Two drugs after our own hearts: preventing cardiac fibrosis
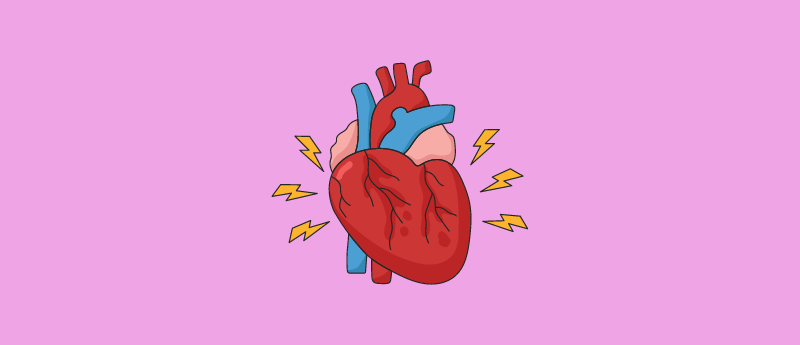
Scientists at the University of Virginia (UVA) (VA, USA) have harnessed the power of machine learning to identify a potential breakthrough drug that could mitigate harmful scarring following heart attacks.
Traditional approaches to drug discovery have struggled with the complexity of diseases like heart disease, cancer, and metabolic disorders. However, the UVA researchers have pioneered a novel method, combining decades of human knowledge with machine learning to understand how fibroblasts are affected by drugs.
Fibroblasts are cells that help maintain the structural framework of tissues, and they are crucial for heart repair after an injury. While fibroblasts aid in wound healing, they can also contribute to scarring, known as fibrosis. The challenge has been to pinpoint drugs that can target fibroblasts effectively, and thus prevent scarring.
To predict which drugs might be effective, but also understand how exactly they influence fibroblast behavior, the UVA researchers introduced “logic-based mechanistic machine learning.”
Their experiment involved analyzing the impact of 13 promising drugs on human fibroblasts, and using this analysis data to train the machine learning model to predict the drugs’ effects and behaviors.
Interestingly, the model successfully provided novel insights into how pirfenidone, an FDA-approved medication for idiopathic pulmonary fibrosis, inhibits the stiffening of the heart by reducing the formation of contractile fibers within fibroblasts. It also predicted how an experimental Src inhibitor, WH4023, could target a different type of contractile fiber, a finding that was experimentally validated in human cardiac fibroblasts.
While the drugs’ effectiveness needs further validation in animal models and human patients, the research showcases the potential of mechanistic machine learning to uncover biological cause-and-effect relationships.
This is just one study among many that demonstrates the collaborative power of machine learning and human understanding in advancing drug discovery, revealing an encouraging potential therapy for cardiac fibrosis, as well as other diseases.