Transforming Protein Design | Creating Soluble Membrane Protein Analogues with Deep Learning
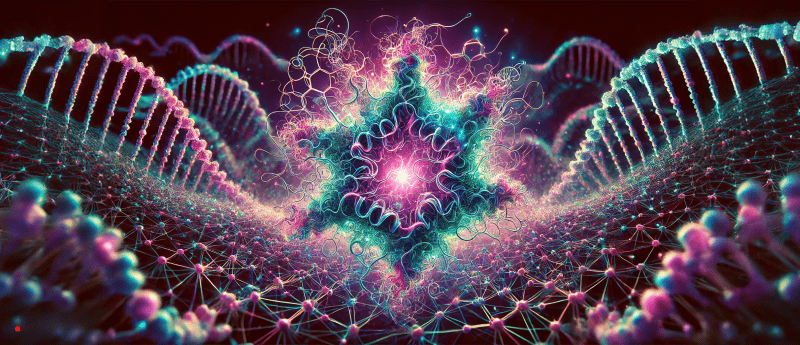
Designing complex protein structures from scratch has always been a significant challenge in biology. However, recent advances in deep learning have provided a promising solution. Researchers have successfully used deep learning to design complex protein structures and soluble versions of membrane proteins. This breakthrough could revolutionize drug discovery and expand our understanding of protein functions.
The Challenge of Protein Design
Traditionally, protein design has relied on methods like Rosetta, which require detailed restraints and extensive experimental testing. These methods struggle with designing proteins that have complex shapes.
Deep learning tools like AlphaFold2 (AF2) have greatly improved our ability to predict protein structures, but creating stable, functional proteins, especially those with intricate shapes, remains difficult.
Why Soluble Membrane Proteins Matter
Many drug discovery efforts focus on membrane proteins, which are proteins embedded in the cell’s outer layer. These proteins are crucial because they interact with drug molecules, triggering changes in the cell.
However, because they are part of the cell membrane and are hydrophobic (water-repellent), they are hard to study and produce in large quantities.
Researchers at the Laboratory of Protein Design and Immunoengineering (LPDI) aimed to solve this problem by redesigning membrane proteins to be soluble and stable. Casper Goverde, a PhD student at LPDI explained the teams’ rationale:
“We wanted to get these proteins out of the cell membrane, so we redesigned them as hyperstable, soluble analogues, which look like membrane proteins but are much easier to work with,”
This approach allows these proteins to be produced in large quantities using bacteria, making the process more efficient and cost-effective.
The Deep Learning Pipeline
The researchers developed a new computational pipeline that combines AF2 with a sequence optimization tool called ProteinMPNN. This pipeline can design stable protein structures, including soluble versions of membrane proteins like claudins, rhomboid proteases, and G-protein-coupled receptors (GPCRs). This method avoids the need for complex design restraints and extensive experimental testing.
Key Findings
- Complex Protein Design: The pipeline successfully designed complex protein structures such as Ig-like folds, β-barrels, and TIM-barrels. These proteins showed high stability and accuracy, demonstrating the potential of deep learning in protein design.
- Soluble Membrane Proteins: Researchers created soluble versions of membrane proteins, achieving high stability and solubility. For example, they designed soluble claudin analogues that maintained the functional properties of the original membrane proteins, making them useful for drug discovery.
- Functionality: The soluble analogues retained key functional features from the membrane proteins. For instance, soluble GPCR analogues were designed to keep their G-protein-binding sites. This shows that the deep learning pipeline can create functional proteins with complex features.
Making Protein Design More Accessible
Traditionally, designing proteins involved predicting new structures based on amino acid sequences. The LPDI team took a different approach by starting with the structure of membrane proteins and generating the corresponding amino acid sequences for soluble versions. Using AF2 for initial sequence generation and ProteinMPNN for optimization, they achieved impressive results.
Implications for Drug Discovery
Creating soluble versions of membrane proteins with functional properties opens new possibilities for drug discovery. Membrane proteins are key drug targets, and making them soluble makes it easier to study and develop new drugs. This method could lead to more efficient and innovative drug discovery processes.
LPDI scientist Martin Pacesa highlighting the significant impact of their work:
“We showed for the first time that we can redesign the GPCR shape as a stable soluble analogue. This has been a long-standing problem in biochemistry, because if you can make it soluble, you can screen for novel drugs much faster and more easily,”
Published in the journal Nature, the study also shows potential for applications in vaccine research and cancer treatment, demonstrating the wide-reaching benefits of this innovative protein design method.