SLIViT AI Equals Human Clinicians in Medical Imaging Accuracy
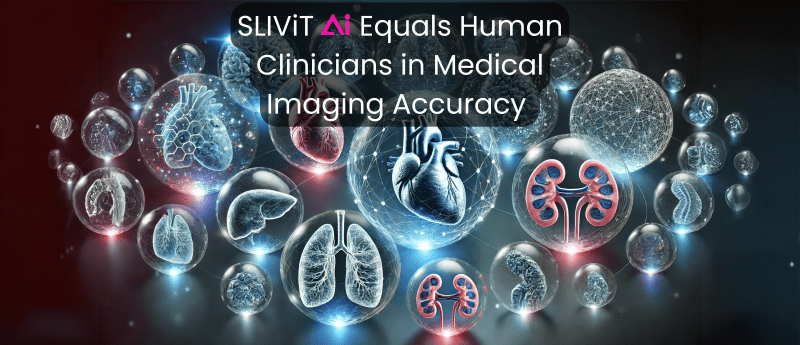
An AI system developed by researchers at the University of California, Los Angeles (UCLA; CA,US) has matched the precision levels of clinical specialists in analyzing detailed medical imaging procedures including magnetic resonance imaging (MRI) and 3D scans.
SLIViT (SLice Integration by Vision Transformer), has been tested on a variety of imaging data, such as three-dimensional retinal imaging, ultrasound scans, and MRIs for liver health evaluation among others. This presents an innovative approach to clinical imaging, as existing AI models are often trained by concentrating on a single form of scan or specific diseases.
The results of the study have been published in the journal Nature Biomedical Engineering.
An Increasing Need for Speed
Clinical imaging plays a critical role in early disease detection, diagnosis, and treatment. However, 3D scanning procedures, such as MRI and ultrasound, can be complex, as they involve capturing and analyzing width, height, and depth data, which must then be translated for clinician interpretation.
This complexity often contributes to lengthy patient waiting lists. For example, in Canada, wait times for MRI services ranged from 65 to 105 days last year. In England, 26% of patients requiring an MRI waited over six weeks in 2022/23. Similarly, in Norway, data from the Norwegian Directorate of Health showed that between 2018 and 2021, the average wait times for MRI scans and ultrasounds were 8.7–12.0 weeks and 7.9–11.4 weeks, respectively.
Key factors driving these delays include limited resources, such as a shortage of devices and staff, outdated or insufficient equipment, and challenges in scheduling and resource management.
These prolonged wait times not only complicate patient care, as medical conditions may worsen due to delays in diagnosis or treatment, but they also erode patient satisfaction and trust in the healthcare system, potentially discouraging future timely care.
How Can SLIViT Help Make a Change?
To create 3D images, artificial neural networks analyze large datasets that frequently focus on a single type of scan or target specific diseases.
In contrast, SLIViT approaches this procedure differently. By integrating two AI components, the system employs an innovative learning method that assesses illness risk from different volumetric modalities rather than focusing only on a single type.
Additionally, the system has also demonstrated increased effectiveness in real-world conditions by relying on a lower number of training datasets than other models.
By moving away from more limited information compilations to larger volumetric dataset analysis, SLIViT could serve as a foundational model for developing future predictive AI models, potentially enhancing and speeding up current medical imaging services.
Impressive Results
The UCLA study found that SLIViT matched the accuracy of human clinicians and more specialized models in identifying disease risk markers.
“We show that SLIViT, despite being a generic model, consistently achieves significantly better performance compared to domain-specific state-of-the-art models,” explained UCLA PhD student Berkin Durmus. “It has clinical applicability potential, matching the accuracy of manual expertise of clinical specialists while reducing time by a factor of 5,000. And unlike other methods, SLIViT is flexible and robust enough to work with clinical datasets that are not always in perfect order.”
The discovery of SLIViT could represent a significant improvement in medical imaging, as it not only equals the diagnostic accuracy of human doctors but also greatly simplifies the process. By demonstrating the capacity to efficiently manage large clinical datasets, SLIViT could pave the way for greater use of AI technologies in medical imaging, ultimately increasing diagnostic capabilities and patient outcomes across a wide range of healthcare settings.
Near Future Challenges
Despite the increasing application of AI in modern medical imaging and organ printing, UCLA researchers acknowledge the hurdles involved in implementing AI-driven systems in current medical imaging procedures.
AI tools can add biases to scans that may stem from different elements including unrepresentative training data and lack of diversity in the datasets used for training. These factors could skew the AI’s learning process, ultimately resulting in reduced diagnostic accuracy and suboptimal medical imaging care services.
To prevent this from happening, UCLA researchers aim to address potential bias concerns by continuing to refine SLIViT’s model features. Their goal is to advance the system for effective integration into contemporary medical imaging while ensuring patient result accuracy and contributing to reliable medical imaging services.