Take a chance on ML: predicting the risk of cardiovascular disease with AI
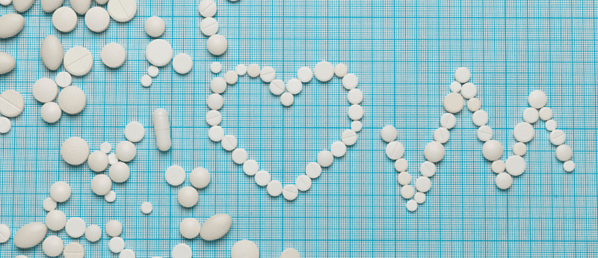
Machine learning can now predict which patients are at risk of cardiovascular disease by identifying genetic risk variants, according to a study recently published in Genomics.
A novel machine learning (ML) tool may aid physicians in the early diagnosis of patients at risk of cardiovascular disease, including heart failure and arterial fibrillation. A research team from Rutgers Institute for Health, Health Care Policy and Aging Research (NJ, USA) have utilized AI to predict highly significant cardiovascular genes that are linked to demographic variables such as age, gender and race.
Genes are mysterious entities. Filled with hidden secrets and uncharted territory, an abyss of infinite possibilities. Some of these possibilities are already defined. But most are gambles, risks that intertwine to form an outcome in our lives. Like gambling, some things are impossible to control, but sometimes, we can succeed in life’s game and predict possible outcomes based on chance.
Genomics is a rapidly evolving field of science; however we have barely scratched the surface. Huge progress has been made in determining some genetic outcomes, such as causal variants and genes that lead to diseases such as sickle cell anemia, cystic fibrosis and Huntington’s Disease. We also understand a lot about genetic risk variants, but their complexity and integration within certain multifactorial diseases is complex and it is challenging to pick this information apart in the abundance of heterogeneous genetic data. Yet, we may now have a solution to identifying disease-associated genes and variants in the form of AI.
The Centers for Disease Control and Prevention reports cardiovascular disease as the main cause of death worldwide. Of the 17.9 million deaths per year, approximately 45% of cardiovascular disease deaths are due to atrial fibrillation and heart failure and the majority of deaths can be prevented by managing risk factors.
Cardiovascular diseases are a group of several complex conditions affecting the heart and blood vessels, ranging from coronary heart disease to strokes. Cardiovascular disease is clinically difficult to diagnose, meaning management and treatment of the disease is also hindered. Risk assessments are particularly difficult as cardiovascular disease is dependent on a wide range of factors, including genetic and environmental factors and lifestyle choices. However, early identification of cardiovascular disease-related genes can lead to advancements in treatment and diagnosis.
In this study, the team utilized a ML model to examine and identify genes in patients diagnosed with cardiovascular disease and healthy controls that were associated with the most prevalent cardiovascular diseases, including heart failure and atrial fibrillation.
The model identified a total of seven genes that were significantly associated with heart failure and other cardiovascular diseases, all of which were downregulated in patients with cardiovascular disease compared to healthy controls. These genes were also significantly associated with demographic variables, including race, age and gender.
Age and race factors were associated with atrial fibrillation, while age and gender were correlated to heart failure. One of the most significant positive correlations was patient age, as cardiovascular disease likelihood increased with age.
“Timely understanding and precise treatment of cardiovascular disease will ultimately benefit millions of individuals by reducing the high risk for mortality and improving the quality of life.” declared Zeeshan Ahmed, an Assistant Professor from the Department of Medicine at Rutgers Robert Wood Johnson Medical School (NJ, USA).
Further research is necessary for additional clinical application of this ML approach to a larger dataset. Future studies could analyze the entire range of genes in cardiovascular disease patients, potentially discovering novel risk genes and biomarkers that can influence susceptibility to the disease. The application of AI in such diagnostic methods may accelerate our ability to identify genes that have significant effects for many diseases.