The Journey of Medical LMMs and Where we are Now

The medical field is evolving rapidly, now racing toward an AI-driven future powered by massive amounts of data. As words like “efficiency” and “speed” ricochet across the industry, it’s important to tune into the resounding melody at the heart of this effort: better patient care.
Ever since the craze around Open AI’s Large Language Model (LLM) “ChatGPT” in November 2022, we have been left to wonder where AI’s capabilities might take us—not just for simple, administrative tasks but for highly complex ones that require deep reasoning and cross-contextual understanding.
While general-purpose LLMs captured the public’s imagination, a wave of domain-specific innovation showcased what AI can really do. In 2022, LLM-based platform Harvey partnered with OpenAI to help lawyers cut down the time spent on legal research and contract reviews. Which it did—and by a lot. Now integrated across 250 leading law firms and enterprises from 42 countries, the AI slashes the time taken on legal tasks by up to 50%.
Laying the Groundwork for AI in Health
Through a medical lens, the LLM timeline begins in earnest with the 2022 release of Med-PaLM—a generalist model from Google Research and DeepMind specifically fine-tuned to answer multiple-choice medical exam questions. Although MedPaLM was the first AI system to surpass the pass mark (60%) in US Medical Licensing Examination-style questions, the real buzz came from its successor, Med-PaLM-2, in 2023, after the AI reached “human expert level” when tackling the same exam.
But AI, particularly in healthcare, was still in its infancy. Media headlines lit up with claims that MedPaLM-2 could “rival your GP”—an enthusiasm not reflected by the scientific community. Experts remained skeptical, pointing to risks of bias, hallucinations, and misdiagnosis, with the World Health Organization issuing a “stark warning” that echoed these concerns.
Regardless, Med-PaLM —and Med-PaLM-2— signaled a milestone for AI in healthcare, though not the first development in the space.
Systems like Google’s BioBERT —a classification model for biomedical text— and its clinical counterpart, ClinicalBERT —for understanding doctors’ notes or discharge summaries— had already been in circulation since 2019. In 2022, Microsoft Research released its BioGPT—a model that could generate and summarize biomedical text. Then, in 2022, but still pre-dating Med-PaLM, NVIDIA collaborated with the University of Florida to develop GatorTron—a large clinical language model that built on the momentum of earlier models like ClinicalBERT, but trained on a much larger corpus of electronic health records.
But these models were smaller and built with specific tasks in mind, trained exclusively on biomedical data—something that restricted both their capabilities and accuracy. Med-PaLM, however, marked a leap toward bigger, general-purpose AI in healthcare by “opening up the training grounds”: first, exposing the model to massive, diverse datasets, unshackling it from the restraints of biological data alone, and then tailoring it with specialized medical data. This progression owes much to the rise of more powerful models like GPT-4, which paved the way for larger, more adaptable systems that could excel in a broader range of applications—including healthcare.
AMIE: The AI Model That Talks Like a Doctor
Tracing the LLM evolutionary tree, we reach a key branching point with Google’s 2024 release of Articulate Medical Intelligence Explorer (AMIE). Moving away from the static, Q&A-like platform of Med-PaLM, AMIE is geared towards a more dynamic experience, capable of “talking” directly to patients and pinpointing a diagnosis—just as accurately as human doctors, but with better bedside manner.
In the seminal paper “Towards conversational diagnostic artificial intelligence,” published in Nature earlier this month, the authors place physician-patient interactions at the heart of effective care—in some cases, 60–80% of diagnoses can be made just from a patient’s clinical history.
Addressing the Physician Burnout Crisis
But not all of these conversations end in clarity. Things like personality differences, tight and busy schedules, or dealing with abrupt patients can make consultations disjointed and jagged—as a result, key details might slip through the cracks.
Meanwhile, doctors are burnt out, and the workforce is stretched thin—two sides of the same “deteriorating healthcare” coin that rolls towards missed diagnoses and worse patient outcomes. In 2023, the Great British Medical Council reported that clinicians’ stress had reached an all-time high, with 44% of doctors struggling to provide sufficient patient care on at least one occasion per week. A year later, in 2024, the NHS’s Ombudsman warned that cancer patients could be at risk because of “overstretched and exhausted health staff.”
He commented: “Patient safety will always be at risk in environments that are understaffed and where staff are exhausted and under unsustainable pressure. We need to see concerted and sustained action from the Government to make sure NHS leaders can prioritize the safety of patients and are accountable for doing so.”
And it’s not just the UK. Physician burnout has been termed a “global crisis” for over a decade; in 2019, more than one in three (37%) doctors reported feeling burnt out, and 10% said they were experiencing both burnout and depression. Hand-in-hand with this, physicians are feeling undervalued and underpaid, seeking refuge in alternative careers or other countries with better benefits. In the UK, about a third of all doctors consider leaving—from a global perspective, at least 50% of older physicians said they would stop seeing patients in the next three years.
These statistics paint a vivid picture of “rats abandoning a sinking ship”—not from lack of care, but pure exhaustion. Unlike this image, though, as more doctors flee the medical scene, the healthcare “ship” only gets weaker.
AI with a Stethascope: Is This the Future of Your GP Appointment?
This is where tools like AMIE come in. While doctors may falter during the medical consultation due to stress or patient hostility, LLMs offer a more resilient tactic, immune to these external pressures.
But perhaps more important than consistency is empathy—often described as the “cornerstone of effective doctor-patient communication.” And, despite long-standing concerns surrounding AI’s capacity for human emotion, recent studies show that LLMs emulate empathy well; one study, comparing ChatGPT’s responses to those of physicians, found that patients preferred the AI’s responses in more than 78% of cases.
It’s this potential that AMIE’s developers are tapping into, noting that AI systems capable of diagnostic dialogue could increase “accessibility, consistency, and quality of care.” Similar to previous AI models, AMIE could diagnose patients as well as —or even more accurately than— physicians. But unlike earlier systems, it didn’t rely on pre-collected data like MRI scans or blood test results. Instead, AMIE had to actively ask questions to piece together a diagnosis.
To build it, the Google team started with their generalist large language model PaLM-2—the same one used to build Med-PaLM-2. But to fine-tune the model, they used a novel approach. AMIE was “taught” using a “self-play” mechanism, where the AI creates simulated dialogues, critiques itself, and refines its responses—all on its own.
AMIE then interviewed 20 actors pretending to be patients and diagnosed them, where it managed to surpass or match medical professionals’ accuracy across 159 scenarios. The “patients” also evaluated the system’s bedside manner, which was deemed more favorable than the physicians’.
Although Google stressed that the research was purely “experimental,” the results did invite the intriguing —if somewhat dubious— question, “Will we ever get to a stage where AI is running our GP appointments?” Perhaps, a more realistic version of this scenario might involve an initial AI-led screening process, carefully monitored by human clinicians.
AMIE Outperforms Clinicians at Diagnostic Reasoning
Paralleling this research, Nature published another AMIE paper at the same time: “Towards accurate differential diagnosis with large language models.” While the other paper explored AMIE’s potential to simulate patient-doctor conversations, this one asked whether AMIE could use nuanced clinical reasoning to diagnose a patient—essentially, emulating the role of a specialist, rather than a GP.
To fit the task, the researchers started with the “malleable” PaLM-2 model—just like they had for the conversational study. To fine-tune it, though, required more data: electronic health record summaries, over 11,000 medical exam questions, and records of 218 patient-doctor conversations.
Clinicians tested AMIE on over 300 medical transcripts, prompting the AI to generate a list of possible diagnoses from real patient cases. Once an initial list was made, they could ask follow-up questions to help reach a final decision. Each differential diagnosis was then evaluated on (1) quality—“Does the list include the correct diagnosis?”, (2) appropriateness—“How suitable is the list of diagnoses?”, and (3) comprehensiveness— “Are all the potential diagnoses covered?”
Across all three, AMIE improved clinicians’ scores compared to the differentials they made alone. AMIE managed to get the correct diagnosis in its list of top-10 possibilities nearly 60% of the time. Without assistance, doctors’ top-10 accuracy almost halved, naming the right diagnosis only 34% of the time. Even when these doctors had access to references and internet search, AMIE still outperformed them (see below).
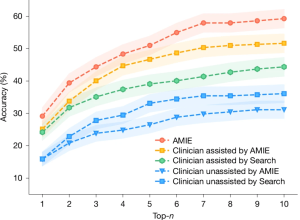
Percentage accuracy of differential diagnoses lists, evaluated by humans. Taken from the original paper.
While the authors urge caution in using these results “towards any implications about the utility of AMIE as a standalone diagnostic tool,” we can consider this a leap towards powerful assistive technology that could help clinicians confidently make smarter decisions. At a time when the workforce is dwindling, and exhausted health workers are more susceptible to mistakes, it begs the question: “Can we afford not to invest in tools like AMIE?”
Stretching AMIE’s Capabilities For Long-Term Patient Care
Now, Google is taking strides with AMIE towards long-term disease management—going far beyond the initial diagnostic stage and exploring how AI fares with a longer clinical timeframe.
In a paper recently submitted to arxiv, “Towards Conversational AI for Disease Management,” AMIE combines a dialogue agent, just like the one discussed in the first paper, with a management agent. Here, the dialogue agent “texts” patients over the course of multiple visits to retrieve relevant medical information, which the management agent considers within the broader context, i.e., current clinical guidelines, to piece together a tailored management strategy.
Essentially, this tested AMIE’s ability to:
- Make an accurate diagnosis using both the initial consultation and follow-up test results.
- Recommend a clinically safe and effective treatment tailored to the patient—such as prescribing an appropriate blood pressure medication.
- Review the patient’s progress over time and adjust the plan if needed—for instance, switching to an alternative medication in response to side effects, just as a doctor might.
This time, instead of starting with PaLM-2, the researchers built AMIE using a Gemini 1.5 Flash base—a choice driven by Gemini’s ability to understand and remember a lot of information all at once.
Interwoven with electronic health records and medical exam questions, they fine-tuned AMIE on real-world patient-doctor dialogues and simulated scenarios, following the patient over multiple visits rather than just one consultation.
AMIE frames these conversations within almost 630 clinical guidelines, generating multiple management plans in just one minute before refining them into a comprehensive plan.
AMIE: AI Beats Physicians at Disease Management for the First Time
Tested with 21 patient actors and 21 board-certified practitioners, AMIE was evaluated along two key dimensions, reflecting its dual-agent system: (1) Its ability to communicate—judged by patient actors on how well it managed medical worries and built a sense of comfort; (2) the quality of its care—evaluated by medical professionals for clinical soundness and adherence to medical guidelines. These core dimensions were further broken down into 25 axes: 10 measured its ability to communicate like a doctor, and the remaining 15 captured the quality of its clinical decisions.
And the results were promising. Across all 15 management axes and three recurrent patient visits, AMIE performed at least as well as real human doctors. But in terms of precision, i.e., asking if it gave sufficient detail for recommended treatments or follow-up investigations, it scored significantly higher—and consistently. At the end of the first visit, AMIE achieved a 94% rating for its precision in treatment prescribing, compared to the human doctors’ score of 67%. By the end of the third and final visit, AMIE was rated 91%, while human doctors’ scores had only increased to 70%.
Not only was it more precise, but AMIE followed clinical standards more closely. For all three visits, AMIE received significantly higher scores for recommending treatments that were aligned with the guidelines compared to human doctors (89% vs 75%).
That being said, this might not always be ideal. For example, AI systems may be reluctant to suggest new therapies that haven’t yet been incorporated into formal guidelines, even if they could offer very real benefits to certain patients—something a human doctor might be better positioned to justify when the circumstances call for it.
Regardless, scientists are calling the study “revolutionary” and “truly groundbreaking.” As the first time that AI has matched or even outperformed physicians at disease management, AMIE represents a milestone in the future of AI and healthcare. Now, we can see that agentic LLMs have the potential to safely and effectively navigate patients’ health over a long period—or, at least, across three visits.
Zooming Out From Google’s AMIE
AMIE aside, researchers are continually exploring how LLMs might intersect with the different aspects of healthcare.
From an academic standpoint, Open AI’s Chat-GPT is considered a major “disruptor” in the space—being the model of choice for nearly 80% of all healthcare-LLM research. With some sources estimating GPT-4 to have over 1.7 trillion parameters, this selection is often because of its immense reasoning capabilities—having been exposed to a diverse dataset, LLMs like ChatGPT demonstrate a remarkable ability to perform complex tasks with nuanced contextual cues.
Think of it like a consultant. A smaller model trained solely on health data is like hiring a specialist who’s only ever worked in one hospital—they’re sharp in their field but limited to that environment. In contrast, GPT-4 is like bringing in a polymath who’s worked across industries, countries, and cultures. Its vast and diverse experience lets it draw connections across domains, navigate nuanced situations, and offer solutions that go beyond textbook answers.
But there’s a caveat. Adapting ChatGPT for tasks such as comprehensive disease management raises concerns about transparency, or the lack thereof—more commonly known as the “black box” conundrum. According to a Nature review from January 2025, a majority of studies report model opacity as a “significant barrier to their application in healthcare,” leaning into the critical question: if healthcare professionals can’t understand how a model has arrived at its answer, what happens when AI makes a mistake?
Google’s AMIE directly addresses this with its “structured generation” framework, which breaks down the reason behind each of its decisions—bulstering both traceability and interpretability of the model. That way, if a clinician questions one of AMIE’s recommendations—such as prescribing a particular medication—they can easily review the model’s rationale and make an informed choice to accept or challenge it.
Limitations to Consider
Still, there are some restrictions to this research.
Firstly, the study is exclusively based on simulations, which may lack some of the complexities we would usually expect in real life—for example, these “made-up” scenarios have a clear-cut solution, but this is not necessarily the case in clinical practice.
In a similar vein, simulations require actors who are psychologically removed from the situation. If, in a study, they are told they have a serious medical condition, they may act distressed, but they will not experience it the way a real patient would—the emotional dynamics of such a consultation are almost impossible to replicate. Whether an AI could effectively navigate these emotionally-charged encounters and provide the same level of comfort as a human doctor is questionable.
Secondly, while the study encouraged the human doctors to follow clinical guidelines as closely as possible, practitioners are not ordinarily constrained by a strict set of rules—instead, they often draw on personal experiences or point-of-care reference tools to supplement their decisions. Because of this, human doctors are more likely to suggest an innovative treatment if they believe their patient will gain real benefit from it, even if this doesn’t rigidly align with the guidelines.
The time elapsing between each visit was also not realistic—usually, a patient with a long-term health concern will revisit their doctor over a period of several weeks or months. But, in this study, these visits took place only 1–2 days apart. It would be more meaningful to assess how AI actually manages health conditions over a long period, rather than estimating how well it could perform based on a compressed timeline.
Concluding remarks
But, this being said, AMIE represents an exciting milestone for AI’s unravelling role in healthcare. It does something we have not seen before—integrating three complex but essential aspects of healthcare into one agentic system:
- Interacting directly with patients to obtain relevant information.
- Reasoning step-by-step through medical scenarios.
- Constructing complete patient management plans grounded in clinical guidelines and making any necessary adjustments over an extended period.
Although far off from clinical deployment, the evolution of AMIE exemplifies LLMs’ broad potential applications, from emulating patient-doctor conversations, to performing accurate differential diagnoses, to managing long-term health in-keeping with clinical guidelines. While most AI systems aim to augment clinicians, Google’s AMIE pushes the boundaries and asks: “Could AI actually replace doctors one day?”