Studies Reveal Gender and Ethnicity Biases in AI-Generated Images
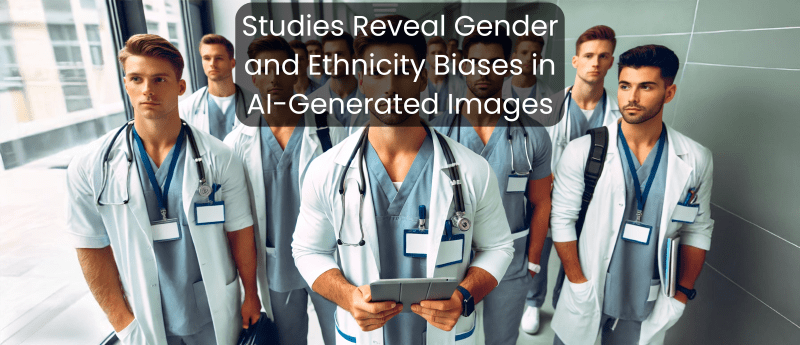
Two recent studies by researchers at Charles Sturt University (CSU; Australia) have found that Generative AI (GenAI) could reflect gender and ethnicity bias when generating visuals in healthcare.
The studies, published in the journals Sage and International Journal of Pharmacy Practice respectively, identified bias disadvantaging women and ethnic minorities in AI-driven image representations of undergraduate medicine students and Australian pharmacists.
Studies Methodology and Results
Both CSU studies examined the application of DALL-E 3, a text-to-image generative AI tool integrated within ChatGPT, to create various individual and group images of undergraduate medical students and pharmacists. The studies were both led by Prof Geoff Currie.
In the first study, 47 pictures were created with DALL-E 3 for examination. Of these, 33 featured solitary characters and the remaining 14 included multiple characters. Three reviewers individually assessed each photograph for apparent gender and skin tone. A total of 448 characters were assessed, including 33 individual figures and 417 in groupings.
The results showed that in both individual and group images, 58.8% of the photos DALL-E 3 generated featured male medical students, while only 39.9% were of women. Moreover, 92.0% of the individuals were light-skinned, and 0% of them had a dark skin tone. Among the photos of individual medical students, 92% of the pictures generated by DALL-E 3 were of men, and all of them had light skin tones.
Image: Study 1; Representative examples of DALL-E 3-generated images of typical undergraduate medical students studying in Australia (top left and right, and bottom middle and right), and a typical undergraduate medical student studying in Australia and receiving an award or scholarship (bottom left).
Similarly, the second study, conducted by CSU’s School of Dentistry and Medical Sciences, also employed DALL-E 3 to create individual and group images of Australian pharmacists specifically.
A total of 40 images were generated for evaluation, comprising 30 individual characters and 10 images featuring multiple characters. Two independent reviewers analyzed all images for characteristics such as gender, age, ethnicity, skin tone, and body habitus.
Collectively, 69% of the individual and group pictures created by DALL-E 3 featured men pharmacists, while only 29.7% featured females. Additionally, 93.5% of them were light skinned. Among the images of individual pharmacists, DALL-E 3 generated only males, and all were light-skinned.
Picture: Study 2; Representative examples of DALL-E 3 generated images of typical pharmacists in Australia. Top left is an Australian pharmacist and top right is an Australian community pharmacist. Middle left is an Australian hospital pharmacist and middle right is an Australian research pharmacist. Bottom left is an Australian pharmacy student and bottom right is an Australian pharmacist academic.
Australia: Where Female Clinicians Lead Medicine
According to CSU researchers, 54.3% of medical students in Australia are women, and 64% of pharmacists in the country are female.
However, the CSU studies highlighted that artificially generated images failed to reflect these numbers.
This underscores the biases inherent in AI-driven tools, which may exacerbate gender and ethnicity disparities by significantly depicting medical students and pharmacists in Australia as white males. This is an inaccurate portrayal of the current diversity within the profession in the country.
“If images carrying such bias are circulated, it erodes the hard work done over decades to create diversity in medical and pharmacy workforces and risks deterring minority groups and women from pursuing a career in these fields.”
Geoff Currie, Professor in Nuclear Medicine at CSU’s School of Dentistry and Medical Sciences
The Limitations of AI
AI’s transformative potential has become increasingly evident through its recent contributions to various areas of healthcare, including pulmonary embolism diagnosis, protein stability prediction and research on tuberculosis and malaria.
However, as the CSU studies have evidenced, AI technologies are also prone to error, bias, and prejudice.
According to Hewis, “Society has been quick to adopt AI because it can create bespoke images whilst negating challenges like copyright and confidentiality”.
“However, accuracy of representation cannot be presumed, especially when creating images for professional or clinical use”.
The fast deployment of AI for medical image production must not overwhelm the critical requirement for accuracy and fairness. Therefore, further efforts must focus on addressing AI-generated biases to leverage AI’s transformational promise in healthcare while ensuring integrity and inclusion.