRI and then it gives you the results like a blood test. It’s one patient. But we also have among our customers, big pharma companies that analyze cohorts of patients. For example, if they want to find patients for a new drug, they can use our tools to say, okay, we want to find patients in a hospital with a fat delivery disease above 15%. Then they can use our tools to do that.
We have a database ourselves, fully anonymized, and we also do agreements with the hospitals when they are interested to having this kind of impact in healthcare. Say, okay, do you allow us to have an anonymized use of your data for research to improve the detection of new potential molecules that might treat these patients? And usually in the frame of projects or collaborations, hospitals will accept. And through years we have been building our own database. And there are drugs like, for example, Resmetirone. That’s a metabolic associated liver disease. And that’s one of the hot topics right now. And also, I had here in my notes that recently there are new guidelines from the European Association for the Study of Liver and Lancet. There’s a commission for the early identification of progressive liver disease instead of focusing on end-stage cirrhosis, trying to focus on progressive liver disease. And therefore, using our tool might allow to align with these guidelines and to follow up this progression on liver syndrome rather than just screening population for cirrhosis, which is too late.
What sort of challenges have you faced with regards to using AI for your products?
One challenge is related to the process of manufacturing or training the AI models themselves because you need data and you need access to high quality data. And then you need to end up signing agreements with hospitals, doing research projects to allow them to have access to this data to be used for training. And that’s a big bottleneck that not all startup companies can afford because you need muscle, you need energy to go with every single hospital signing and that takes time, IRBs (institutional review boards) take time. So that’s definitely data access is one of the first challenges we have and we need to sort it out. And then the second is implementation.
So implementation is also relevant. Once you have an AI model, probably sometimes if you asked to a hospital manager, they would tell you, no, I want an on-premise solution. I don’t want my data to leave the hospital. But that is not really agile with AI. AI needs a lot of upgrades, frequent upgrades. We are used to that. Now we have a phone and all applications are being updated one day after the other. The idea of putting an AI tool that you designed to identify patients in a hospital, in an on-prem mode means that it will be static, right? If it will be in the cloud, it allows us to update it in real time. Of course, this is a medical device. So we need to send all the notifications and explain to the customers when these upgrades happen, what is changing, what is not changing and all the documentation on the products is for that. And there is already regulatory aspects in the medical devices that go for it. But at Quibim, we really believe in the business model based in the cloud. And that was also a challenge to convince and to create a focusing on the importance of cloud for AI, because you need to do updates on the versions of the algorithms, you know, with a certain frequency. And that means you need to convince hospitals to work in the cloud. And the way we did that was basically trying to smooth all the customers’ journey. And one of the developments that allowed us to scale was basically a tool we created called QP-Link that we don’t even need to install ourselves to the hospitals. We just give the URL where the hospital IT managers can download it and they install it themselves when they want. They don’t need to give us access remotely. So they are extremely accessible. Yeah, they are sometimes scared of opening doors for third parties to install stuff in hospital networks. We just tell them, listen, here you have the tool, the QP-Link that connects with our cloud. Just download it, install it whenever you want. QP-Link does not need to enter into your hospital network to your premises. So just install it, it will connect to our cloud and your hospital will be live. And that is working great for us to deploy fast. Right now we can onboard a hospital in less than a morning, right? In just a few hours.
Can you tell me a bit about the back-end compute required for your platform?
So the biggest compute is mainly related to the manufacturing process, so the training of the algorithms. And for that we need access to machines like DGX for example. Right now the partner we work the most with is Nvidia of course. It’s a company providing most of the GPUs (graphical processing units) on the planet, the GPUs that we use for training the models. And when you train the models you need an immense amount of computing performance, right? We can be days, weeks or months training those models. And once they are trained, the good thing of it is that the inference does not take that long and that a high-performance computing to execute. That means we can allocate them in machines or in servers with GPUs or even CPUs (central processing units) in some cases which makes our cost go down once you are in production. You don’t need that compared to the training phase, you don’t need that high performance computing as compared to training.
There is obviously a high opportunity cost with all the training for a given algorithm, so how did you decide on QP-Liver as your next product over something else?
It has many factors, right? One is the ongoing, I would say, epitome of obesity that we have. The need for really objective tools to quantify the status of the internal organs. So I really believe there is an unmet need right now on trying to know the age of our liver, how are we internally. The reason for an unmet need is because nowadays this is done with invasive procedures, this is done with a biopsy which could be avoided in many situations like follow-up. So that unmet need added to the fact that in our first research experience we started to see that the accuracy was insanely high. I mean when we created the first imaging biomarkers to quantify the liver, we saw that the error we were doing with regards to biopsy was very, very low and the correlation was almost one with digital pathology. So we took the liver tissue and we analyzed all the liver fat in the slides, compared it to the measurements we were obtaining from the MRI, and the correlation was very close to one. That is when we said, okay there is a clear unmet need plus we have an accurate technique, so we have a product, right? And that’s what motivated us increasingly to build it as a product and to commercialize it. And right now I think that the product has also a hypotension, not only because this diagnosis, which is okay, but also from the monitoring of treatment effects, right? And hopefully in the years to come we will see how the effect of these treatments will need to be monitored with this kind of objective techniques.
So what do you envision for the future of Quibim? How do you envision it changing in the years to come? Particularly considering the profound increases in compute and AI capabilities.
That’s a great question. Today I would say our acquisition capabilities in terms of imaging and our computational performance, let’s say, has been mainly limited in some cases. That’s why we ended up having to develop solutions for the liver, for example, I mean specific to the organ. So still when you do MRI examinations, MRI is great, by the way, because it’s non-ionizing, so you can repeat it whenever you want and this will be very important for what I want to say. But MRI is still mainly designed to provide high resolution only when you measure body part by body part. Still we don’t have absolutely high quality on doing whole body MRI examinations, but I think in the years to come the improvement in whole body MRI, like having high spatial resolution, organ level, acquiring an entire human body is great because it will allow us not only to analyze the liver but also to analyze the whole system, right? I mentioned previously metabolic syndrome, metabolic syndrome is not only about the liver, it’s also about the pancreas, it’s also about the spleen, vertebra, bone marrow, fat, so we have a lot of areas, visceral fat areas that we can explore. I clearly see that the combination of the improvement in imaging exams resolution plus in access to computing performance, allowing us to analyze bigger and bigger volumes of data, will allow to move imaging to be a reactive technique that is today used in a sick care model to a preventive healthcare model. And MRI, as I mentioned, can be repeated at any time.
I clearly see that in the next 10 years we will be more and more like care providers providing preventive health check-ups based on whole body MRI. Ultimately AI tools will be able to be trained also to analyze the whole body and to be able to provide what is our inner age, right? How are we? I mean, because doing a genetics test is great, doing a blood test or, you know, doing exercise is great, but we might have an abnormality inside in an organ that we need to spot early to save our life. And I really think that having tools based on AI that analyze the 3D volume of our human body and tell you, okay, in this area, your spleen is different than in the average population, right? I think it will be important to achieve health assurance. Instead of the sick care model that we have today that is all symptomatic, right?
What is your greatest motivation for your work (i.e. what gets you up in the morning)?
Yeah, what I get excited about is on the revolution we are living right now and having had the chance to create a leading company that, you know, initially we were very intensive research in the area of imaging biomarkers, but later we saw a gap between our research and what we could find in the commercial scanners, for example. And right now I clearly see that being in this leading position is allowing us to create whatever we want, right? I don’t really see limits and that’s what motivates me. For example, what I was just mentioning, I clearly see that if there is someone that has to lead that innovation, it’s ourselves, right? We have learned throughout these years to characterize our inner bodies, organ by organ it’s true, but we are ready to be able to end up, you know, making a significant leap into building the digital twins of humans in the near future, right? And I think that ambition is really what motivates me and I cannot stop thinking about it.
Interviewee profile:
Angel Alberich-Bayarri is the CEO and co-founder of Quibim, a global company specializing in designing and developing cutting-edge tools that merge advanced medical image analysis and AI to achieve more precise diagnoses and suitable treatments. He holds a degree in Telecommunications Engineering from the Polytechnic University of Valencia and a doctorate in Biomedical Engineering. He is the inventor of 6 patents and has received numerous international awards for his innovative work, including the MIT Innovators Under 35. With 15 years of experience in the field of medical imaging and computer vision, he possesses deep knowledge of the challenges and opportunities in diagnostics and drug development. Previously, he served as Corporate Director of Biomedical Engineering at Quirónsalud and as Scientific-Technical Director of the Biomedical Imaging Research Group at the University and Polytechnic Hospital La Fe. He has authored over 100 articles in prestigious international journals and is a featured speaker at major international conferences. In the social sphere, he serves as a Trustee of the Conexus Foundation and is the founder of the Imaging Foundation, among other initiatives aimed at promoting knowledge and science.
Disclaimers:
The opinions expressed in this feature are those of the interviewee and do not necessarily reflect the views of Future Medicine AI Hub or Future Science Group.