Stanford Medicine Develops AI Tool to Enhance ADHD Care
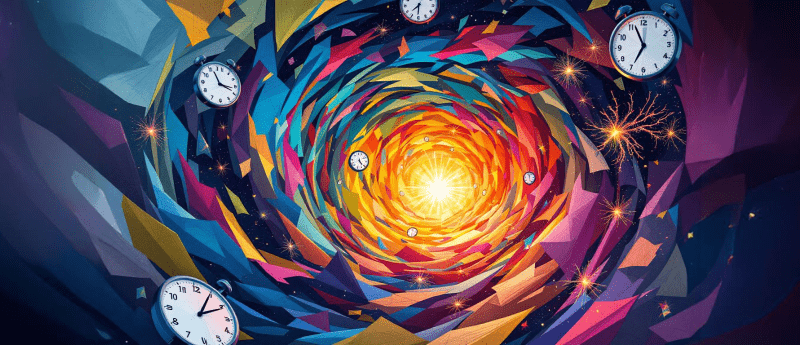
Stanford Medicine (CA, U.S.) researchers developed an AI-powered tool to scan clinical documentation efficiently while detecting patterns and gaps in medical care. This technology aims to enhance the monitoring and management of attention deficit hyperactivity disorder in children.
Described in a study published in the journal Pediatrics, the AI was designed to ascertain if children suffering from attention deficit hyperactivity disorder (ADHD) were provided with adequate monitoring and care after the prescription of new medications.
A Solution to Streamline ADHD Monitoring
ADHD is a prevalent condition among children, and its treatment often involves prescribing medications that require careful monitoring for side effects and effectiveness. However, to do this, clinicians face the daunting task of sifting through vast amounts of medical records, a process that can delay crucial decisions in patient care.
This is where the tool developed by Stanford Medicine proves its potential. The system seeks to free clinicians from time-intensive data retrieval, and identifies gaps in care, enabling targeted improvements in patient management.
How Was the AI Trained?
The researchers trained the AI using an already existing open-source large language model (LLaMA), fine-tuned to analyze clinical notes specific to ADHD-related clinical encounters.
The study reviewed medical records of 1,201 children aged 6 to 11, who were prescribed at least one ADHD medication. Specifically, they focused on a set of 501 notes documenting interactions between clinicians and patients with ADHD, to identify side effects of prescribed medications, such as reduced appetite.
To train the model, the researchers used 411 of the 501 notes to help the AI understand patterns in documenting medication side effects. The remaining 90 notes were set aside for testing, ensuring that the model could accurately identify side effects when applied to new, unseen data.
The results were impressive, with the AI model correctly classifying 90% of the notes, demonstrating its ability to analyze clinical data effectively.
Uncovering Care Gaps and Enhancing Outcomes
Once the system was optimized, the AI was deployed to analyze 15,628 patient chart notes. This task, which would have required over seven months of manual review, was completed by the AI in a fraction of the time.
The insights gained were profound. For example, the tool revealed discrepancies in how practices addressed concerns of side effects brought up during patient follow-ups.
Identifying these gaps is significant as it allows healthcare providers to improve their communication strategies and ensure that all patients are consistently monitored for potential medication side effects.
Thus, researchers concluded that deploying an AI model such as this “offered scalable measurement of quality of care and uncovered opportunities to improve psychopharmacological medication management in primary care.”
“That is something that you would never be able to detect if you didn´t deploy this model on 16,000 notes the way we did, because no human will sit and do that”.
Yair Bannett, MD, Assistant Professor of Pediatrics, Study Lead Author.
The Uncertain Future of AI in Healthcare
As Bannet highlights, AI is particularly effective for word-heavy tasks, such as sorting through medical records. It is a tool that should be leveraged to allow for more streamlined and hands-on patient care.
In the context of ADHD, the tool provides a scalable method to measure care quality and improve medication management in primary care settings.
Consequently, many large companies have already taken the initiative to develop AI tools to enhance clinical note-taking, and streamline administrative tasks, allowing doctors to redirect their time and focus on patient interaction.
However, some areas require careful evaluation to ensure AI integration enhances care without disrupting clinical workflows. AI models are trained on existing data, and extensive research has shown that disparities exist among individuals who participate in studies that are aimed at collecting medical data on various factors, including socioeconomic status, race, and geographic location.
Using this data to train AI would in turn cause inequitable predictions by the AI tools. If these biases are not addressed, AI tools may inadvertently perpetuate or even worsen existing inequities in patient care.
Therefore, as new AI tools are developed, efforts must be made to address and reduce these biases. Researchers and developers must focus on creating fair, inclusive AI systems that work for all populations.
Nevertheless, despite these concerns, with the proper safeguards in place, AI has the potential to enhance the work of doctors. This potential has already been demonstrated in various areas of healthcare, such as protein research, dialysis care, and pathology disease diagnosis.
“Each patient has their own experience, and the clinician has their knowledge base, but with AI, I can put at your fingertips the knowledge from large populations”.
Yair Bannett, MD, Assistant Professor of Pediatrics, Study Lead Author.