Stanford’s AI Tool Nucleio.io Accelerates Pathology Disease Diagnoses by 62%
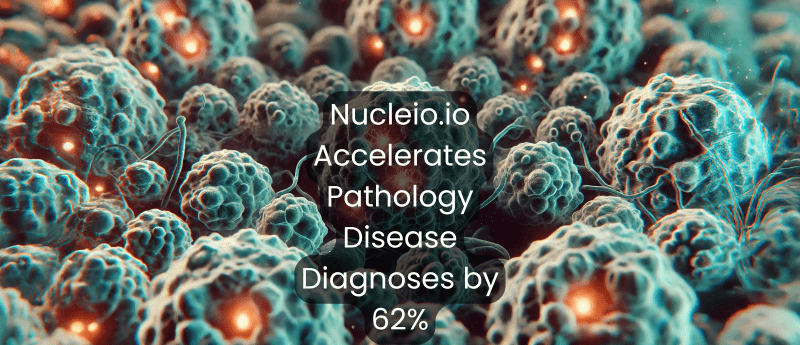
Researchers from Stanford University Medicine (CA, U.S.) have created an AI tool that can assist pathologists in detecting diseases with 72% more accuracy and 62% faster than those who did not use the software.
Nuclei.io, described in a Nature Biomedical Engineering paper, can detect diseased cells under a microscope, potentially assisting clinicians in recognizing those that could be signs of endometritis or metastatic colon cancer.
Pathologist Scarcity
Pathologists, who analyze tissue or fluid samples to diagnose diseases, frequently encounter complex challenges when differentiating between diseased and healthy cells. Mastering the ability to isolate diseased cells and make accurate diagnoses typically demands years of extensive training and can often be a tedious and time-extensive procedure.
This is probably one of the reasons why, according to Andrew Bychkov and Junya Fukuoka, there is a severe global scarcity of pathologists—four times fewer than required. Additionally, studies suggest that the “pathologist gap” may grow through 2030.
Thus, there is a need to fill in this pathologist gap and speed up time-consuming tasks in pathology to relieve the strain on medical services.
Overcoming Challenges in AI Development for Pathology
Developing an AI system that assists pathologists is challenging, hugely due to the existence of over 8,000 disease classifications, each with molecular and cellular foundations that continue to evolve with ongoing research. As a result, compiling a dataset that comprehensively represents all these diseases is extremely difficult.
Training an AI model for pathology with an incomplete dataset may lead to inconsistency and inaccuracy when the AI model is deployed in clinical settings.
Thus, researchers at Standford University aim to develop Nuclei.io, a system that leverages active learning and integrates real-time feedback from pathologists during training, enabling the system to adapt dynamically throughout the model-building process.
This approach ensures that the AI is finely tuned to meet specific diagnostic requirements, while actively incorporating data from a broader range of disease classifications. By enabling pathologists to provide real-time feedback during training, Nuclei.io dynamically expands its dataset, corrects errors, and adapts to pathologists’ workflows. This makes nuclei.io increasingly more comprehensive and adaptable to a wider set of disease classifications and other pathologists’ workflows.
Additionally, unlike other AI pathology models that primarily focus on tissue-level analysis, Nuclei.io emphasizes nuclear features, which enables detailed single-cell analysis for greater precision and interpretability. According to the Nature paper researchers, the goal of the device is “to ensure patients are receiving fast and accurate diagnoses.”
The Potential of Nuclei.io
According to researchers, Nuclei.io machine learning support decreased the average diagnosis time from 209 to 79 seconds, resulting in a 62% increase in efficiency.
“This is one of the first tools that enables personalized assistance, that adapts AI models through an active learning approach to personalize it to the needs of individual doctors”.
James Zou, PhD, Associate Professor of Biomedical Data Science and Co-Senior Author of the Paper.
During initial trials, nuclei.io expedited pathologists’ work, and researchers concluded that “doctors using nuclei.io to diagnose endometritis – an inflammation of the urine lining- or metastatic colon cancer were 62% faster at making diagnoses and 72% more accurate than they were without the program.”
Nuclei.io’s potential to enhance clinicians’ performance in disease diagnosis, by speeding up and facilitating the process while reducing the risk of human error, highlights the potential for a reliable and balanced collaboration between human and AI-powered healthcare, with the ultimate purpose of improving patient care.
“We don’t want a tool that replaces doctors, but something that collaborates well with doctors. We found that a pathologist assisted by the AI is much better than either the pathologist by themselves or the AI by itself.”
James Zou, PhD, Associate Professor of Biomedical Data Science and Co-Senior Author of the Paper.
Challenges Ahead
AI has recently emerged as a transformative force in healthcare, showing promise in areas such as early detection of breast cancer, predicting fetal health, and personalizing antibiotic treatments. Nuclei.io adds to this growing list, as its open-source nature allows the system to be adopted by other institutions beyond Stanford University, amplifying its potential implementation in broader healthcare contexts.
However, the integration of AI into medical practices is not without significant challenges. As a relatively new technology, AI faces a lack of standardized regulations around its ethical application, raising concerns about device reliability, inherent biases and the protection of patient privacy.
Even though AI has enormous potential in pathology and despite the researcher’s effort to maximise the amount of data nuclei.io is trained on, it might still be difficult to supply sufficient data for training. The sheer number of disease classifications, combined with continuous breakthroughs in research, necessitates regular updates to the AI’s dataset to keep it current and effective.
According to The Stanford Daily, Eric Yang, a pathologist involved in developing Nuclei.io, points out the challenge of collecting adequate training data. Currently, the widespread use and reliability of AI in pathology may not be fully applicable due to the limited amount of data available.
However, he explains that active learning helps streamline the training process. By enabling the model to focus on the most valuable data points, active learning not only shortens the time needed for training but also reduces the overall data volume required.
Therefore, with ongoing training, active learning, and collaboration from a broader range of pathologists, the AI system has the potential to improve significantly. As more diverse data is incorporated and the model becomes more refined through continuous feedback, it could eventually become a highly reliable and widely implemented tool in the field, offering greater accuracy and support for pathologists in their work.